Instantaneous estimation of momentary affective responses using neurophysiological signals and a spatiotemporal emotional intensity regression network
NEURAL NETWORKS(2024)
摘要
Previous studies in affective computing often use a fixed emotional label to train an emotion classifier with electroencephalography (EEG) from individuals experiencing an affective stimulus. However, EEGs encode emotional dynamics that include varying intensities within a given emotional category. To investigate these variations in emotional intensity, we propose a framework that obtains momentary affective labels for fine-grained segments of EEGs with human feedback. We then model these labeled segments using a novel spatiotemporal emotional intensity regression network (STEIR-Net). It integrates temporal EEG patterns from nine predefined cortical regions to provide a continuous estimation of emotional intensity. We demonstrate that the STEIR-Net outperforms classical regression models by reducing the root mean square error (RMSE) by an average of 4 similar to 9 % and 2 similar to 4 % for the SEED and SEED-IV databases, respectively. We find that the frontal and temporal cortical regions contribute significantly to the affective intensity's variation. Higher absolute values of the Spearman correlation coefficient between the model estimation and momentary affective labels under happiness (0.2114) and fear (0.2072) compared to neutral (0.1694) and sad (0.1895) emotions were observed. Besides, increasing the input length of the EEG segments from 4 to 20 s further reduces the RMSE from 1.3548 to 1.3188.
更多查看译文
关键词
Affective computing,electroencephalogram,Biomedical signal processing,Machine learning,Deep learning
AI 理解论文
溯源树
样例
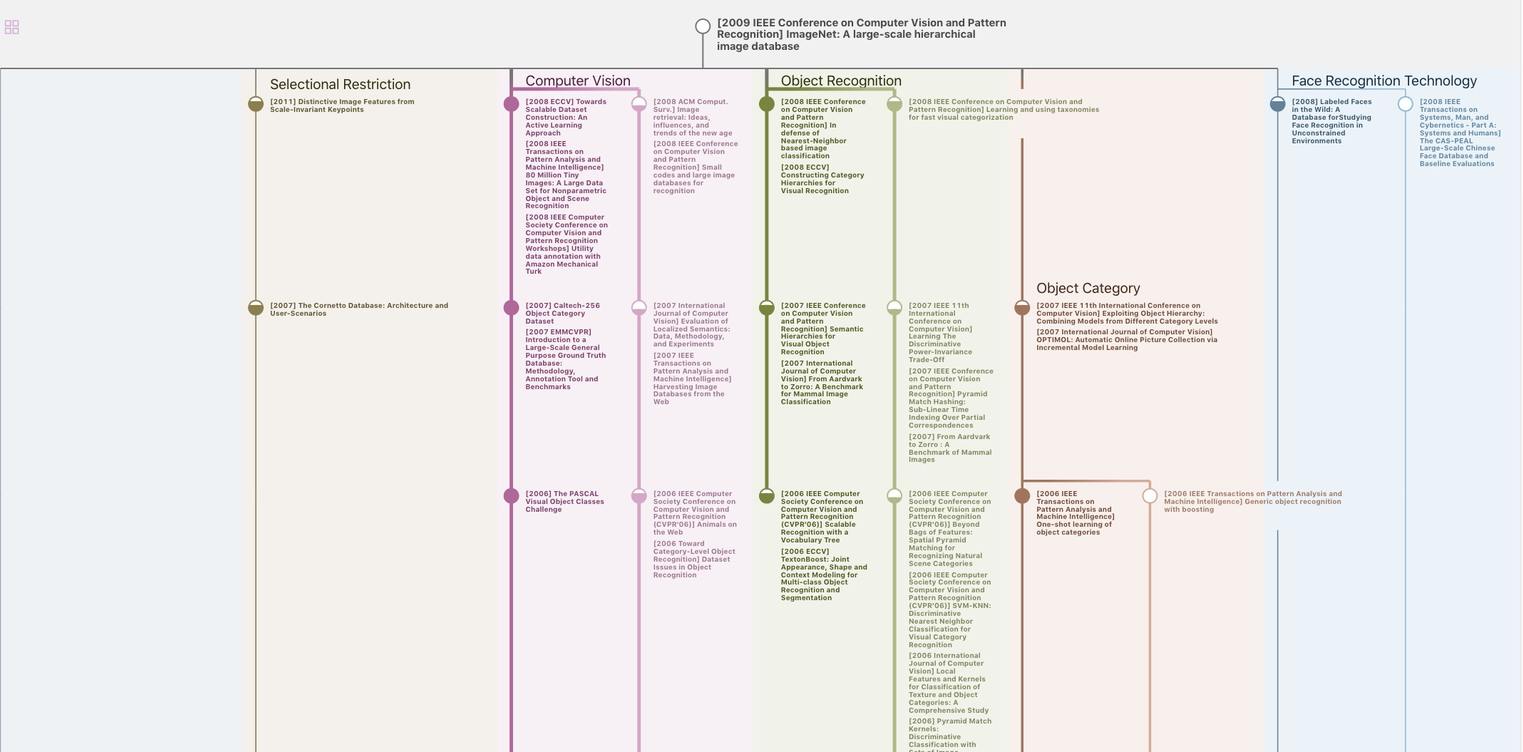
生成溯源树,研究论文发展脉络
Chat Paper
正在生成论文摘要