A joint learning method with consistency-aware for low-resolution facial expression recognition
EXPERT SYSTEMS WITH APPLICATIONS(2024)
摘要
Existing facial expression recognition (FER) methods are mainly devoted to learning discriminative features from high-resolution images. However, when applied to low-resolution images, their performance drops rapidly. This paper proposes a unified learning framework (namely SR-FER) by cascading the image super-resolution (SR) task and FER task to alleviate the low-resolution challenge. It effectively feeds back expression-related information from the FER network to the SR network, and returns the quality-enhanced expression images via a SR network. Specifically, a multi-stage attention-aware consistency loss module is introduced to help the SR network achieve discriminative feature restoration guided by attention information. Furthermore, a prediction consistency loss module is also developed to encourage the SR network to restore discriminative features by reducing the difference in prediction information between the restored and original normal-resolution images. Therefore, more accurate results are obtained by performing FER on the restored images. We conduct extensive experiments to demonstrate that the proposed low-resolution FER solution can help SR methods restore features favorable for FER while maintaining acceptable FER performance in various resolution degradation scenarios. The proposed method effectively improves the FER challenge under resolution degradation conditions, which is of good reference value for real-world applications.
更多查看译文
关键词
Facial expression recognition,Image super-resolution,Deep learning,High-level vision task
AI 理解论文
溯源树
样例
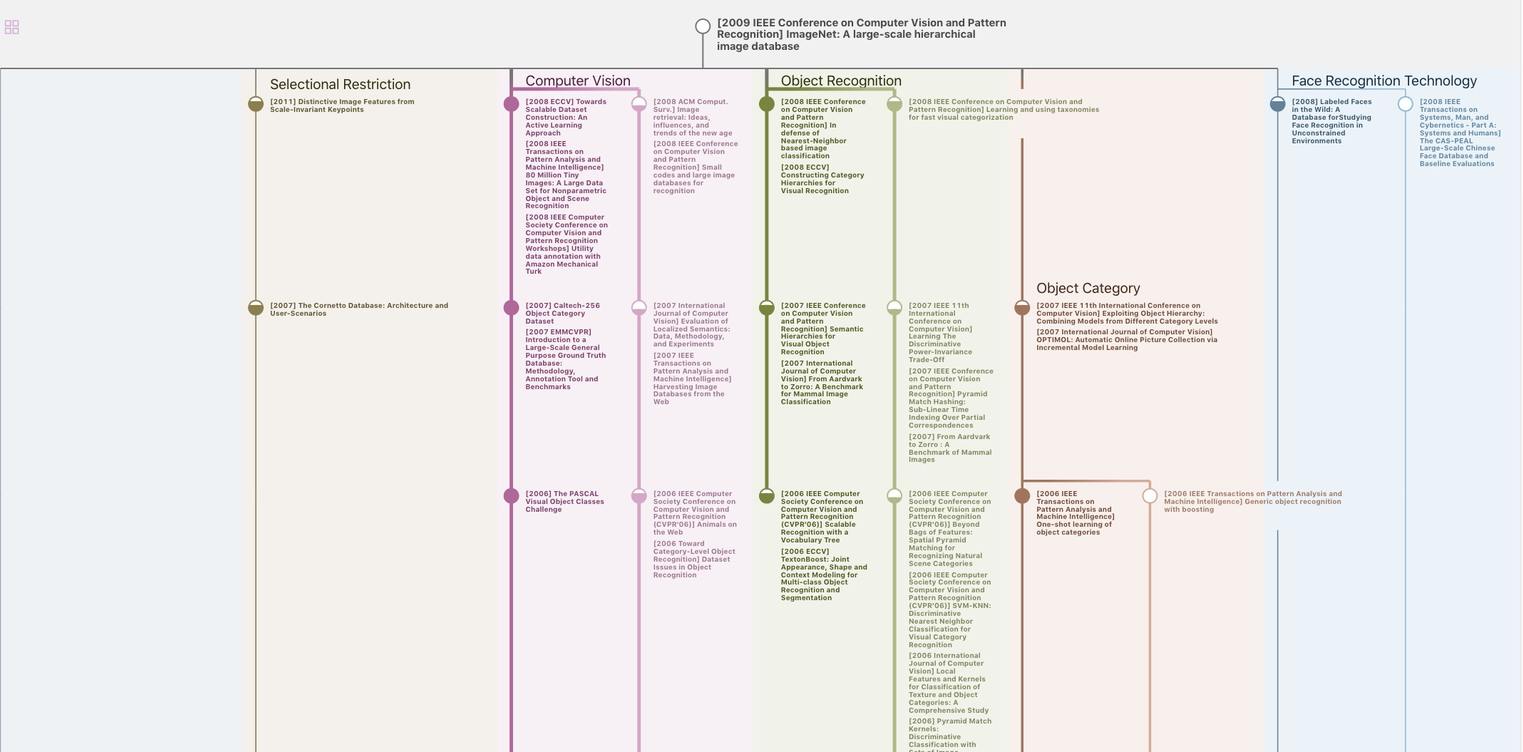
生成溯源树,研究论文发展脉络
Chat Paper
正在生成论文摘要