Tempo-CIM: A RRAM Compute-in-Memory Neuromorphic Accelerator With Area-Efficient LIF Neuron and Split-Train-Merged-Inference Algorithm for Edge AI Applications
IEEE JOURNAL ON EMERGING AND SELECTED TOPICS IN CIRCUITS AND SYSTEMS(2023)
摘要
Spiking neural network (SNN)-based compute-in-memory (CIM) accelerator provides a prospective implementation for intelligent edge devices with higher energy efficiency compared with artificial neural networks (ANN) deployed on conventional Von Neumann architectures. However, the costly circuit implementation of biological neurons and the immature training algorithm of discrete-pulse networks hinder efficient hardware implementation and high recognition rate. In this work, we present a 40nm RRAM CIM macro (Tempo-CIM) with charge-pump-based leaky-integrate-and-fire (LIF) neurons and split-train-merged-inference algorithm for efficient SNN acceleration with improved accuracy. The single-spike latency coding is employed to reduce the number of pulses in each time step. The voltage-type LIF neuron uses a charge pump structure to achieve efficient accumulation and thus reduce the requirement for large capacitance remarkably. The split-train-merged-inference algorithm is proposed to dynamically adjust the input of each neuron to alleviate the spike stall problem. The macro measures 0.084mm(2) in a 40nm process with an energy efficiency of 68.51 TOPS/W and an area efficiency of 0.1956 TOPS/mm(2) for 4b input and 8b weight.
更多查看译文
关键词
Neurons,Encoding,Computer architecture,Capacitors,Capacitance,Biological system modeling,Computational modeling,Computing in memory (CIM),resistive random-access-memory (RRAM),spiking neural network (SNN),neuromorphic accelerator
AI 理解论文
溯源树
样例
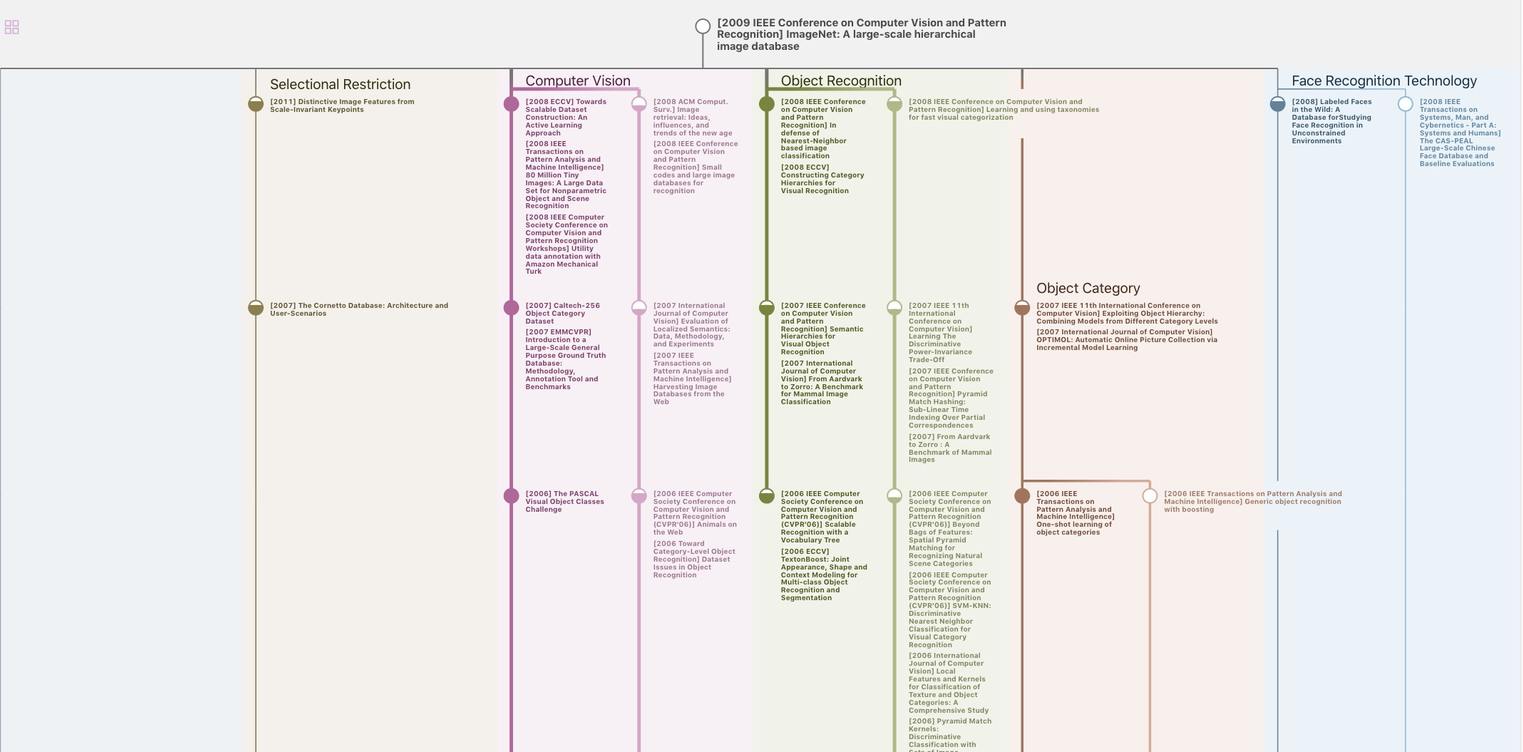
生成溯源树,研究论文发展脉络
Chat Paper
正在生成论文摘要