SHARE: Single-view Human Adversarial REconstruction
CoRR(2023)
摘要
The accuracy of 3D Human Pose and Shape reconstruction (HPS) from an image is
progressively improving. Yet, no known method is robust across all image
distortion. To address issues due to variations of camera poses, we introduce
SHARE, a novel fine-tuning method that utilizes adversarial data augmentation
to enhance the robustness of existing HPS techniques. We perform a
comprehensive analysis on the impact of camera poses on HPS reconstruction
outcomes. We first generated large-scale image datasets captured systematically
from diverse camera perspectives. We then established a mapping between camera
poses and reconstruction errors as a continuous function that characterizes the
relationship between camera poses and HPS quality. Leveraging this
representation, we introduce RoME (Regions of Maximal Error), a novel sampling
technique for our adversarial fine-tuning method.
The SHARE framework is generalizable across various single-view HPS methods
and we demonstrate its performance on HMR, SPIN, PARE, CLIFF and ExPose. Our
results illustrate a reduction in mean joint errors across single-view HPS
techniques, for images captured from multiple camera positions without
compromising their baseline performance. In many challenging cases, our method
surpasses the performance of existing models, highlighting its practical
significance for diverse real-world applications.
更多查看译文
AI 理解论文
溯源树
样例
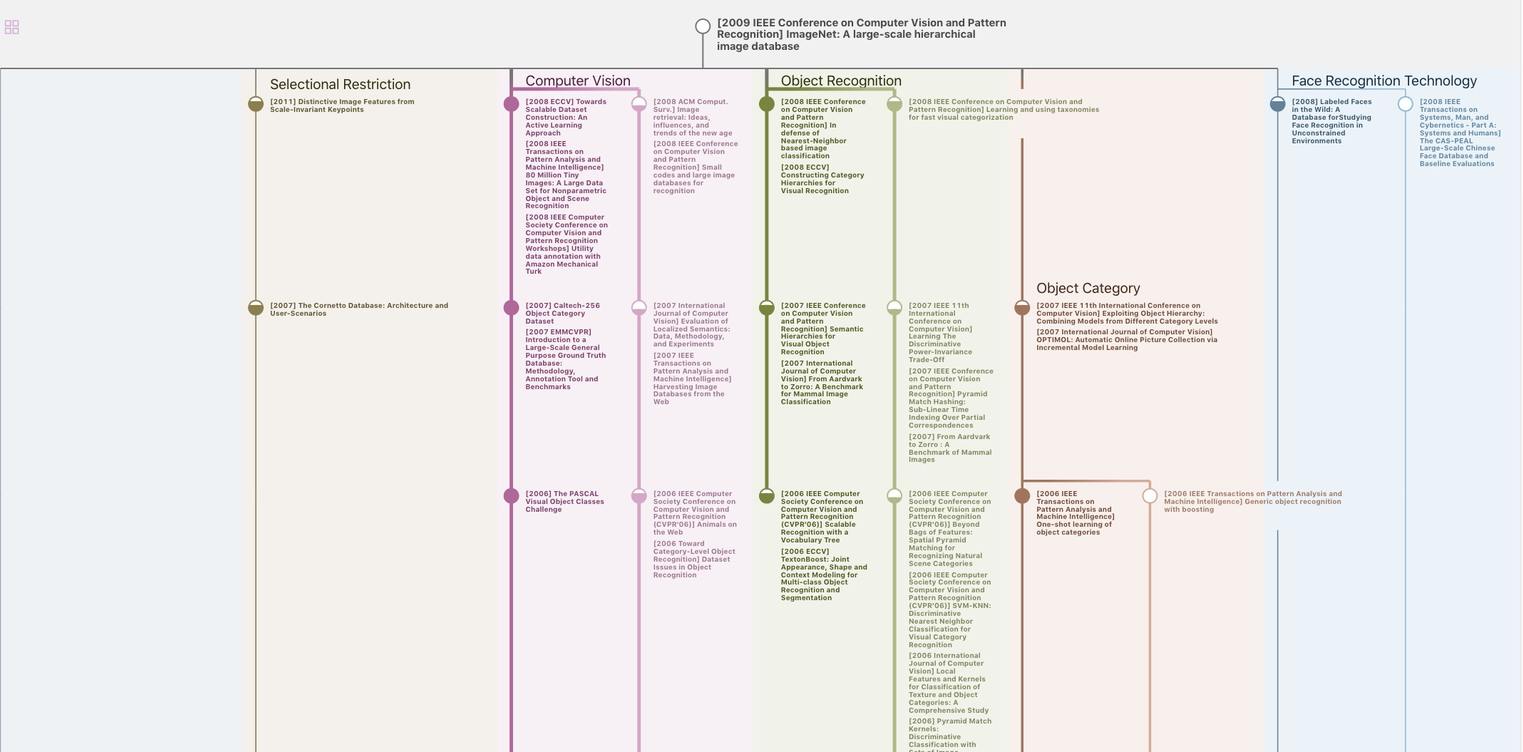
生成溯源树,研究论文发展脉络
Chat Paper
正在生成论文摘要