SAGESDA: Multi-GraphSAGE networks for predicting SnoRNA-disease associations
CURRENT RESEARCH IN STRUCTURAL BIOLOGY(2024)
摘要
Over the years, extensive research has highlighted the functional roles of small nucleolar RNAs in various biological processes associated with the development of complex human diseases. Therefore, understanding the existing relationships between different snoRNAs and diseases is crucial for advancing disease diagnosis and treatment. However, classical biological experiments for identifying snoRNA-disease associations are expensive and time-consuming. Therefore, there is an urgent need for cost-effective computational techniques that can enhance the efficiency and accuracy of prediction. While several computational models have already been proposed, many suffer from limitations and suboptimal performance. In this study, we introduced a novel Graph Neural Network-based (GNN) classification model, called SAGESDA, which is implemented through the GraphSAGE architecture with attention for the prediction of snoRNA-disease associations. The classifier leverages local neighbouring nodes in a heterogeneous network to generate new node embeddings through message passing. The mini-batch gradient descent technique was applied to divide the graph into smaller sub-graphs, which enhances the model's accuracy, speed and scalability. With these advancements, SAGESDA attained an area under the receiver operating characteristic (ROC) curve (AUC) of 0.92 using the standard dot product classifier, surpassing previous related studies. This notable performance demonstrates that SAGESDA is a promising model for predicting unknown snoRNA-disease associations with high accuracy. The SAGESDA implementation details can be obtained from https://github.com/momanyibiffon/SAGESDA.git.
更多查看译文
关键词
Small nucleolar RNAs,Diseases,snoRNA-disease associations,GraphSAGE,Heterogeneous network
AI 理解论文
溯源树
样例
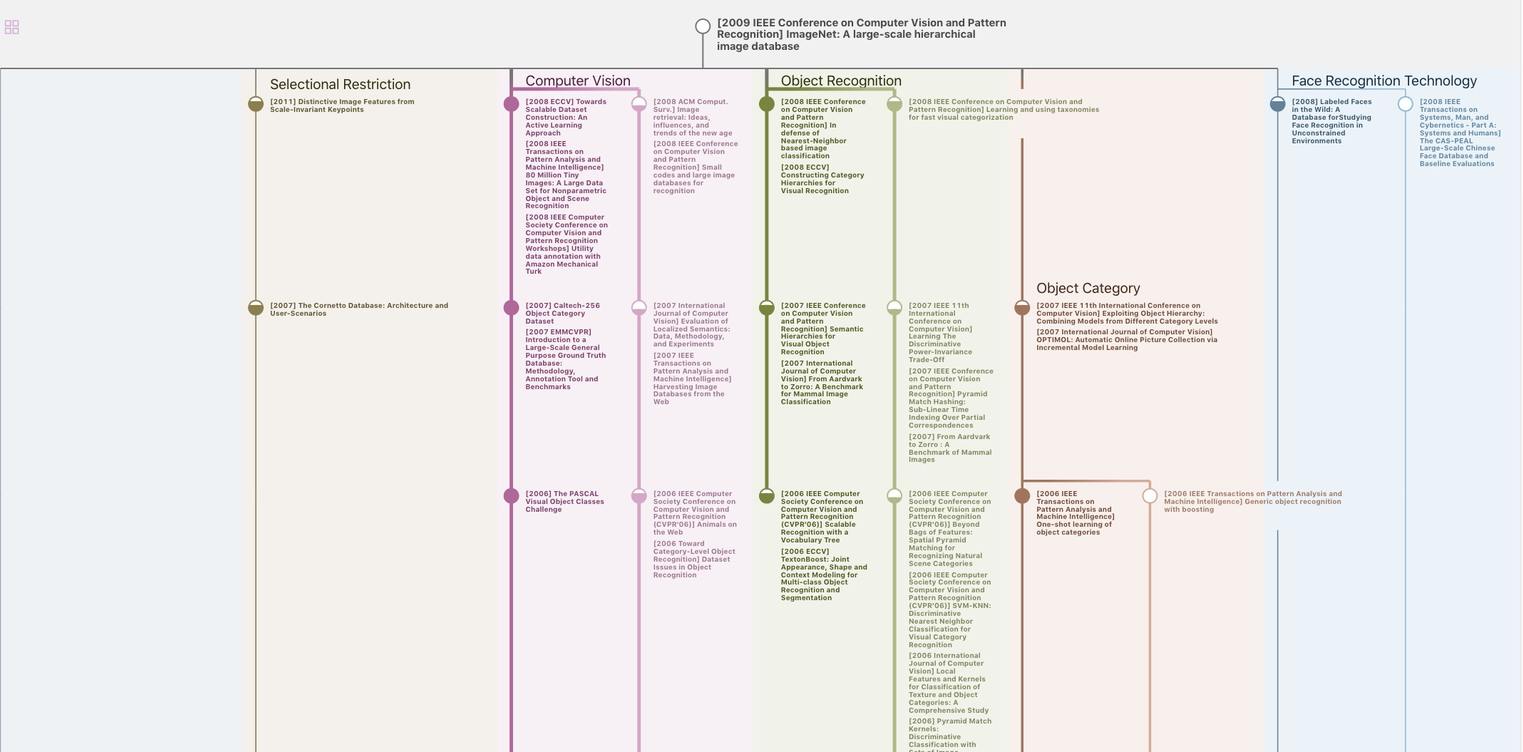
生成溯源树,研究论文发展脉络
Chat Paper
正在生成论文摘要