Insulator Defect Detection in Power Lines Based on Improved Convolution Neural Network
2023 IEEE Conference on Energy Conversion (CENCON)(2023)
摘要
In a transmission line architecture, an insulator is essential for preventing the unintended dissipation of electrical current from the conductive elements into the surrounding environment. This purpose is accomplished by effectively isolating the conductors from the supporting framework. A defect in the insulator may cause several malfunctions in the transmission line. It can range from a minor failure to catastrophic damage. Previous studies have investigated some insulator defect detection technologies using image processing methods. In modern research, classifiers are frequently used for this function in widespread detection systems. However, there are still some issues with computational effectiveness and detecting accuracy. This paper introduces an innovative approach by proposing a hybrid system based on You Only Look Once (YOLOv5) and Residual Neural Network (Resnet50) architectures. The proposed methodology achieves an excellent accuracy of 99.0 ± 0.233%. It takes 25 minutes to complete the training process for a dataset containing 1,000 photos of insulators. The suggested method can transform the inspection procedure for high-altitude insulators by smoothly merging the advantages of YOLOv5 and Resnet50 through a carefully thought-out hybrid approach.
更多查看译文
关键词
Convolution Neural Network,Insulator,unmanned aerial vehicle,Resnet50,YOLOv5
AI 理解论文
溯源树
样例
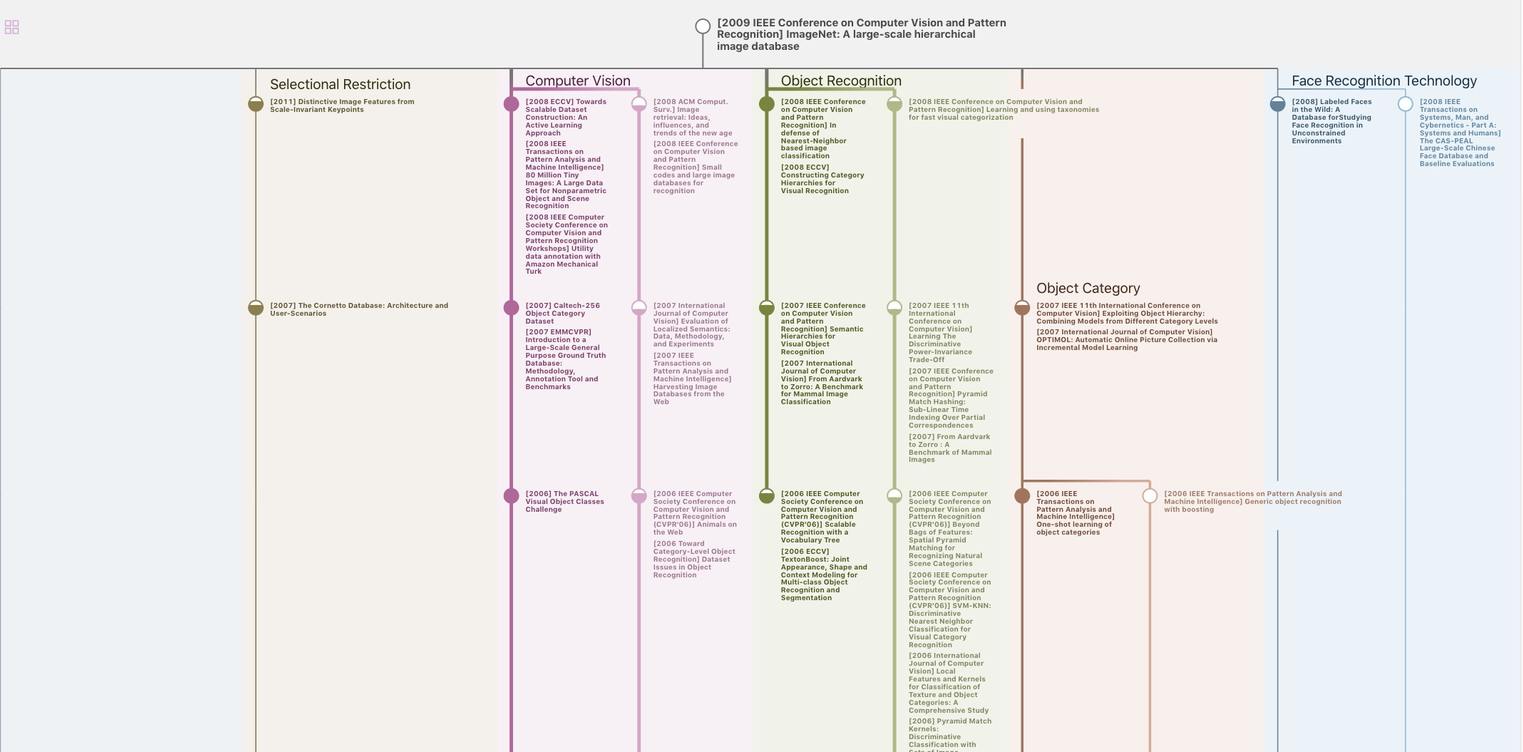
生成溯源树,研究论文发展脉络
Chat Paper
正在生成论文摘要