Multi Sensory Distributed Bearing Fault Classification using Wavelet Scattering Transform
2023 IEEE Energy Conversion Congress and Exposition (ECCE)(2023)
摘要
Distributed bearing faults are a prevalent issue in the industrial sector, primarily attributed to factors such as inadequate lubrication, contamination, electrical erosion, and propagation of localized faults. This research offers a multi-signal fault diagnosis framework aimed at identifying and categorizing such faults in induction machines. The framework leverages the wavelet scattering transform to extract feature sets from nonstationary signals, including tri-axial vibrations and stray magnetic flux signals. Data for this investigation are collected from two induction motors, with capacities of 3 and 10 horsepower respectively. The investigation includes a comprehensive analysis across 50 unique operating scenarios, covering ten different rotational speeds and five distinct torque levels. To reduce the redundancies among features, two dimension reduction algorithms such as Principal Component Analysis (PCA) and Linear Discriminant Analysis (LDA)are employed and compared. Ensemble classification techniques, namely XGBoost and Random Forest, are used for the classification of distributed faults. Various combinations of dimensionality reduction methods and classifiers are examined to ascertain the most efficient approach in terms of accuracy and training time performance. Additionally, the efficacy of different signal combinations is also studied. Empirical data underscores the effectiveness of combining LDA and Random Forest, achieving an optimal accuracy of 99.4% with a minimum training duration of 15 seconds. Notably, the utilization of vibration signals from the X, Y, and Z axes yielded an accuracy rate above 90%. However, the standalone effectiveness of the stray magnetic flux was found to be minimal.
更多查看译文
关键词
Bearing fault detection,wavelet scattering transform,Distributed bearing fault
AI 理解论文
溯源树
样例
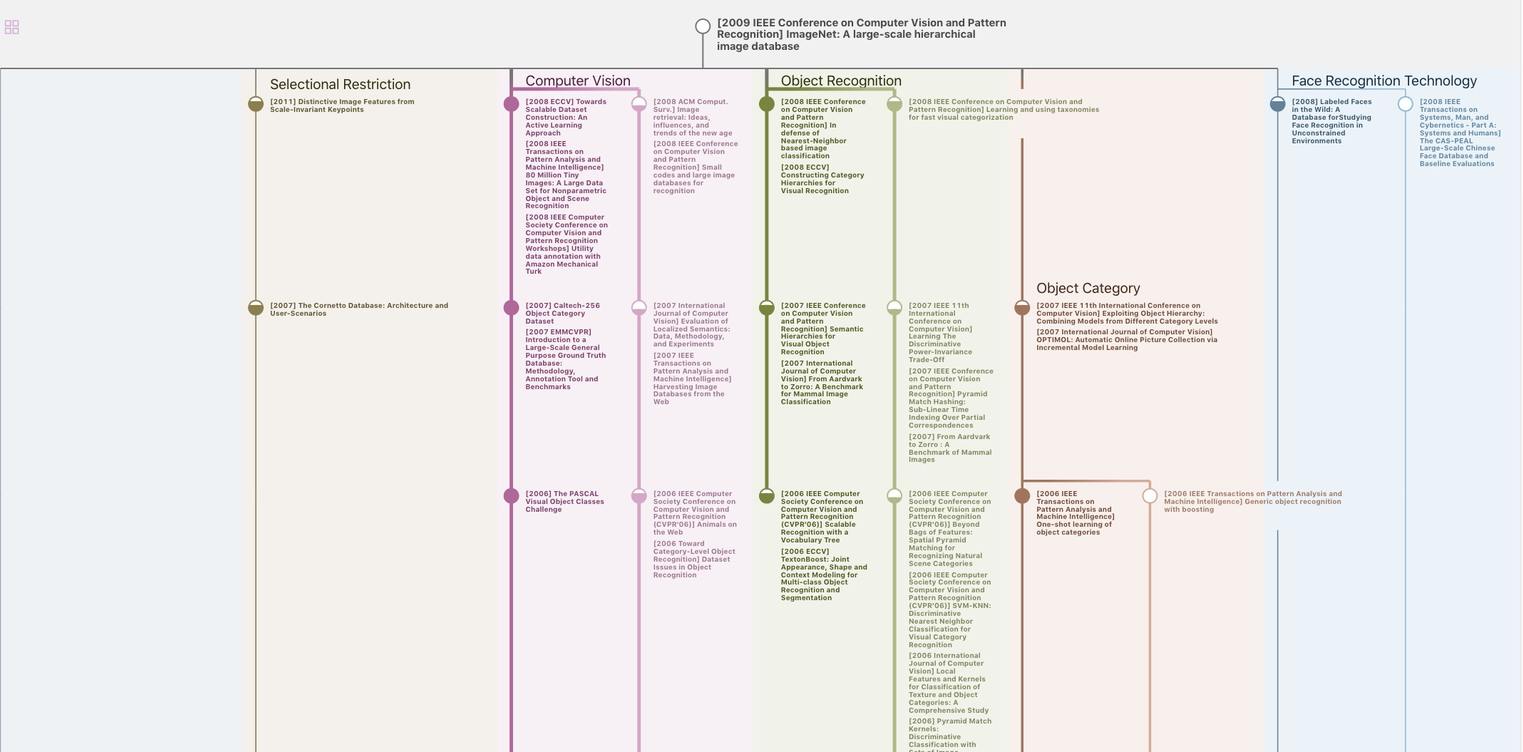
生成溯源树,研究论文发展脉络
Chat Paper
正在生成论文摘要