VOT: Revolutionizing Speaker Verification with Memory and Attention Mechanisms
CoRR(2023)
摘要
Speaker verification is essentially the process of identifying unknown
speakers within an 'open set'. Our objective is to create optimal embeddings
that condense information into concise speech-level representations, ensuring
short distances within the same speaker and long distances between different
speakers. Despite the prevalence of self-attention and convolution methods in
speaker verification, they grapple with the challenge of high computational
complexity.In order to surmount the limitations posed by the Transformer in
extracting local features and the computational intricacies of multilayer
convolution, we introduce the Memory-Attention framework. This framework
incorporates a deep feed-forward temporal memory network (DFSMN) into the
self-attention mechanism, capturing long-term context by stacking multiple
layers and enhancing the modeling of local dependencies. Building upon this, we
design a novel model called VOT, utilizing a parallel variable weight summation
structure and introducing an attention-based statistical pooling layer.To
address the hard sample mining problem, we enhance the AM-Softmax loss function
and propose a new loss function named AM-Softmax-Focal. Experimental results on
the VoxCeleb1 dataset not only showcase a significant improvement in system
performance but also surpass the majority of mainstream models, validating the
importance of local information in the speaker verification task. The code will
be available on GitHub.
更多查看译文
AI 理解论文
溯源树
样例
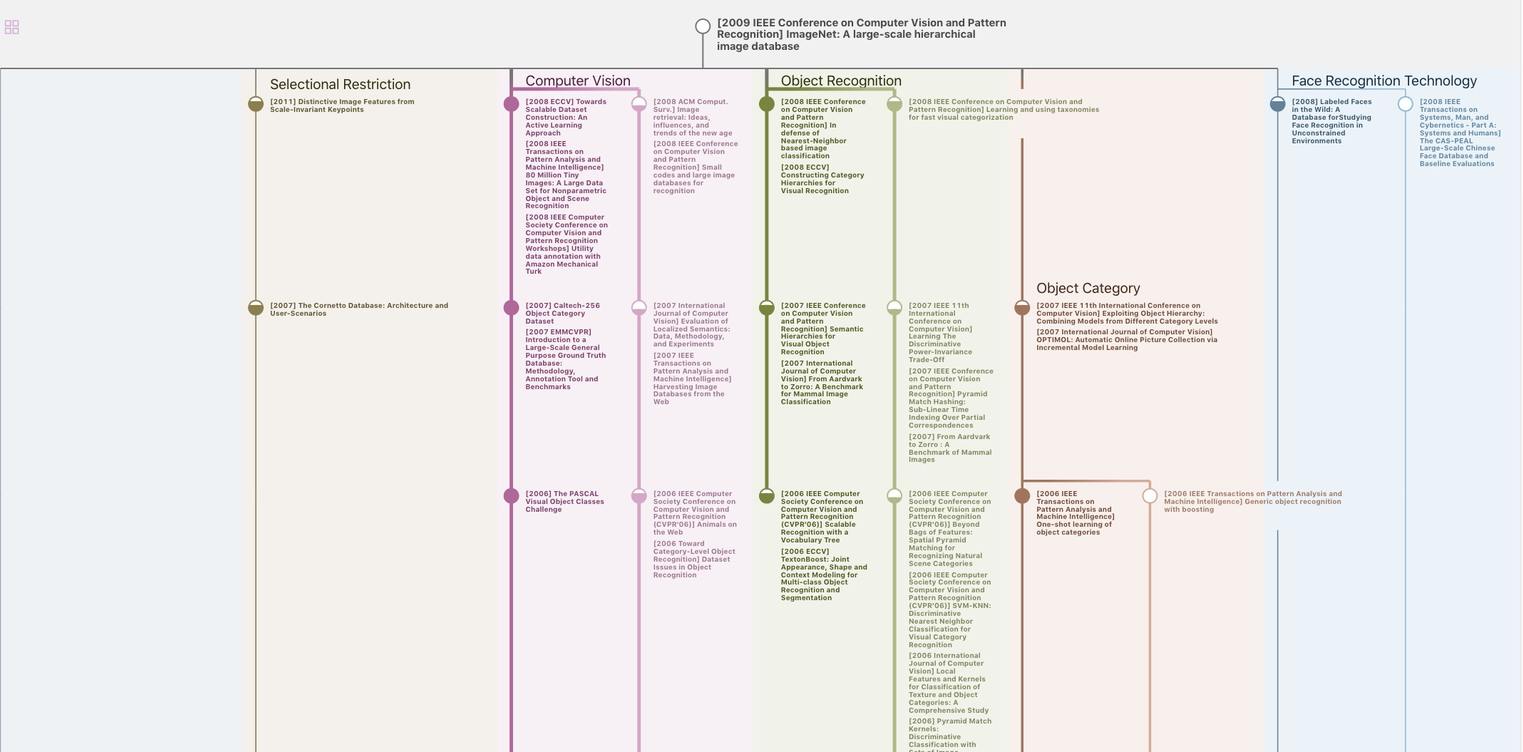
生成溯源树,研究论文发展脉络
Chat Paper
正在生成论文摘要