SENSE: Self-Evolving Learning for Self-Supervised Monocular Depth Estimation
IEEE TRANSACTIONS ON IMAGE PROCESSING(2024)
摘要
Self-supervised depth estimation methods can achieve competitive performance using only unlabeled monocular videos, but they suffer from the uncertainty of jointly learning depth and pose without any ground truths of both tasks. Supervised framework provides robust and superior performance but is limited by the scope of the labeled data. In this paper, we introduce SENSE, a novel learning paradigm for self-supervised monocular depth estimation that progressively evolves the prediction result using supervised learning, but without requiring labeled data. The key contribution of our approach stems from the novel use of the pseudo labels - the noisy depth estimation from the self-supervised methods. We surprisingly find that a fully supervised depth estimation network trained using the pseudo labels can produce even better results than its "ground truth". To push the envelope further, we then evolve the self-supervised backbone by replacing its depth estimation branch with that fully supervised network. Based on this idea, we devise a comprehensive training pipeline that alternatively enhances the two key branches (depth and pose estimation) of the self-supervised backbone network. Our proposed approach can effectively ease the difficulty of multi-task training in self-supervised depth estimation. Experimental results have shown that our proposed approach achieves state-of-the-art results on the KITTI dataset.
更多查看译文
关键词
Self-supervised,monocular depth estimation,pseudo labels,learning paradigm
AI 理解论文
溯源树
样例
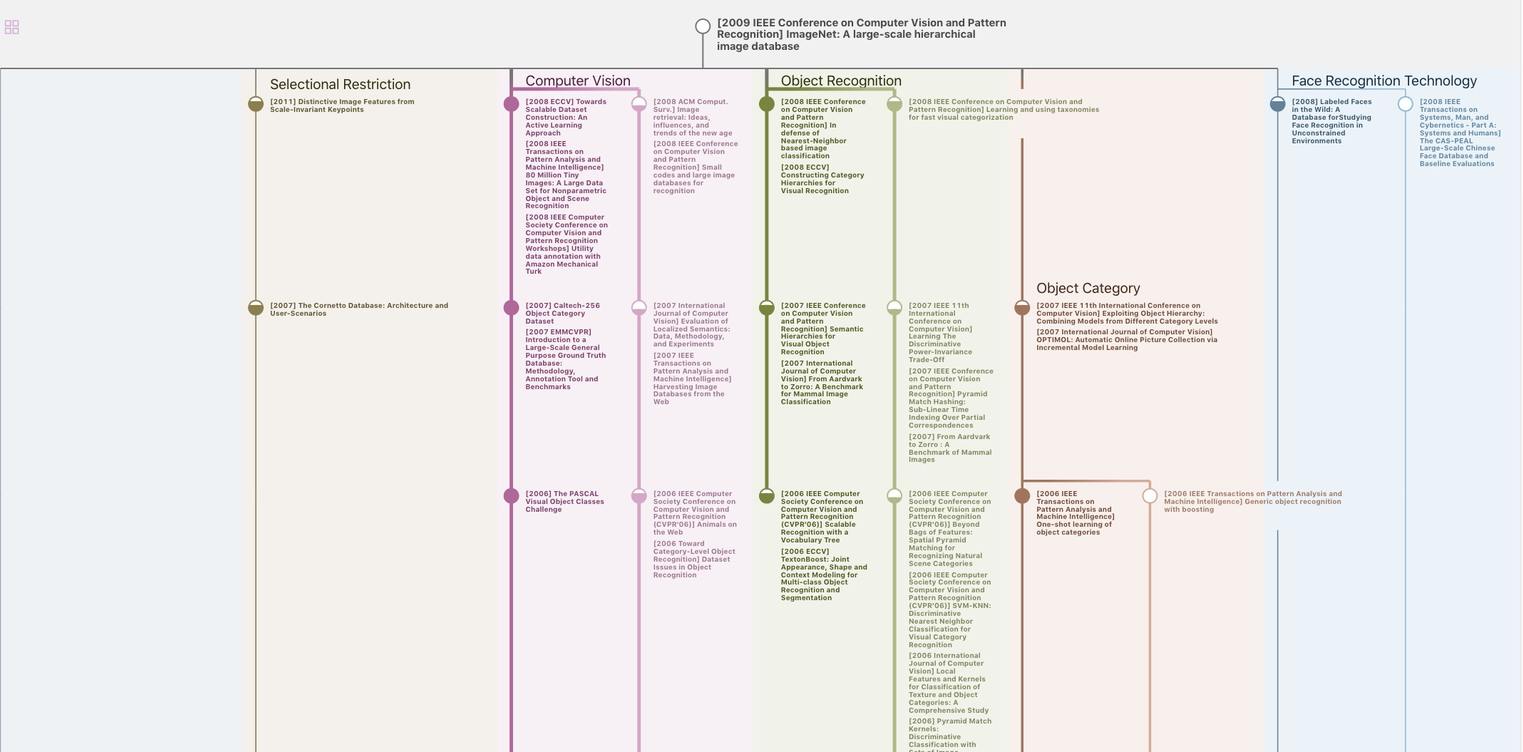
生成溯源树,研究论文发展脉络
Chat Paper
正在生成论文摘要