Deep Learning-Based Bearing Fault Classification Using Stray Magnetic Flux Signal
2023 IEEE Energy Conversion Congress and Exposition (ECCE)(2023)
摘要
Detecting existing faults in bearing systems of electric motors is of utmost importance to prevent further damage and mitigate costly repairs. This paper addresses the challenging task of identifying distributed faults, which differ from the more commonly studied single point faults. Selecting an appropriate input is crucial for accurate fault diagnosis in real-world bearing systems. While various inputs such as vibration signals on different axes (x, y, z), acoustic signals, and current signals have been explored in the literature, this research investigates the performance of stray magnetic flux signals for bearing fault classification. Another key aspect of this study is the focus on real faults rather than simulated faults commonly used in laboratory research. The motivation behind this approach lies in utilizing simple sensor boards to achieve reasonable fault classification results for real-world distributed bearing faults. Deep learning networks were chosen as the methodology due to the limitations of traditional machine learning techniques in reliably detecting fault patterns solely based on stray magnetic flux signals. The proposed deep learning algorithm demonstrates its effectiveness in extracting informative features from the flux signals, enabling accurate classification of five faulty conditions and one healthy condition with a test accuracy exceeding 94%. This highlights the success of the model in reliably identifying and categorizing different bearing fault states using the stray magnetic flux signal as the input.
更多查看译文
关键词
Bearing Fault Detection,Convolutional Neural Network (CNN),Deep Learning,Distributed Faults
AI 理解论文
溯源树
样例
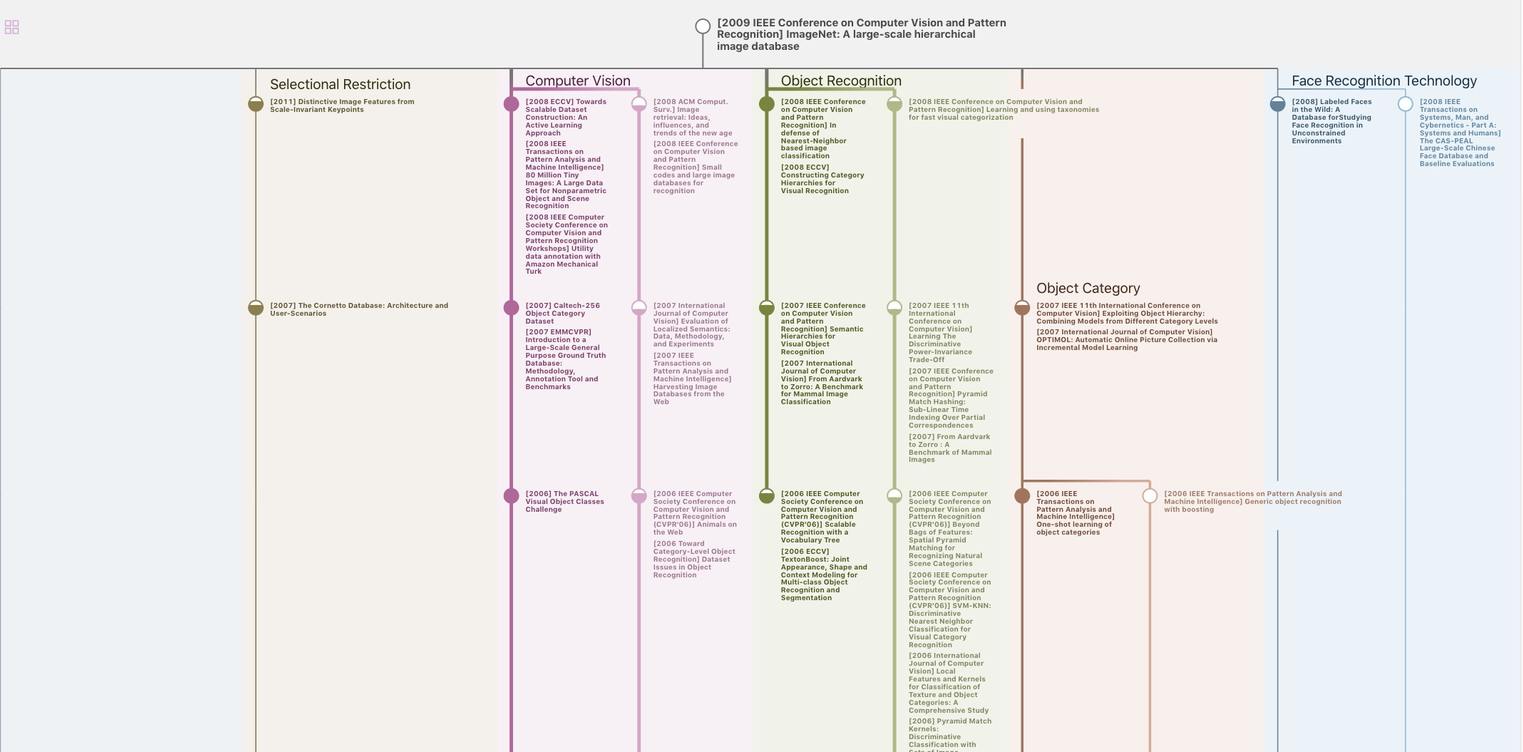
生成溯源树,研究论文发展脉络
Chat Paper
正在生成论文摘要