Leveraging Knowledge Graph Reasoning in a Multihop Question Answering System for Hot Rolling Line Fault Diagnosis
IEEE TRANSACTIONS ON INSTRUMENTATION AND MEASUREMENT(2024)
摘要
Multihop question answering (QA) over knowledge graph (KG) poses significant challenges in the context of industrial processes, due to the intricate semantics of natural language queries and the obscure relationships among triples in the KG. This article presents a multihop fault diagnosis (FD) QA framework based on KG reasoning and decision, called KG-MQA. It consists of three modules: 1) the embedding module is designed to learn representations of queried questions and encode entities and relationships within the input FD KG; 2) the KG reasoning module is proposed to learn to reason candidate answers based on query entities and context; and 3) the answer decision module is applied to obtain final answer by leveraging the attention mechanism to compute the attention scores between the input question and candidate answers. Extensive experiments have been conducted on the dataset from a hot rolling line, and our KG-MQA achieves an average accuracy of 95.7%, surpassing other state-of-the-art models. Ablation studies demonstrate the efficiency of KG-MQA in helping users obtain equipment fault-related information from massive data through the professional knowledge structure. It facilitates reasoning during data queries to efficiently provide multiple rounds of QA for equipment FD in hot rolling lines.
更多查看译文
关键词
Fault diagnosis (FD),knowledge graph (KG),knowledge graph reasoning (KGR),question answering (QA) system,reinforcement learning (RL)
AI 理解论文
溯源树
样例
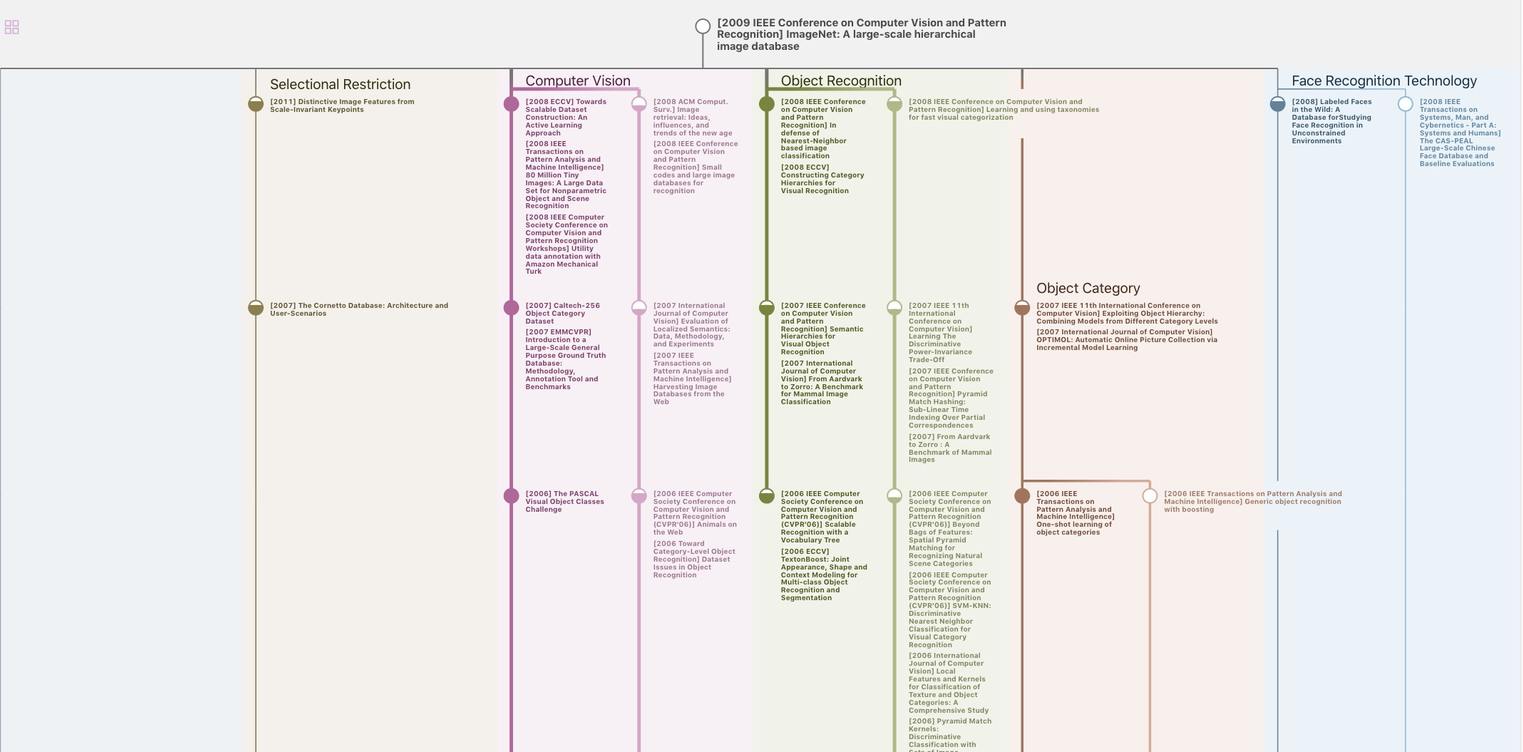
生成溯源树,研究论文发展脉络
Chat Paper
正在生成论文摘要