Packet-Level Intrusion Detection Using LSTM Focusing on Personal Information and Payloads.
2023 18th Asia Joint Conference on Information Security (AsiaJCIS)(2023)
摘要
In recent years, network-based intrusion detection systems (NIDS) based on advanced neural network (NN) technologies have emerged. In the previous study (Hwang et al. 2019), higher classification accuracy than conventional NIDS models was achieved by an NN-based NIDS using features from strings contained in the header information of communication packets. It used LSTM to learn the time series extracted from the packets. However, the data used for training contained information about the attacker’s devices, which we could not utilize in real situations. Therefore, in this study, we conducted experiments using the LSTM model to hide the MAC addresses, IP addresses, and port numbers from the headers, which are the attacker’s personal information. The results show that the accuracy of packet classification was significantly degraded, confirming that the classification results in the previous study depended on the attacker’s personal information. In addition, our experiment, in which personal information was removed and payload information was added to the classification, achieved an F1-Score of 99.0%. The result is comparable to that of the previous study.
更多查看译文
关键词
network security,intrusion detection,packet analysis,machine learning,LSTM
AI 理解论文
溯源树
样例
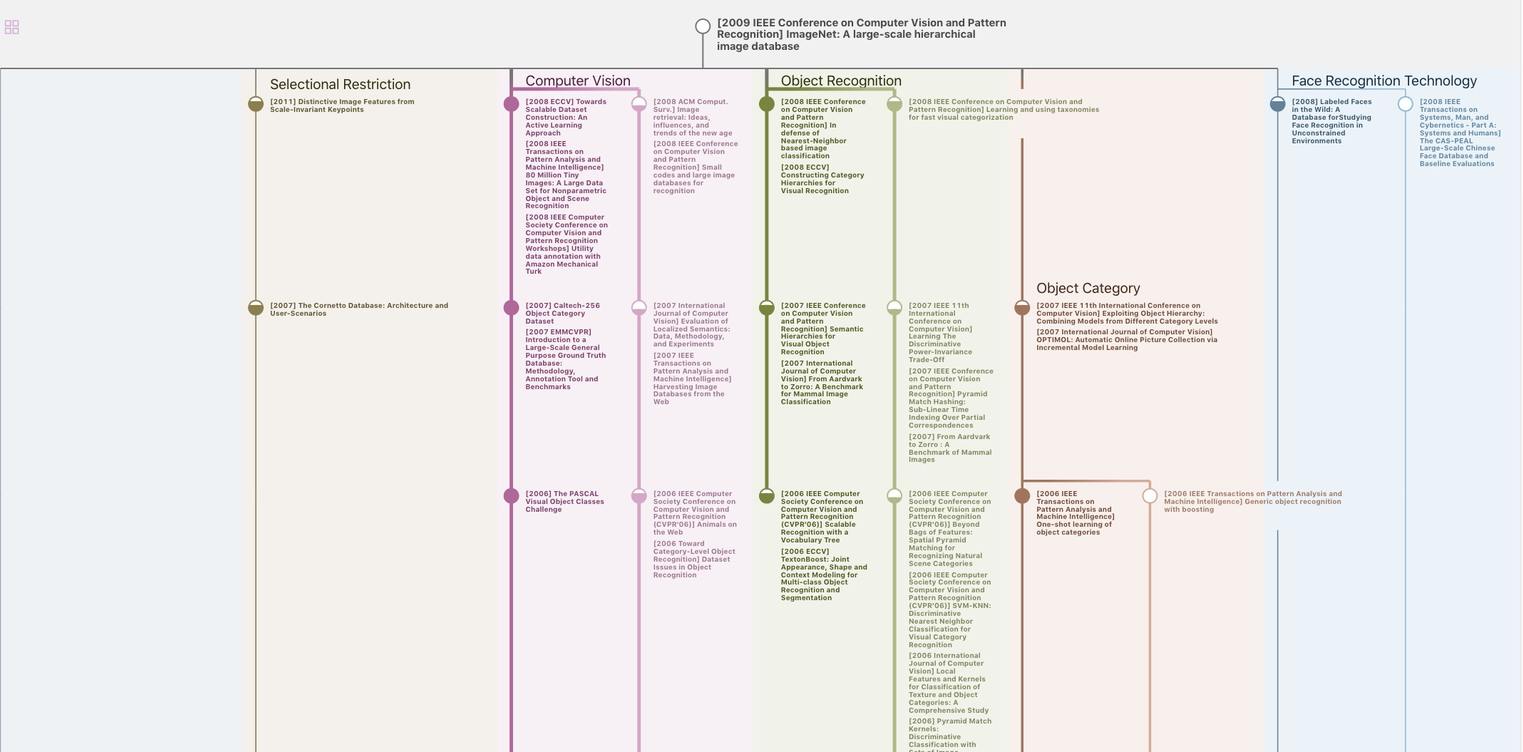
生成溯源树,研究论文发展脉络
Chat Paper
正在生成论文摘要