Ship Object Detection and Classification Based on Improved YOLOv5
2023 13th International Conference on Information Science and Technology (ICIST)(2023)
摘要
In recent years, with the rapid development of the marine economy, maritime transportation industry has also steadily strengthened, leading to an increasing number of ships at sea. However, this growth has also raised safety risks for maritime vessels, making accurate and effective maritime vessel recognition technology essential for improving navigational safety. Presently, most target detection algorithms focus solely on improving model accuracy while neglecting the computational and parameter overheads resulting from the complexity of the models. To address this issue, this paper proposes an improved YOLOv5 object detection algorithm, utilizing MobileN et V3 for lightweight enhancements to minimize model size and computational demands. Additionally, to ensure model precision, Hybrid Channel Attention Mechanism (CBAM) is introduced. Experiments were conducted on the Seaships dataset, comparing the proposed improved YOLOv5 algorithm with YOLOv5 and Faster R-CNN algorithms. The experimental results demonstrate that the enhanced YOLOv5 detection network achieves accuracy of 99.1 % and mean average precision of 99.5%. Compared to other algorithms, it exhibits excellent detection performance.
更多查看译文
关键词
Ship Target Detection,Attention Mechanism,YOLOv5,Convolutional Neural Network,Lightweight Design
AI 理解论文
溯源树
样例
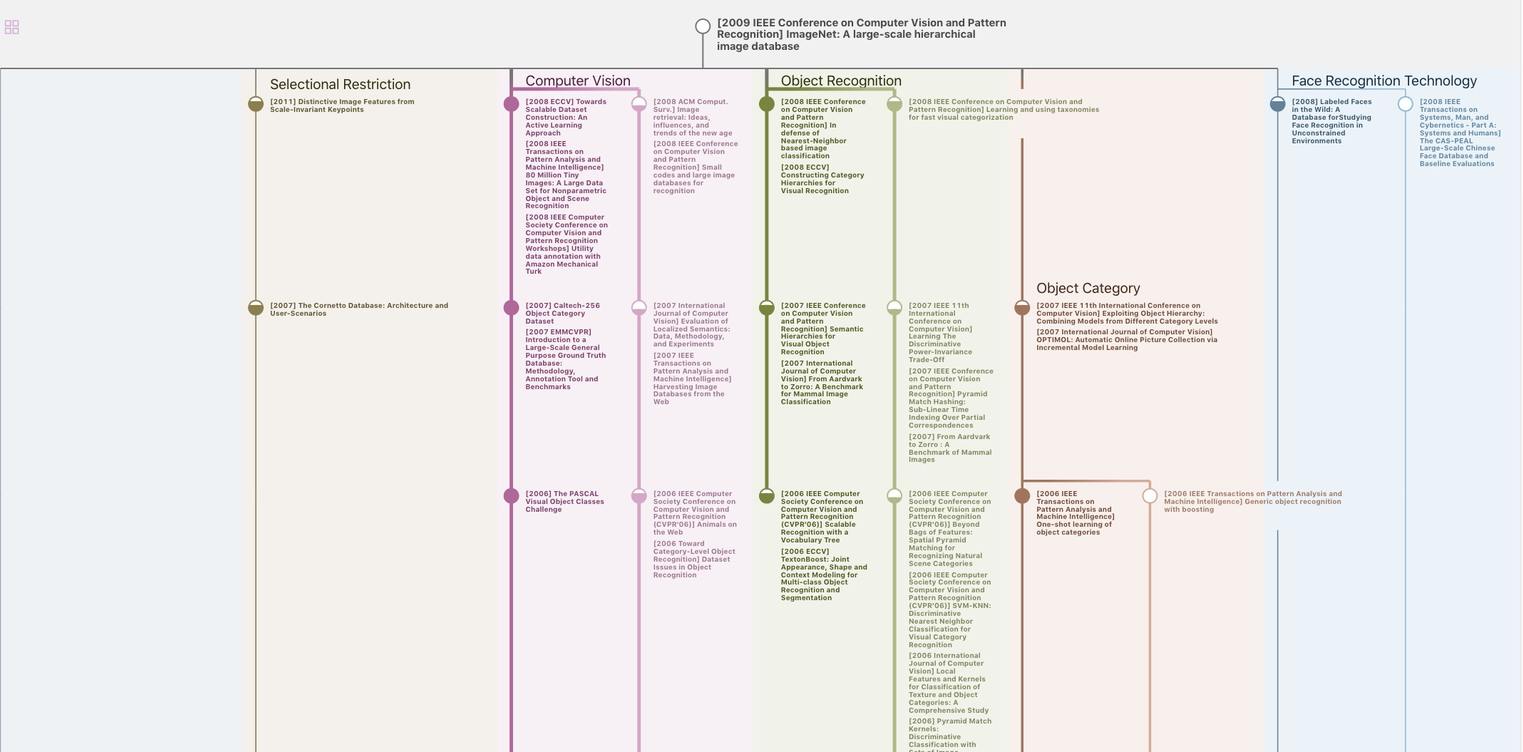
生成溯源树,研究论文发展脉络
Chat Paper
正在生成论文摘要