Residual Attention Module on EEGN et for Brain-Computer Interface.
2023 IEEE Symposium Series on Computational Intelligence (SSCI)(2023)
摘要
Brain-computer interfaces (BCI) allow for the brain to communicate with electronic devices. Concerning the BCI paradigms, motor imagery uses brain signals to decode an imagined movement. However, this is a hard task given the low signal-to-noise ratio. Usually, the main steps in BCI models are pre-processing, feature extraction, and classification. In recent years, Convolutional Neural Networks (CNNs) have been gaining relevance in several areas of science due to their feature extraction, translation invariance, and parameter sharing capa-bilities. Another, more recent way of feature extraction is using attention mechanisms, which are layers of neural networks based on human attention and have the ability to highlight important features. A variation of the attention mechanism is the Convolutional Block Attention Module, which combines the CNN structure with the attention mechanism. In this work, we propose a new model that joins the core architecture of EEGNet, a compact CNN widely used in the literature, with the Convolutional Block Attention Module and residual connections. The residual connections were introduced to lower data degradation throughout the model. The results highlight the residual connection's importance for the performance of the model. The proposed model obtained a kappa result 5.2% better than the EEGNet with a p-value less than 0.01 on BCI Competition IV dataset 2a, which is a well-known dataset for Motor Imagery. Furthermore, the proposal was better than EEGNet for most subjects and had the best-worst case.
更多查看译文
关键词
Brain-Computer Interface,Motor Imagery,Convolutional Neural-Network,Attention Mechanism,Electroencephalogram
AI 理解论文
溯源树
样例
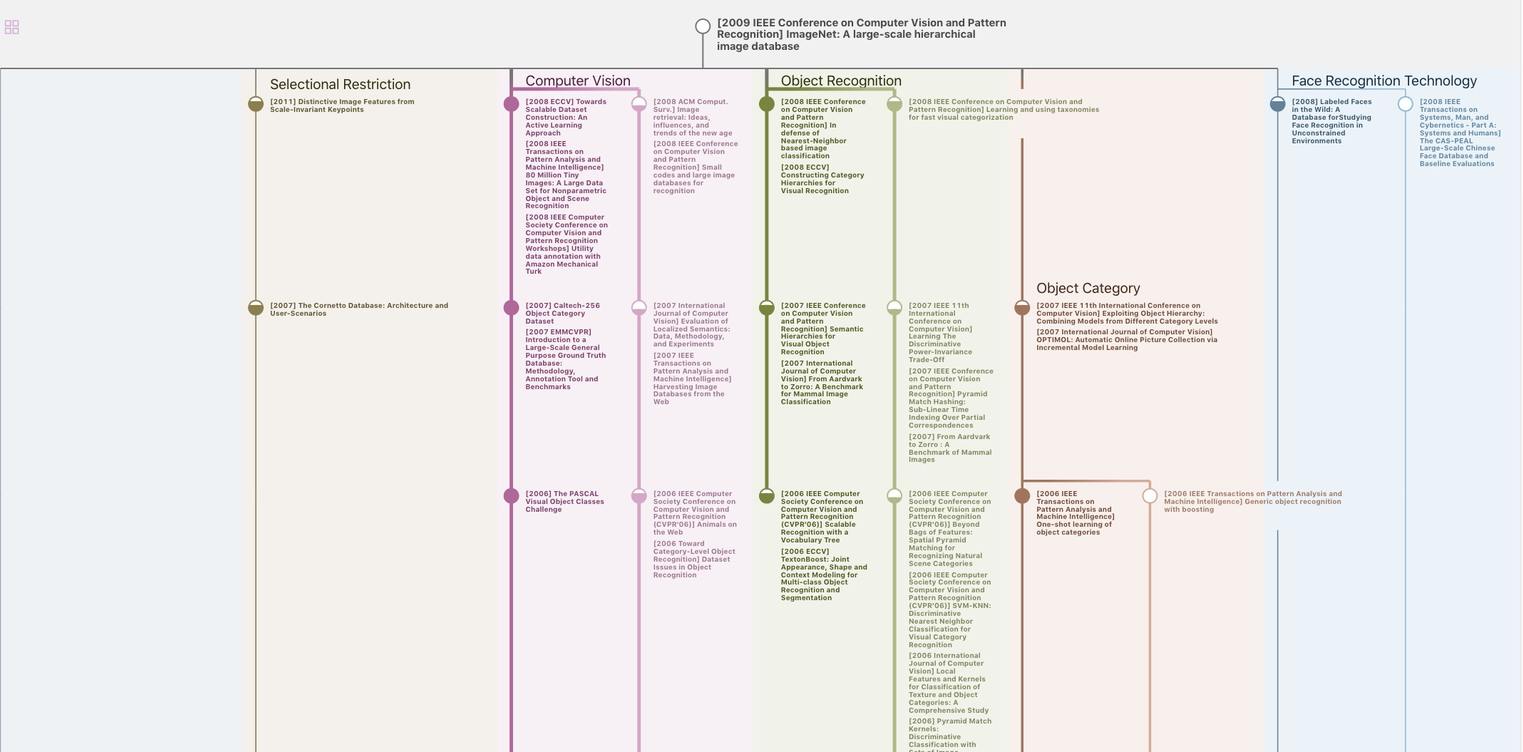
生成溯源树,研究论文发展脉络
Chat Paper
正在生成论文摘要