Shapley Deep Learning: A Consensus for General-Purpose Vision Systems
2023 IEEE/CVF INTERNATIONAL CONFERENCE ON COMPUTER VISION WORKSHOPS, ICCVW(2023)
摘要
Shapely Deep Learning (SDL) targets a new foundation for the design of general-purpose vision systems, by establishing a consensus method that facilitates self-adaptation and flexibility to deal with new computer vision tasks. Today, machine learning did not yet reach the flexible, general-purpose intelligence that biological vision has in mimicking visual descriptions and learning in general-purpose vision algorithms. Currently, each model is built using the domain knowledge of the application in question. Data scientists must consequently be well-versed in the relevant subject. This paper presents SDL as a consensus method for general-purpose intelligence without the help of a domain expert as the trained model has been developed utilizing a general deep learning approach that investigates the contribution of each model in the training process. First, several deep learning models have been trained for each image. The shapley value is then determined to compute the contribution of each subset of models in the training. The model selection is finally performed based on the shapley value and the joint model cost. Optimization of the shapley computation is also carried out by investigating the banzhaf function. We present the evaluation of the generality of SDL using the computer vision benchmarks: MNIST for Shapley value performance, ImageNet for image classification, and COCO for object detection. The results reveal the effectiveness of SDL in terms of accuracy and competitiveness of inference runtime. Concretely, SDL achieved 10%, and 8% over MViTv2 for classification, and object detection tasks, respectively.
更多查看译文
AI 理解论文
溯源树
样例
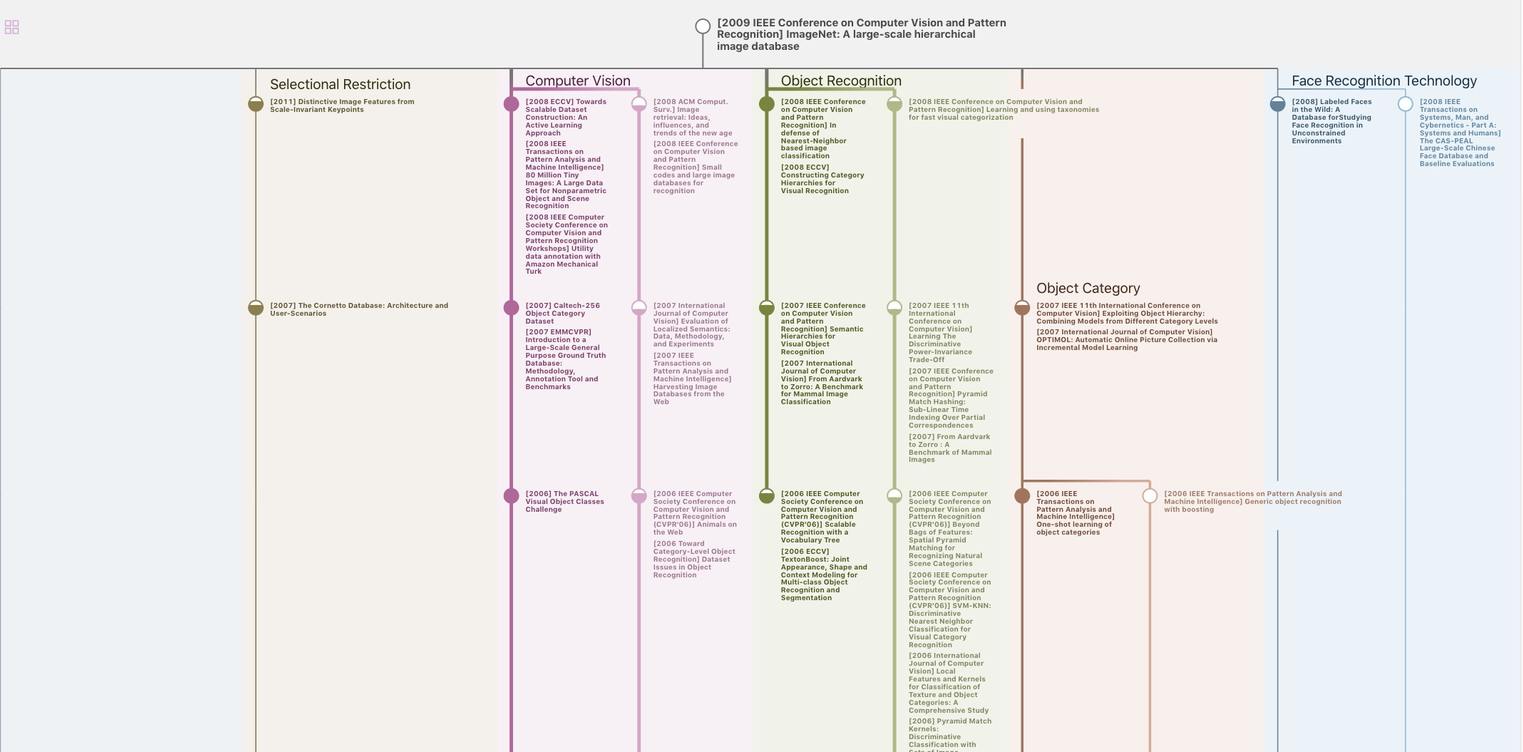
生成溯源树,研究论文发展脉络
Chat Paper
正在生成论文摘要