Drone-to-drone interception path planning by Deep Q-network with Graph Neural Network based (DQN-GNN) model.
2023 IEEE International Conference on Cybernetics and Intelligent Systems (CIS) and IEEE Conference on Robotics, Automation and Mechatronics (RAM)(2023)
摘要
With the increasing ubiquity of drones, criminals may also start using drones more frequently. This may necessitate catching these hostile drones with friendly drones. This paper proposes using reinforcement learning to train an agent involving a deep Q-network (DQN) with an underlying GNN-based model to move and intercept a moving target drone. The results from this approach seem to suggest that the use of GNNs with deep reinforcement learning is a feasible way to tackle this problem. With the method proposed in this work, the chaser drone can be trained to intercept the target drone. Furthermore, the chaser drone can catch the target in a reasonable amount of time.
更多查看译文
关键词
target tracking and following,target and chaser drones,air-to-air interception,reinforcement learning,deep Q-network (DQN),graph neural network (GNN)
AI 理解论文
溯源树
样例
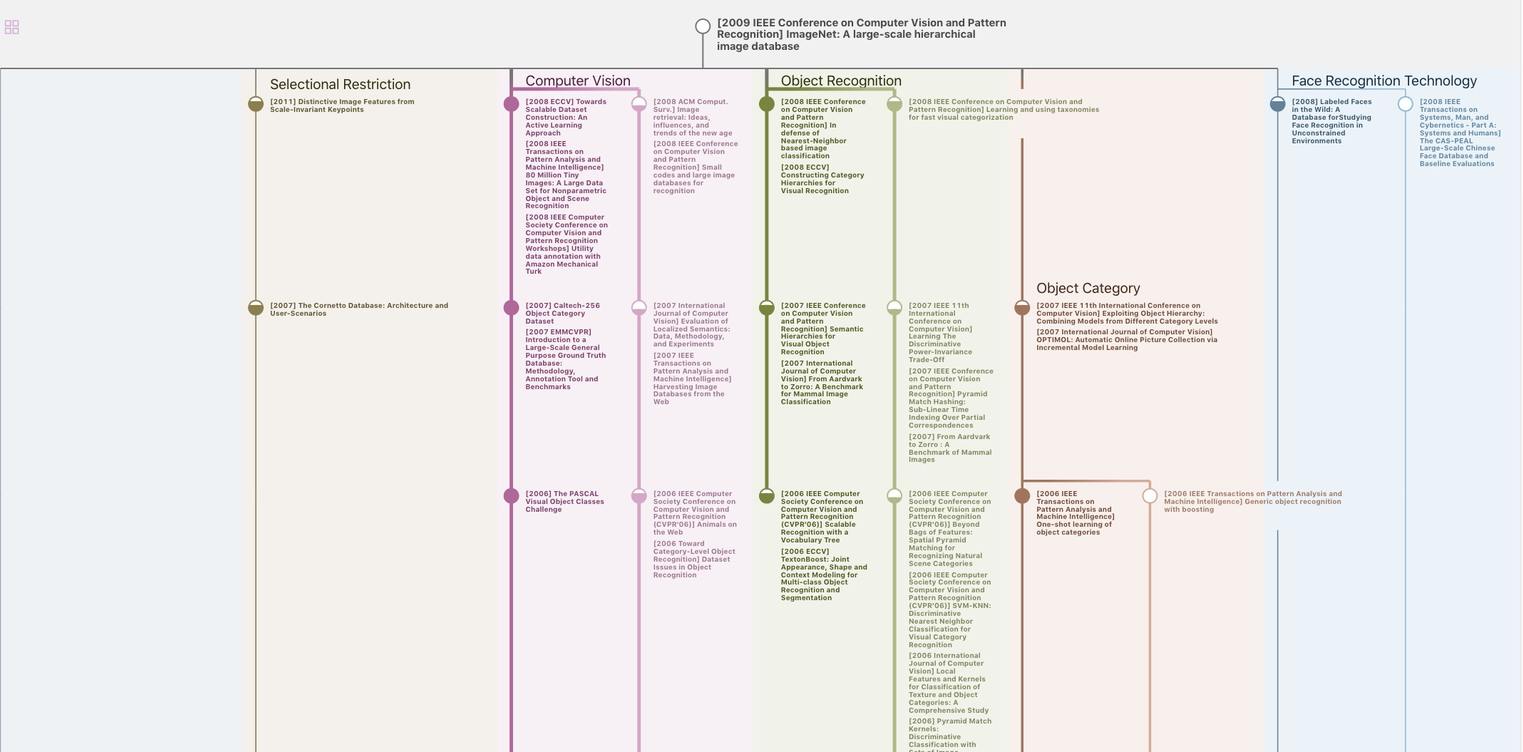
生成溯源树,研究论文发展脉络
Chat Paper
正在生成论文摘要