Vision-Language Models Performing Zero-Shot Tasks Exhibit Disparities Between Gender Groups
2023 IEEE/CVF INTERNATIONAL CONFERENCE ON COMPUTER VISION WORKSHOPS, ICCVW(2023)
摘要
We explore the extent to which zero-shot vision-language models exhibit gender bias for different vision tasks. Vision models traditionally required task-specific labels for representing concepts, as well as finetuning; zero-shot models like CLIP instead perform tasks with an open-vocabulary, meaning they do not need a fixed set of labels, by using text embeddings to represent concepts. With these capabilities in mind, we ask: Do vision-language models exhibit gender bias when performing zero-shot image classification, object detection and semantic segmentation? We evaluate different vision-language models with multiple datasets across a set of concepts and find (i) all models evaluated show distinct performance differences when identifying concepts based on the gender of the person co-occurring in the image (ii) model calibration (i.e., the relationship between accuracy and confidence) also differs distinctly by gender, even when evaluating on similar representations of concepts and (iii) these observed disparities align with existing gender biases in word embeddings from language models. These findings suggest that, while language greatly expands the capability of vision tasks, it can contribute to propagating social biases in zero-shot settings.
更多查看译文
AI 理解论文
溯源树
样例
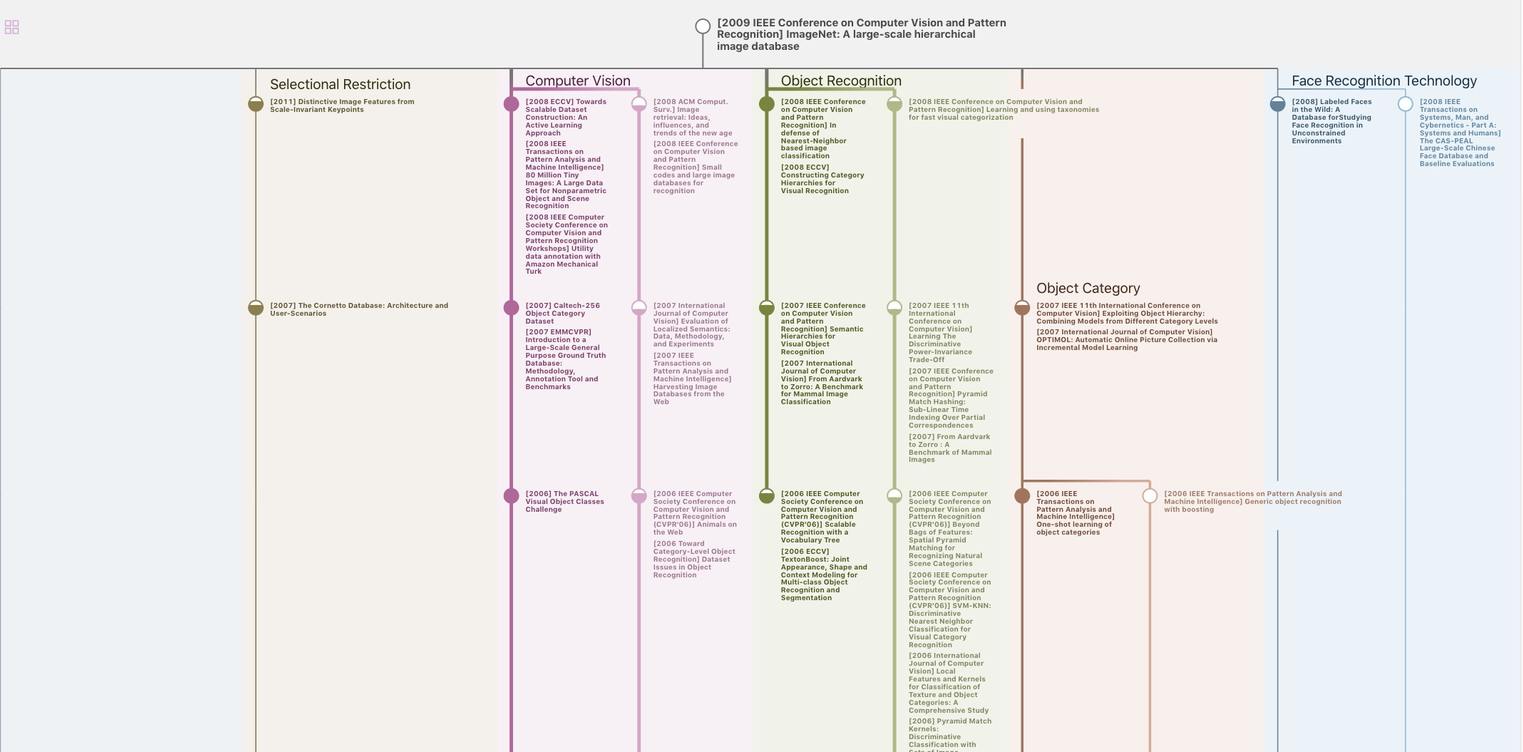
生成溯源树,研究论文发展脉络
Chat Paper
正在生成论文摘要