Weakly supervised end-to-end domain adaptation for person re-identification
COMPUTERS & ELECTRICAL ENGINEERING(2024)
摘要
Unsupervised domain adaptation (UDA) approaches for person re-identification (Re-ID) heavily rely on accurate pedestrian location, which is often unavailable in real-world scenarios. With respect to this situation, a two-step approach is commonly employed. However, this approach involves a complex process of disentangling UDA person Re-ID and pedestrian location, which consumes significant time and resources. This paper introduces a novel approach called Weakly Supervised End-to-End Domain Adaptation for Person Re-ID (WS-PreID) to address this problem. WS-PreID is designed to perform weakly supervised end-to-end person Re-ID by integrating pedestrian location and UDA person Re-ID in a unified manner. Our framework is a weakly supervised end-to-end network (WS-net) that operates solely on pedestrian bounding boxes. This approach mitigates the impact of background noise on the overall performance. Additionally, WS-PreID introduces a pseudo-label generation strategy with feature invariance (PLFI) to calculate pseudo-labels for person Re-ID training. PLFI utilizes both current features and comprehensive features to improve the precision of the assigned pseudo-labels. To evaluate the effectiveness of WS-PreID, we conducted comparative evaluations using the PRW and CUHKSYSU datasets. The experimental results validate that WS-PreID achieves an end-to-end solution and significantly improves the performance of person Re-ID.
更多查看译文
关键词
Weakly supervised,Person re-identification,End-to-end,Pseudo-labels,Pedestrian location
AI 理解论文
溯源树
样例
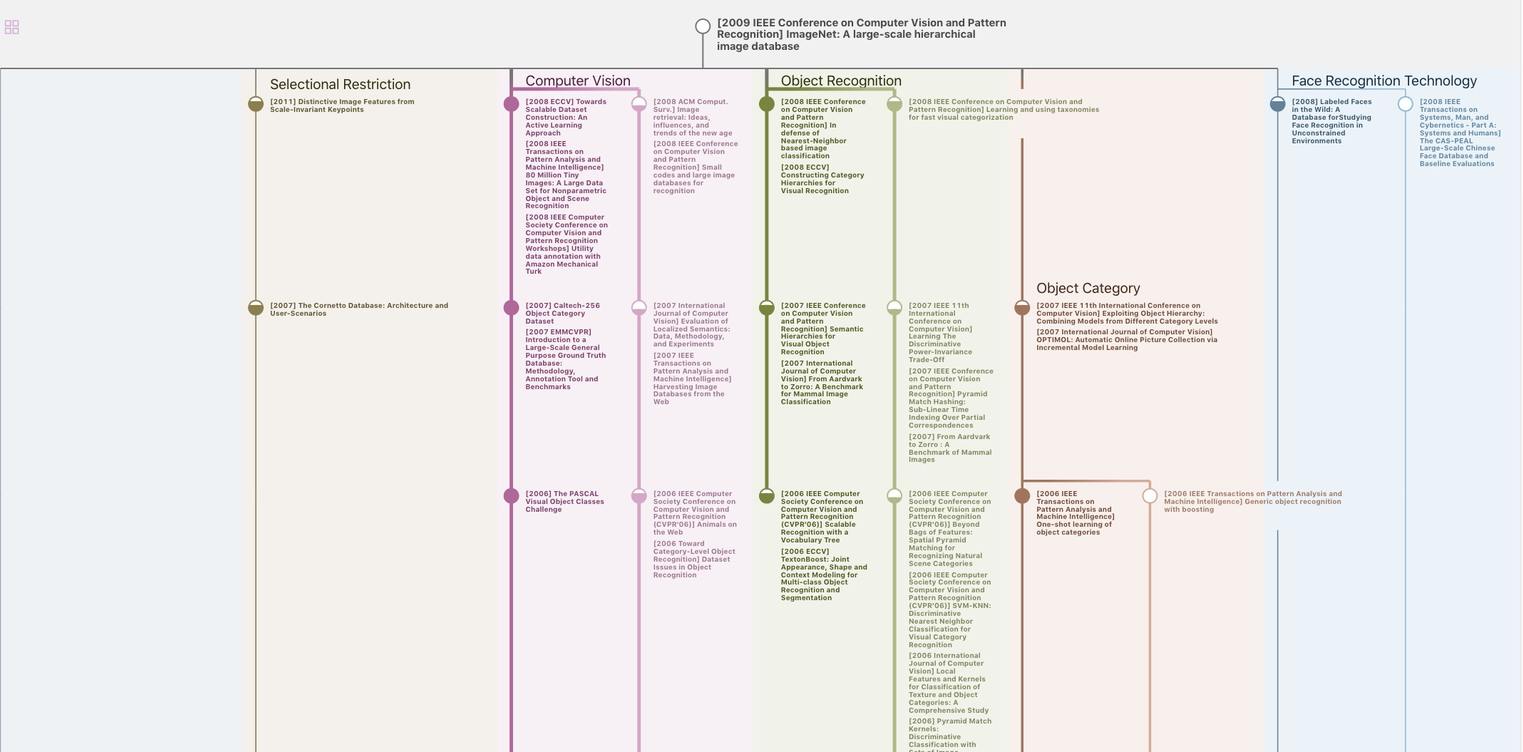
生成溯源树,研究论文发展脉络
Chat Paper
正在生成论文摘要