PanGu-π: Enhancing Language Model Architectures via Nonlinearity Compensation
CoRR(2023)
摘要
The recent trend of large language models (LLMs) is to increase the scale of
both model size (the number of parameters) and dataset to achieve better
generative ability, which is definitely proved by a lot of work such as the
famous GPT and Llama. However, large models often involve massive computational
costs, and practical applications cannot afford such high prices. However, the
method of constructing a strong model architecture for LLMs is rarely
discussed. We first analyze the state-of-the-art language model architectures
and observe the feature collapse problem. Based on the theoretical analysis, we
propose that the nonlinearity is also very important for language models, which
is usually studied in convolutional neural networks for vision tasks. The
series informed activation function is then introduced with tiny calculations
that can be ignored, and an augmented shortcut is further used to enhance the
model nonlinearity. We then demonstrate that the proposed approach is
significantly effective for enhancing the model nonlinearity through carefully
designed ablations; thus, we present a new efficient model architecture for
establishing modern, namely, PanGu-π. Experiments are then conducted using
the same dataset and training strategy to compare PanGu-π with
state-of-the-art LLMs. The results show that PanGu-π-7B can achieve a
comparable performance to that of benchmarks with about 10% inference
speed-up, and PanGu-π-1B can achieve state-of-the-art performance in terms
of accuracy and efficiency. In addition, we have deployed PanGu-π-7B in the
high-value domains of finance and law, developing an LLM named YunShan for
practical application. The results show that YunShan can surpass other models
with similar scales on benchmarks.
更多查看译文
AI 理解论文
溯源树
样例
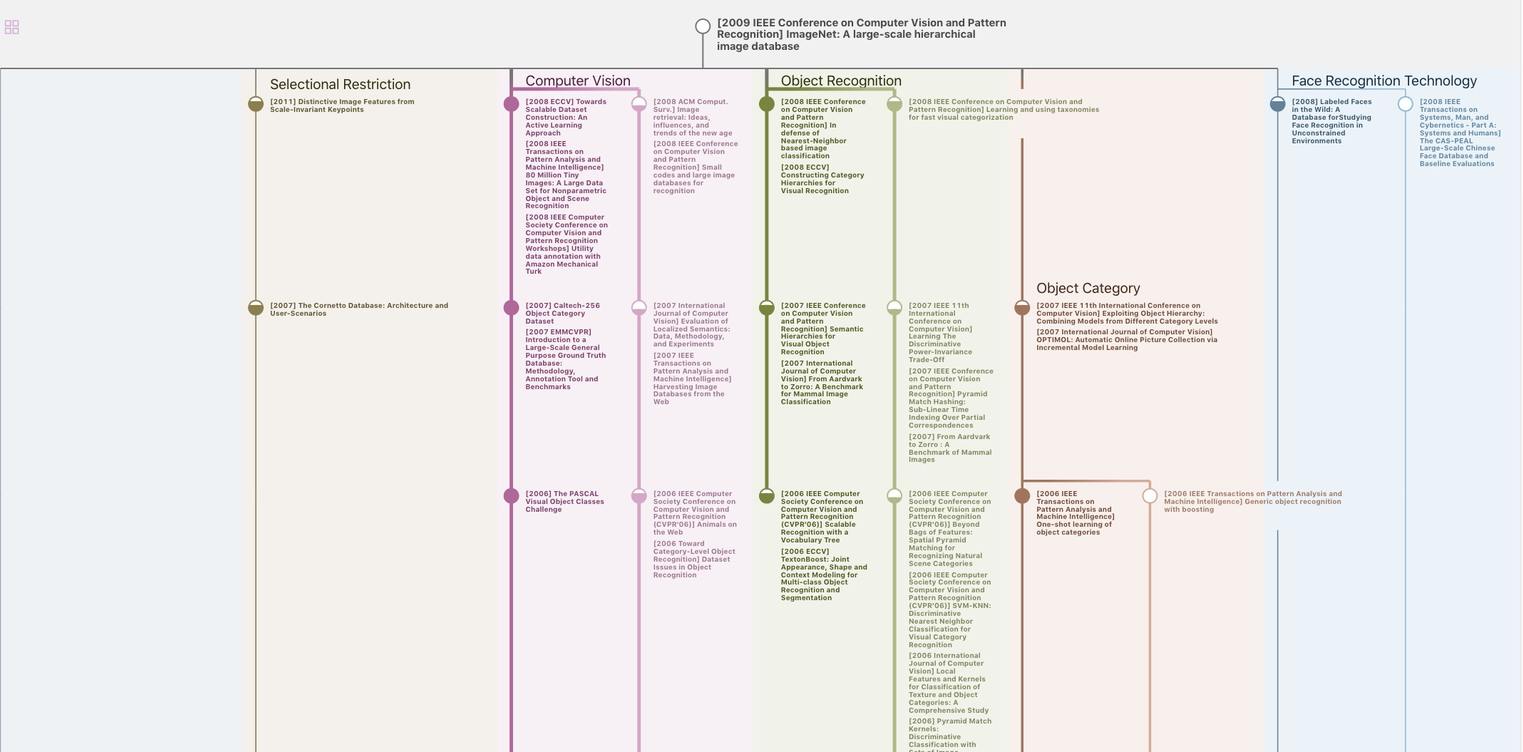
生成溯源树,研究论文发展脉络
Chat Paper
正在生成论文摘要