A new particle-swarm-optimization-assisted deep transfer learning framework with applications to outlier detection in additive manufacturing
ENGINEERING APPLICATIONS OF ARTIFICIAL INTELLIGENCE(2024)
摘要
In wire arc additive manufacturing (WAAM), the electric arc is an essential part of the welding equipment, which serves as the heat source and is directed by the current and voltage. The working status of the electric arc is an important factor in determining the quality of the fabricated components. During the welding process, the current and voltage may change abruptly due to some abnormalities in the operating conditions, which may affect the working status and thereby affect the quality of products. Such abnormal changes in the current and voltage can be treated as outliers. In order to identify outliers in current and voltage to further improve the welding process, in this paper, a novel deep-transfer-learning-embedded outlier detection approach is developed for WAAM. A new domain adaptation strategy is designed where the cross domain discrepancies of the marginal distribution and conditional distribution are minimized. Specifically, two separate coefficients are introduced to adjust the conditional domain discrepancies of normal instances and outliers with the purpose of alleviating the data imbalance problem. The particle swarm optimizer is employed to adjust the hyper-parameters. The developed deep transfer learning framework is exploited in designing a new outlier detector with application to WAAM. The proposed approach is exploited in real-world industrial data collected through the WAAM process. Experimental results demonstrate that the proposed outlier detection approach outperforms the standard deep-learning-based outlier detector approach and the standard transfer-learning-embedded outlier detection approach in terms of detection accuracy.
更多查看译文
关键词
Outlier detection,Deep transfer learning,Particle swarm optimization,Industrial data analysis,Additive manufacturing
AI 理解论文
溯源树
样例
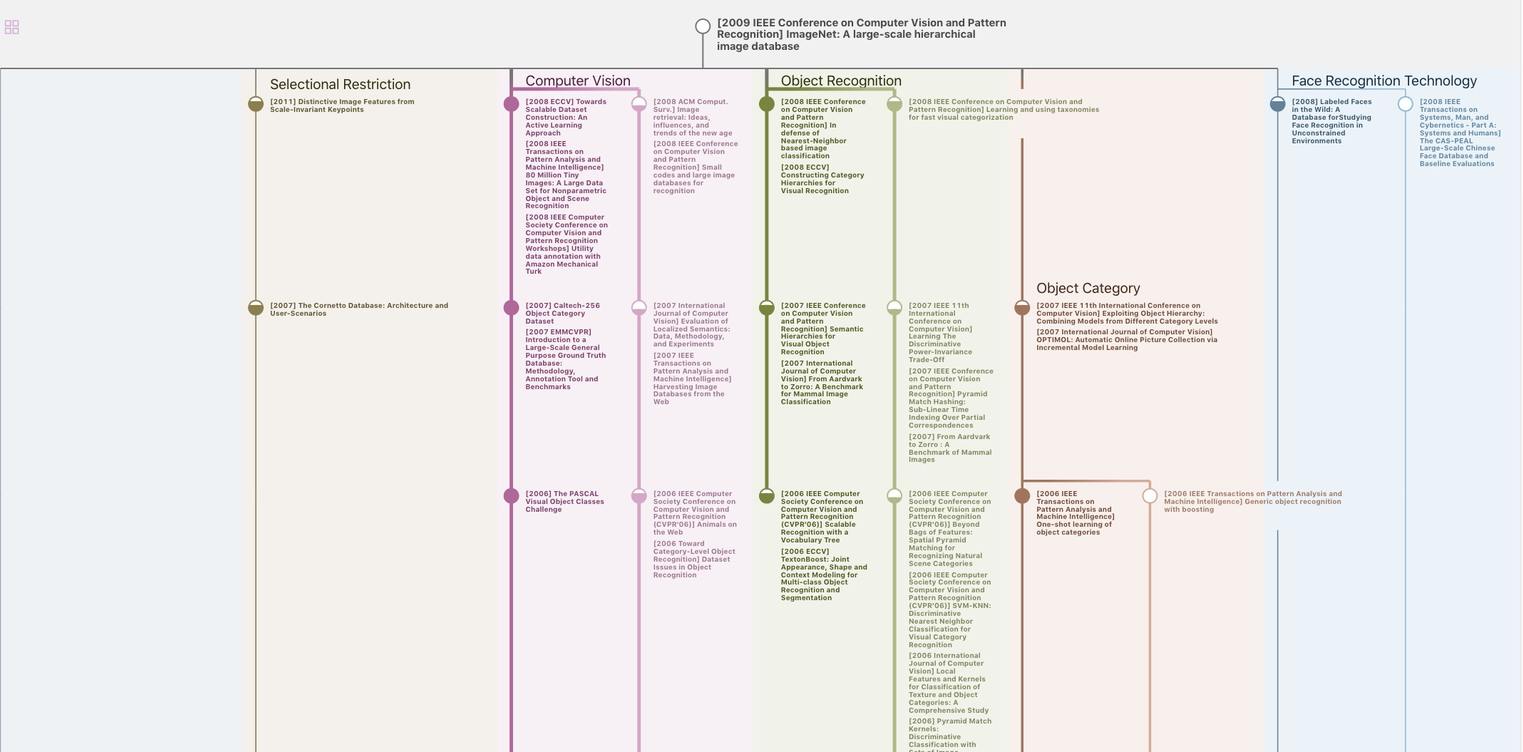
生成溯源树,研究论文发展脉络
Chat Paper
正在生成论文摘要