TbDd: A New trust-based, DRL-driven framework for blockchain sharding in IoT
Computer Networks(2024)
摘要
Integrating sharded blockchain with IoT presents a solution for trust issues and optimized data flow. Sharding boosts blockchain scalability by dividing its nodes into parallel shards, yet it is vulnerable to the 1% attacks where dishonest nodes target a shard to corrupt the entire blockchain. Balancing security with scalability is pivotal for such systems. Deep Reinforcement Learning (DRL) adeptly handles dynamic, complex systems and multi-dimensional optimization. This paper introduces a Trust-based and DRL-driven (TbDd) framework, crafted to counter collusion attack risks and dynamically adjust node allocation, enhancing throughput while maintaining network security. With a comprehensive trust evaluation mechanism, TbDd discerns node types and performs targeted resharding against potential threats. The TbDd framework maximizes the tolerance for dishonest nodes, optimizes node movement frequency, ensures even node distribution in shards, and balances sharding risks. Extensive evaluations validate TbDd’s superiority over conventional random-, community-, and trust-based sharding methods in shard risk equilibrium and reducing cross-shard transactions.
更多查看译文
关键词
Blockchain,Sharding,Collusion attacks,Trustworthiness,Deep reinforcement learning,Internet-of-Things
AI 理解论文
溯源树
样例
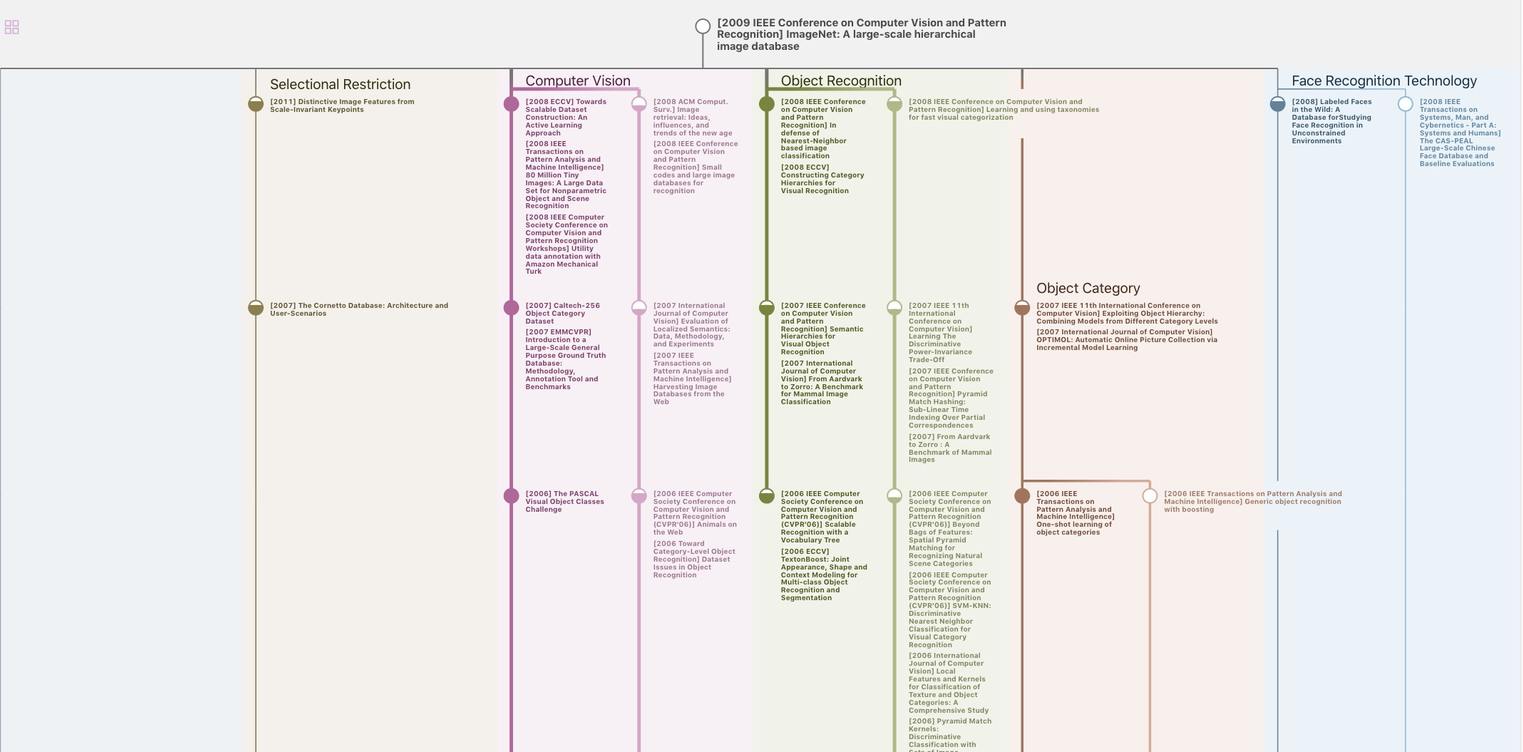
生成溯源树,研究论文发展脉络
Chat Paper
正在生成论文摘要