GTI: A Scalable Graph-based Trajectory Imputation
31ST ACM SIGSPATIAL INTERNATIONAL CONFERENCE ON ADVANCES IN GEOGRAPHIC INFORMATION SYSTEMS, ACM SIGSPATIAL GIS 2023(2023)
摘要
GPS-enabled devices, including vehicles, smartphones, wearable and tracking devices, as well as various check-in and social network data are continuously producing tremendous amounts of trajectory data, which are used consistently in many applications such as urban planning and map inference. Existing techniques for trajectory data imputation rely heavily on the existing maps to perform map-matching operations. However, modern applications such as map construction and map update assume no map exists. In this paper, we propose GTI - a scalable graph-based trajectory imputation approach for trajectory data completion. GTI relies on cross-trajectory imputation, as it exploits "mutual information" of the aggregated knowledge of all input sparse trajectories to impute the missing data for each single one of them. GTI can act as a pre-processing step for any trajectory data management system or trajectory-based application, as it takes raw sparse trajectory data as its input and outputs dense imputed trajectory data that significantly increase the accuracy of different systems that consume trajectory data. We evaluate GTI on junction-scale as well as city-scale real datasets. In addition, GTI is used as a pre-processing step in multiple trajectory-based applications and it boosts the accuracy across these applications compared with the state-of-the-art work.
更多查看译文
关键词
trajectory imputation,GPS,spatial data,road network
AI 理解论文
溯源树
样例
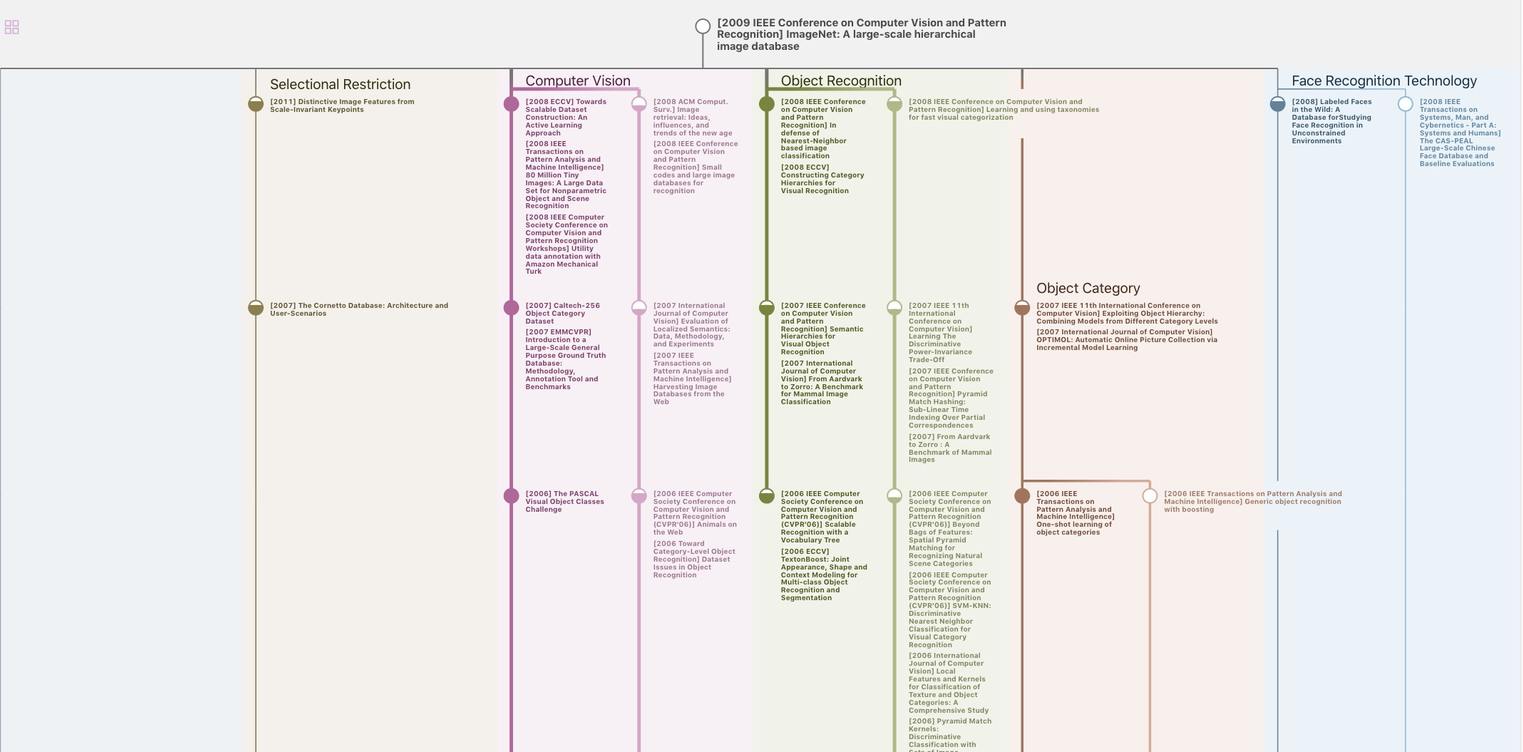
生成溯源树,研究论文发展脉络
Chat Paper
正在生成论文摘要