Quantized Generative Models for Solving Inverse Problems
2023 IEEE/CVF INTERNATIONAL CONFERENCE ON COMPUTER VISION WORKSHOPS, ICCVW(2023)
摘要
Generative priors have been shown to be highly successful in solving inverse problems. In this paper, we consider quantized generative models i.e., the generator network weights come from a learnt finite alphabet. Quantized neural networks are efficient in terms of memory and computation. They are ideally suited for deployment in a practical setting involving low-precision hardware. In this paper, we solve non-linear inverse problems using quantized generative models. We introduce a new meta-learning framework that makes use of proximal operators and jointly optimizes the quantized weights of the generative model, parameters of the sensing network, and the latent-space representation. Experimental validation is carried out using standard datasets - MNIST, CIFAR10, SVHN, and STL10. The results show that the performance of 32-bit networks can be achieved using 4-bit networks. The performance of 1-bit networks is about 0.7 to 2 dB inferior, while saving significantly (32x) on the model size.
更多查看译文
AI 理解论文
溯源树
样例
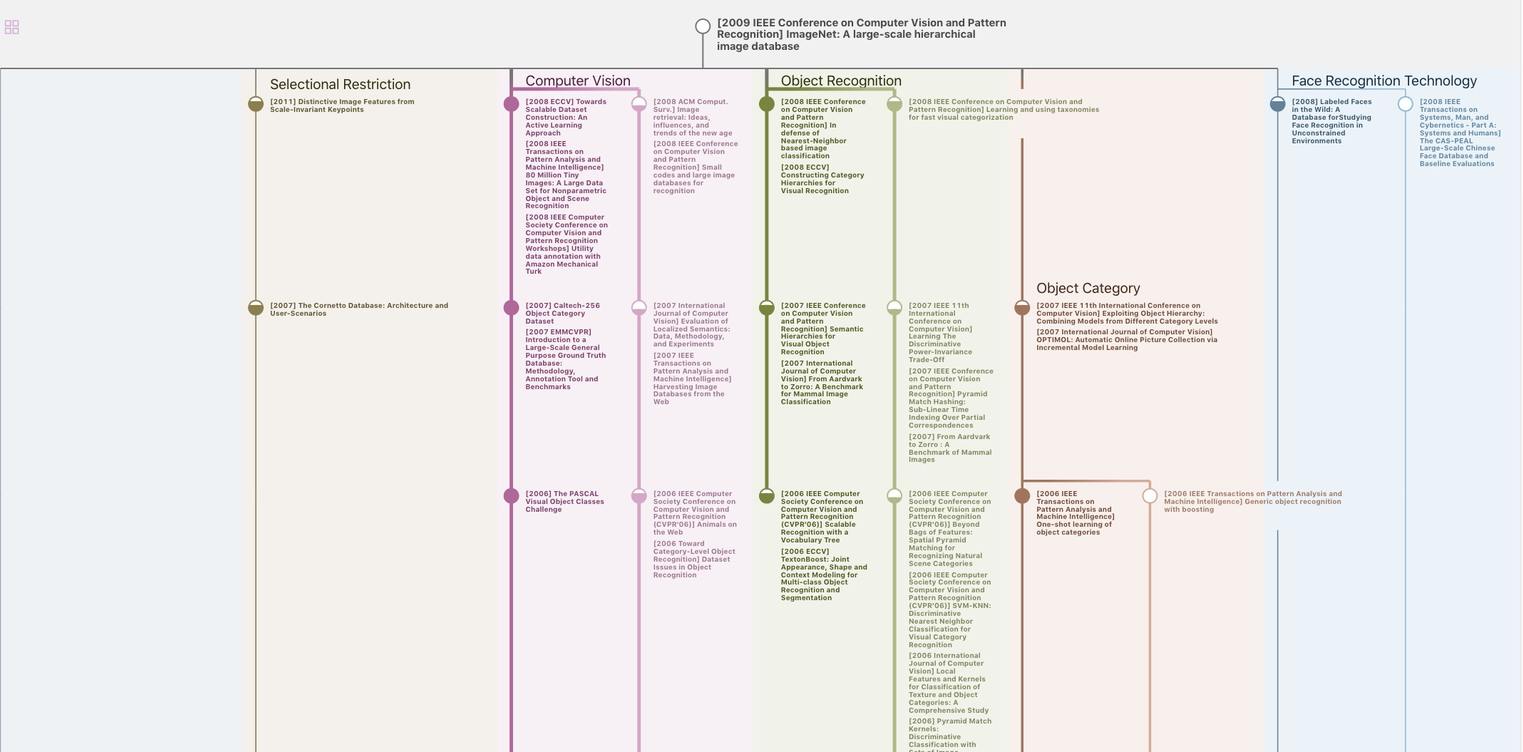
生成溯源树,研究论文发展脉络
Chat Paper
正在生成论文摘要