Combining Convolution Neural Networks with Long-Short Time Memory Layers to Predict Parkinson's Disease Progression
CoRR(2023)
摘要
Parkinson's disease is a neurological condition that occurs in nearly 1
the world's population. The disease is manifested by a drop in dopamine
production, symptoms are cognitive and behavioural and include a wide range of
personality changes, depressive disorders, memory problems, and emotional
dysregulation, which can occur as the disease progresses. Early diagnosis and
accurate staging of the disease are essential to apply the appropriate
therapeutic approaches to slow cognitive and motor decline.
Currently, there is not a single blood test or biomarker available to
diagnose Parkinson's disease. Magnetic resonance imaging has been used for the
past three decades to diagnose and distinguish between PD and other
neurological conditions. However, in recent years new possibilities have
arisen: several AI algorithms have been developed to increase the precision and
accuracy of differential diagnosis of PD at an early stage.
To our knowledge, no AI tools have been designed to identify the stage of
progression. This paper aims to fill this gap. Using the "Parkinson's
Progression Markers Initiative" dataset, which reports the patient's MRI and an
indication of the disease stage, we developed a model to identify the level of
progression. The images and the associated scores were used for training and
assessing different deep-learning models. Our analysis distinguished four
distinct disease progression levels based on a standard scale (Hoehn and Yah
scale). The final architecture consists of the cascading of a 3DCNN network,
adopted to reduce and extract the spatial characteristics of the RMI for
efficient training of the successive LSTM layers, aiming at modelling the
temporal dependencies among the data.
Our results show that the proposed 3DCNN + LSTM model achieves
state-of-the-art results by classifying the elements with 91.90% as macro
averaged OVR AUC on four classes
更多查看译文
AI 理解论文
溯源树
样例
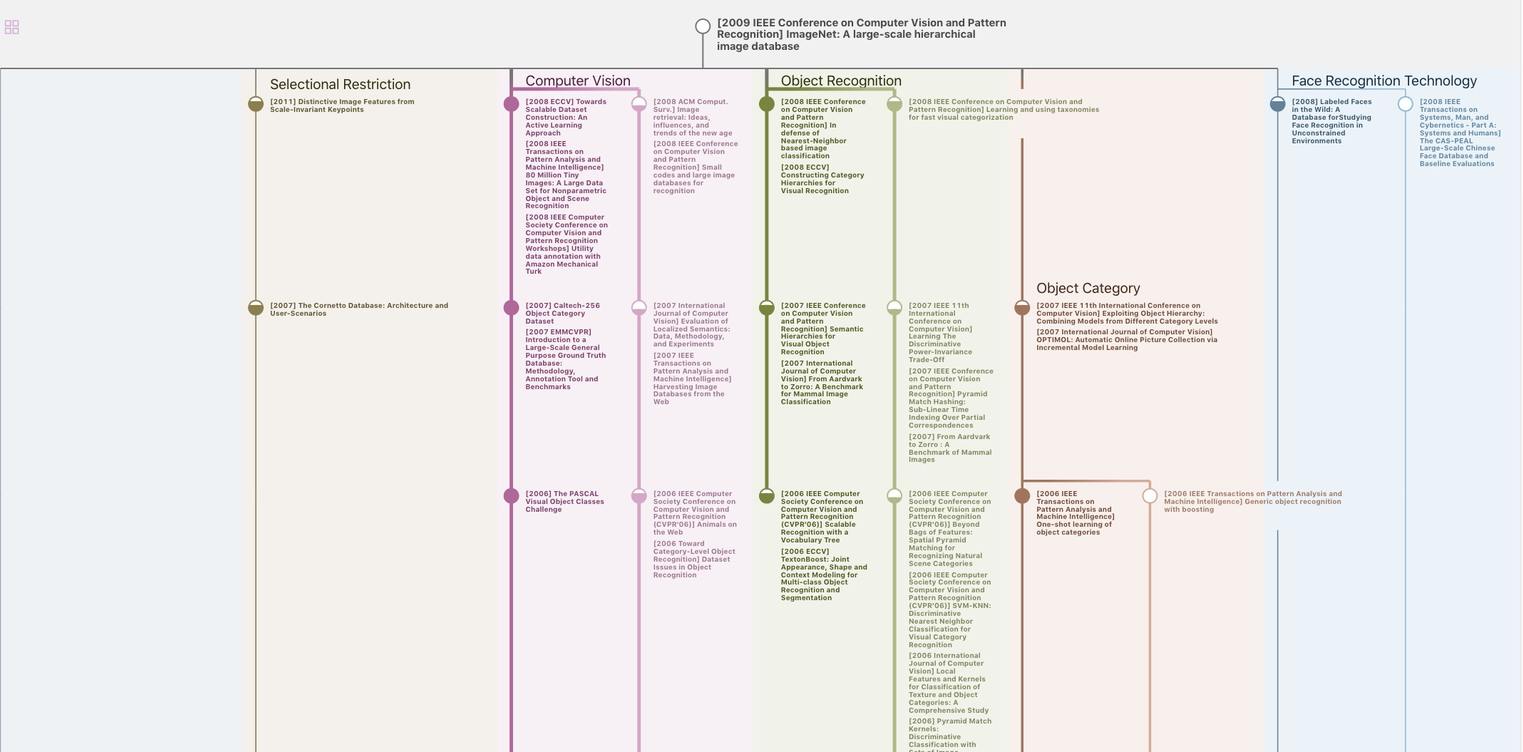
生成溯源树,研究论文发展脉络
Chat Paper
正在生成论文摘要