Development of a Novel Machine Learning-based Methodology for the Differential Diagnosis of Wide QRS Complex Arrhythmias Using Automated Analysis of 12-Lead ECG.
2023 Computing in Cardiology (CinC)(2023)
摘要
This study aimed to advance the differential diagnosis of wide QRS complex arrhythmias by developing a machine learning-based methodology. Utilizing a comprehensive dataset with over 58,253 ECG recordings from composite unified database we trained and validated a convolutional neural network (CNN) model. The model was rigorously tested for its ability to accurately classify arrhythmias, focusing on the differentiation between ventricular tachycardia (VT) and supraventricular tachycardia (SVT), among other arrhythmia types. The robust architecture of the CNN model was demonstrated by its high diagnostic accuracy across various arrhythmias, achieving more than 90% for all arrhythmias and over 97% for LBBB, RBBB, WPW, LAFB, LPFB, and nonspecific IVCD. Our results indicate that machine learning can significantly enhance the precision and efficiency of arrhythmia diagnosis in clinical settings. This research underscores the potential of AI in cardiology, suggesting a future where Al-assisted tools are seamlessly integrated into routine clinical practice to improve patient outcomes.
更多查看译文
AI 理解论文
溯源树
样例
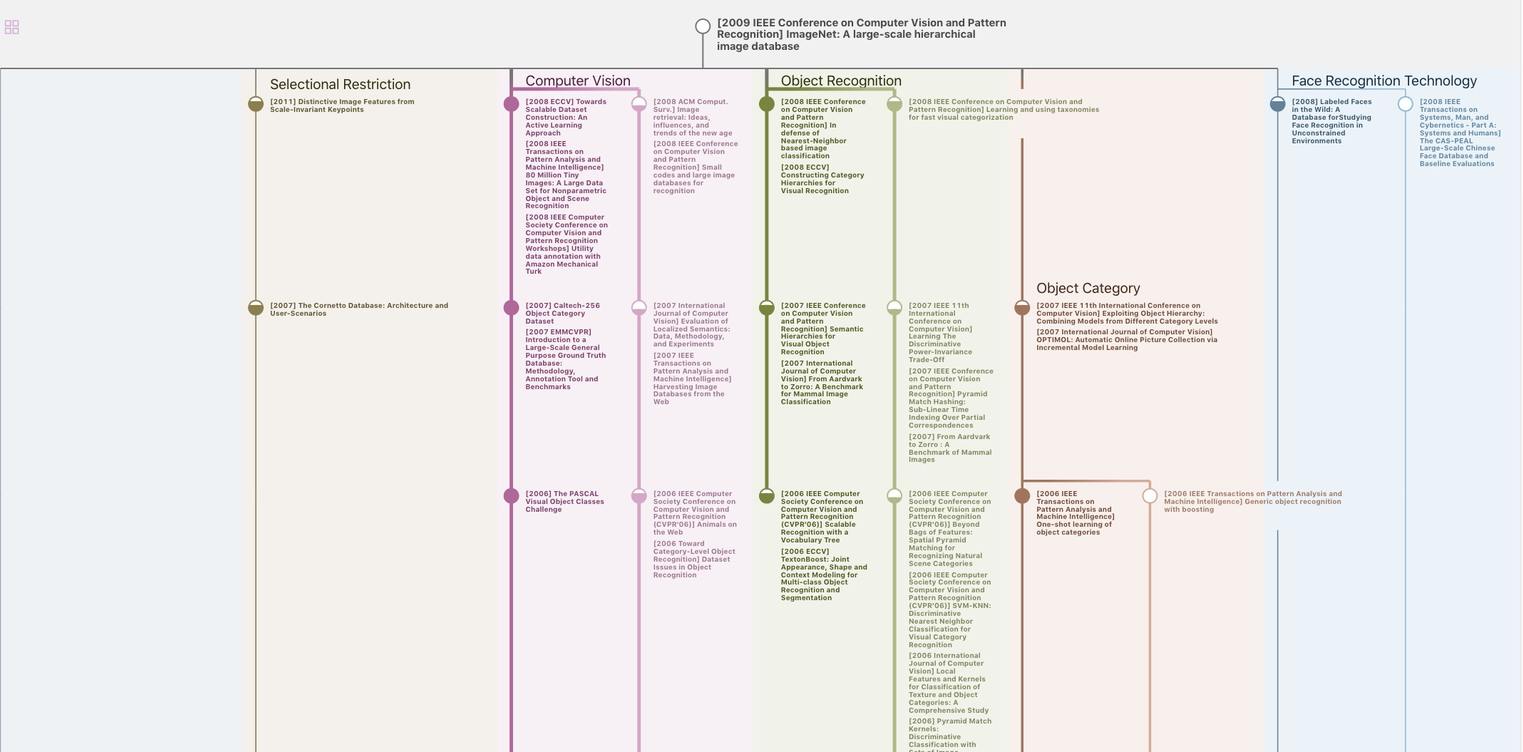
生成溯源树,研究论文发展脉络
Chat Paper
正在生成论文摘要