Enhancing Differentiable Architecture Search: A Study on Small Number of Cell Blocks in the Search Stage, and Important Branches-based Cells Selection
2023 IEEE/CVF INTERNATIONAL CONFERENCE ON COMPUTER VISION WORKSHOPS, ICCVW(2023)
摘要
In recent years, differentiable neural architecture search (DARTS) method has attracted a lot of attention. This method has been proposed to reduce the search cost incurred when using reinforcement learning and evolutionary search strategies. Although several studies have been carried out to improve its performance, most of these existing methods share some common limitations: They use a stack of five to eight cells during the search process to find only two distinct cells. The usage of several cells significantly increases the computation cost of the search process. In this paper, to reduce the search time, we propose to decouple the structure of the architecture used during the search of optimal pair of cells from the final architecture by using only one normal and one reduction cells search architecture during the search stage and the same architecture structure as DARTS during the evaluation stage. We also address the stability and performance drop trade-off by inserting additional residual connection in parallel with every normal cell block. Additionally, adding A convolution skip connection to the evaluation architecture has been shown to improve the performance. Finally, we investigated the effect of searching optimal cell's operation from highly performing branches in the internal structure of every cell. Extensive experiments showed that the proposed method significantly reduces the search cost while achieving promising results on ImageNet, CIFAR-10, and CIFAR-100 compared to existing state-of-the-art methods on DARTS search space.
更多查看译文
AI 理解论文
溯源树
样例
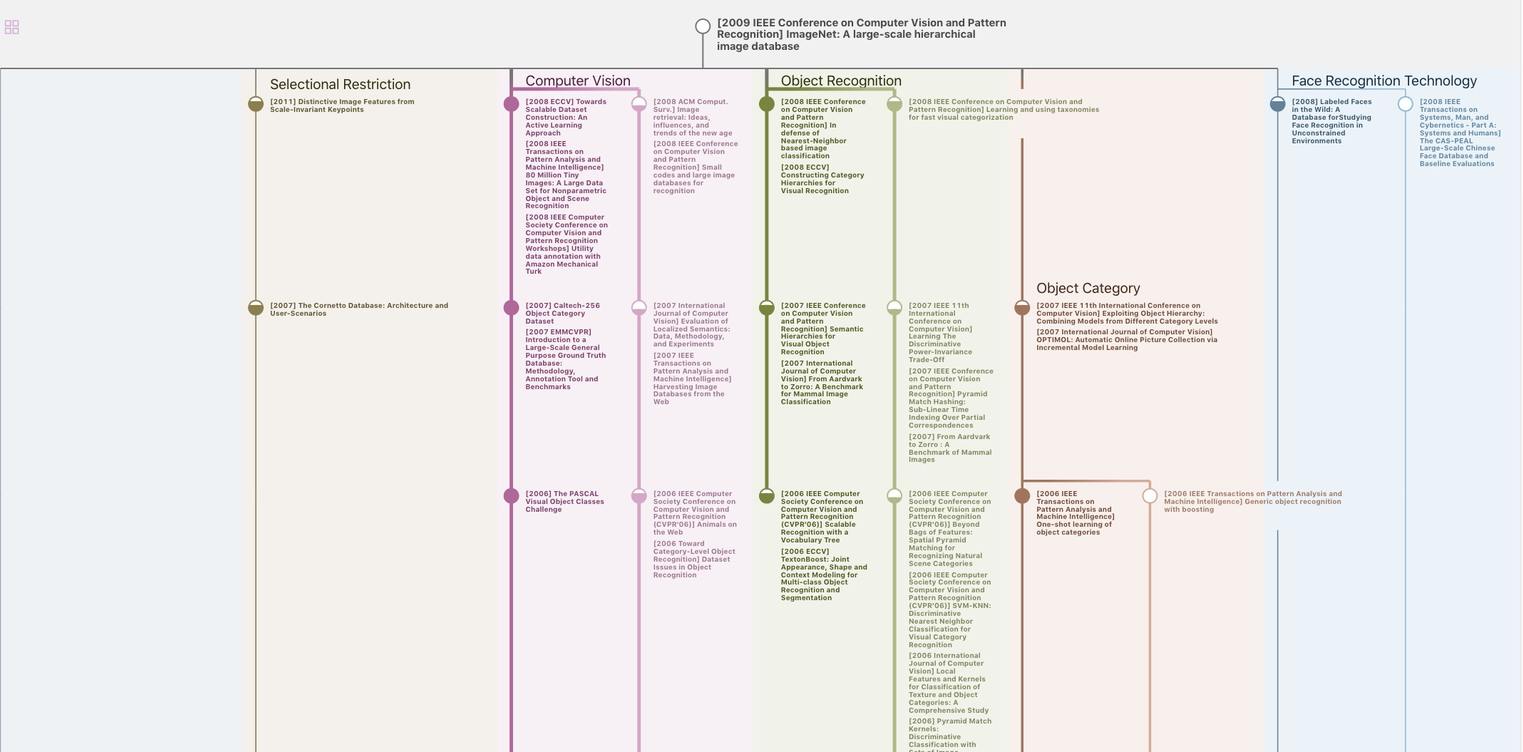
生成溯源树,研究论文发展脉络
Chat Paper
正在生成论文摘要