Prediction of Functional Recovery Post-Cardiac Arrest Using an Ensemble of Extreme Gradient-Boosted Trees.
2023 Computing in Cardiology (CinC)(2023)
摘要
Predicting the outcome of critically ill patients after cardiac arrest is a substantial clinical challenge. As part of the 2023 George B. Moody PhysioNet Challenge, we used an ensemble of extreme gradient boosted (XGB) trees, trained on electroencephalogram (EEG) data across three distinct levels of analysis to predict neurological outcomes. To this end, we preprocessed raw EEG recordings via filtering and motion correction and extracted several features, including connectivity, power spectral density (PSD), and coherence. The first step of model training and optimization occurred at the Recording level, where we trained XGB trees on each feature set (e.g., PSD) derived from every EEG recording. These models predicted the neurologic outcome for each recording. At the Patient level, we computed the median of each feature set for each patient, which we used to make patient-specific predictions. Finally, the Challenge level merged and optimized predictions from the previous levels, thus synthesizing patient and recording specific predictions. Our approach produced successful results on the test set, with Challenge Scores of 0.371 (12 hrs), 0.480 (24 hrs), 0.569 (48 hrs), and 0.678 (72 hrs), resulting in a final ranking of 9/36 (team: WesternUni). The approach showed particular promise at early timepoints, placing 2
nd
when only using EEG available 12-hours post-ictus.
更多查看译文
AI 理解论文
溯源树
样例
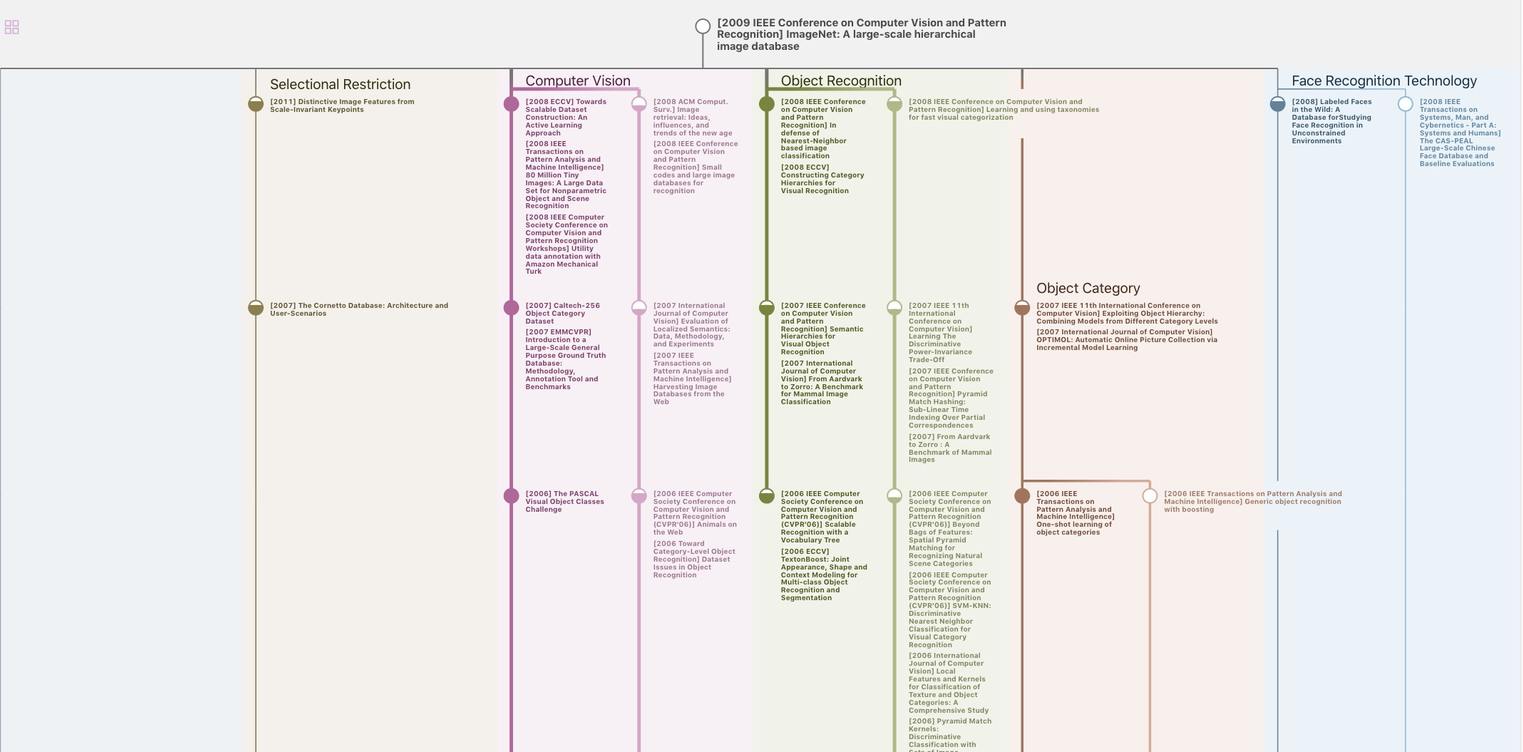
生成溯源树,研究论文发展脉络
Chat Paper
正在生成论文摘要