Statistical Inference for Spatial Regionalization
31ST ACM SIGSPATIAL INTERNATIONAL CONFERENCE ON ADVANCES IN GEOGRAPHIC INFORMATION SYSTEMS, ACM SIGSPATIAL GIS 2023(2023)
摘要
The process of regionalization involves clustering a set of spatial areas into spatially contiguous regions. Given the NP-hard nature of regionalization problems, all existing algorithms yield approximate solutions. To ascertain the quality of these approximations, it is crucial for domain experts to obtain statistically significant evidence on optimizing the objective function, in comparison to a random reference distribution derived from all potential sample solutions. In this paper, we propose a novel spatial regionalization problem, denoted as SISR (Statistical Inference for Spatial Regionalization), which generates random sample solutions with a predetermined region cardinality. The driving motivation behind SISR is to conduct statistical inference on any given regionalization scheme. To address SISR, we present a parallel technique named PRRP (P-Regionalization through Recursive Partitioning). PRRP operates over three phases: the region growing phase constructs initial regions with a predefined cardinality, while the region merging and region splitting phases ensure the spatial contiguity of unassigned areas, allowing for the growth of subsequent regions with predefined cardinalites. An extensive evaluation shows the effectiveness of PRRP using various real datasets.
更多查看译文
关键词
Statistical Inference,Regionalization,Spatial Clustering
AI 理解论文
溯源树
样例
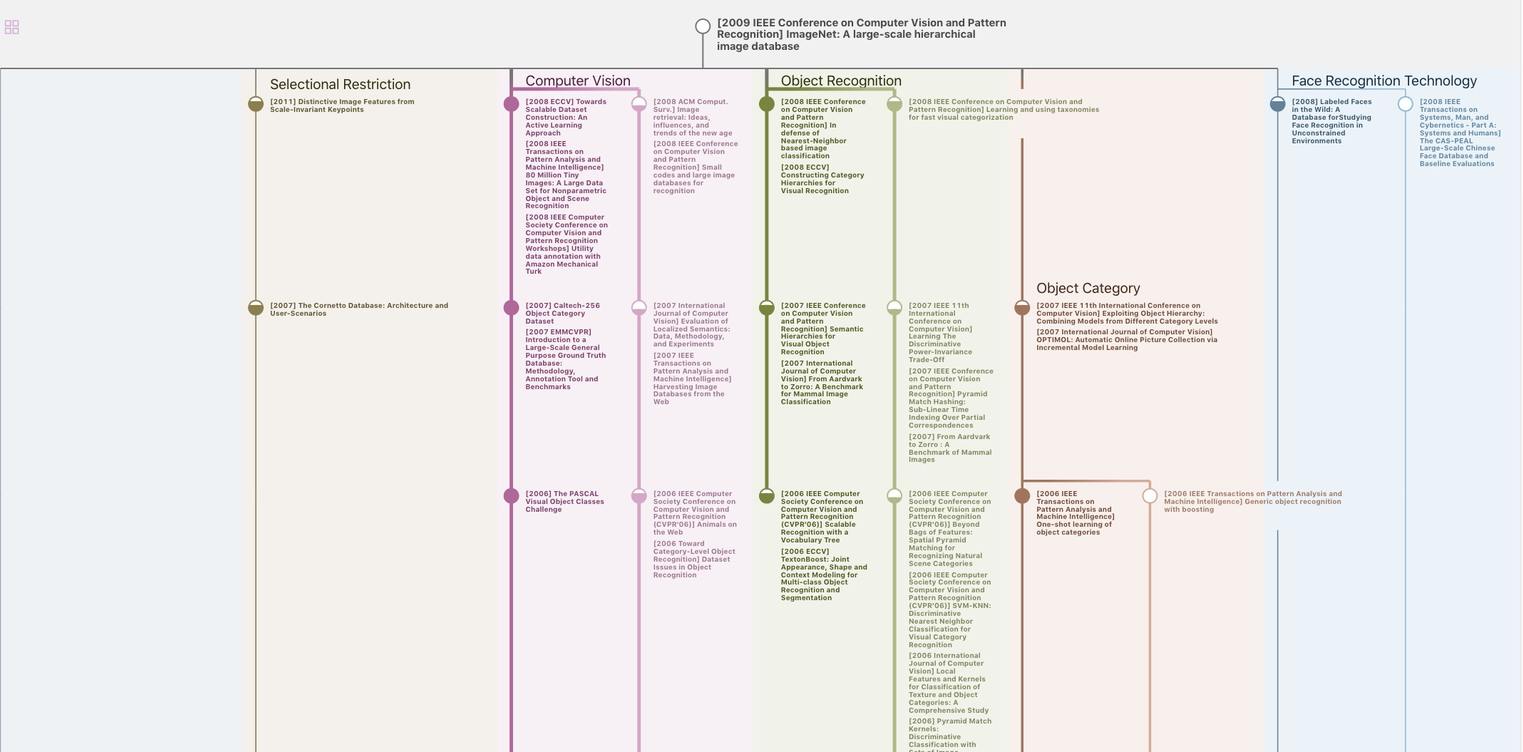
生成溯源树,研究论文发展脉络
Chat Paper
正在生成论文摘要