Multi-view robust regression for feature extraction
PATTERN RECOGNITION(2024)
摘要
Recently, Multi -view Discriminant Analysis (MVDA) has been proposed and achieves good performance in multi -view recognition tasks. However, as an extension of LDA, this method still suffers from the small -class problem and has the sensitivity to outliers. In order to address these drawbacks and achieve better performance on multi -view recognition tasks, we proposed Multi -view Robust Regression (MVRR) for multi -view feature extraction. MVRR is a regression based method that imposes L21 norm as the metric of the loss function and the regularization term to improve robustness and obtain jointly sparse projection matrices for effective feature extraction. Moreover, we incorporate an orthogonal matrix to regress the extracted features to their scaled label to avoid the small -class problem. Therefore, MVRR guarantees the projection matrix to break through the restriction of the number of class for solving the small -class problem. We also propose an iterative algorithm to compute the optimal solution of MVRR and the convergence of MVRR is proved. Experiments are conducted on four databases to verify the performance of MVRR and the result illustrates that MVRR is robust on multi -view feature extraction.
更多查看译文
关键词
Image classification,Small-class problem,Linear regression (LR)
AI 理解论文
溯源树
样例
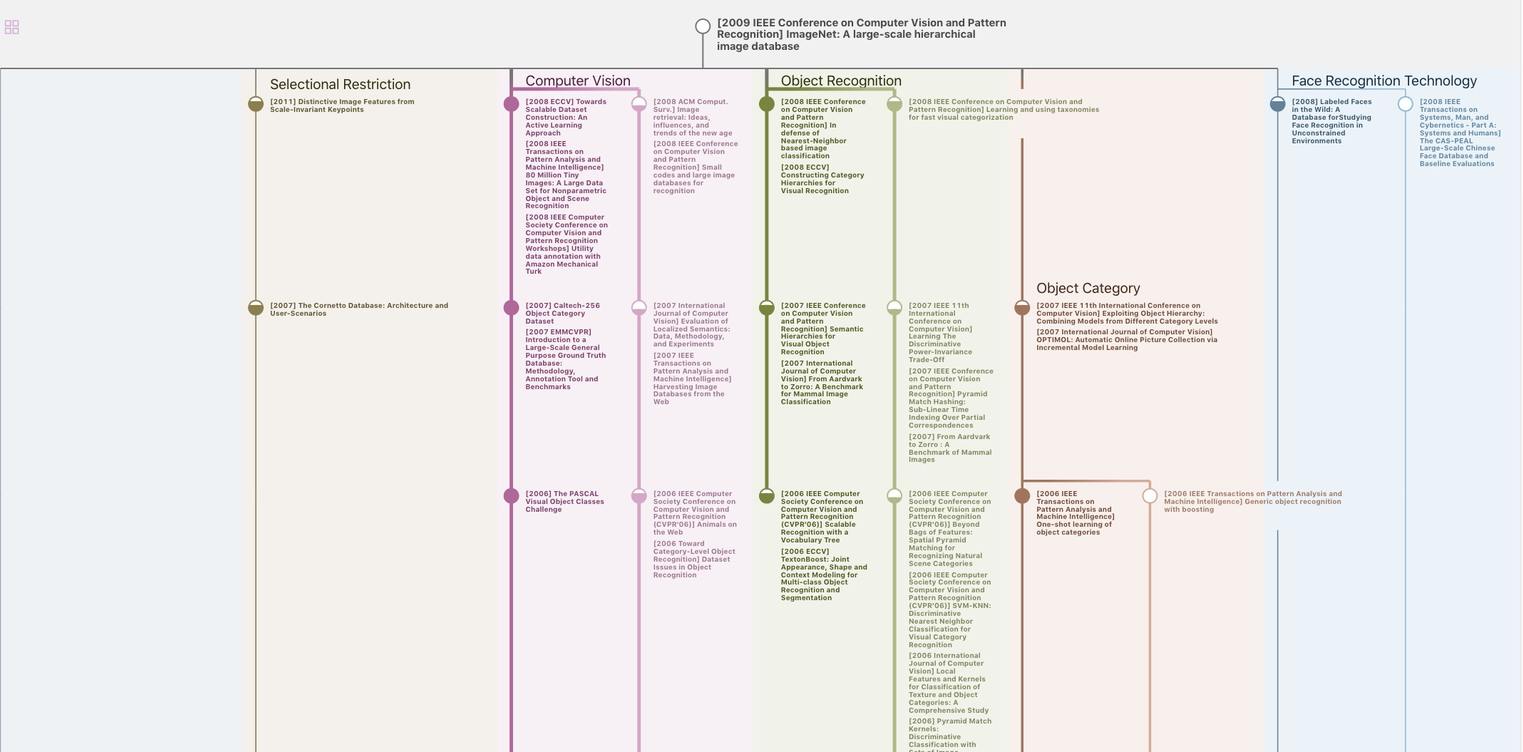
生成溯源树,研究论文发展脉络
Chat Paper
正在生成论文摘要