MADRL-Based Joint Edge Caching and Bitrate Selection for Multicategory 360 Video Streaming
IEEE INTERNET OF THINGS JOURNAL(2024)
摘要
360 degrees video streaming has gained increasing attraction in the current popular virtual reality, AR, and MR applications, which can provide users with an immersive experience. In tile-based 360 degrees video streaming, edge caching and bitrate selection strategies are jointly designed to improve users' Quality of Experience (QoE), which incorporates video quality and rebuffer. However, the existing QoE-driven approaches use a unified QoE function to guide the decisions of edge caching and bitrate selection, which neglect the impact of video quality and rebuffer on different categories of 360 degrees videos, thus failing to provide high-average QoE for users. In this article, we propose a multiagent deep-reinforcement-learning-based joint edge caching and bitrate selection strategy for multicategory 360 degrees video streaming to improve users' average QoE. The key idea is to employ different edge caching and bitrate selection strategies for different video categories to enable fine-grained performance optimization. Based on multicategory 360 degrees video streaming, we first model a joint edge caching and bitrate selection problem as a multiagent cooperative Markov decision process with the goal of maximizing users' average QoE. Next, an Field-of-View-aware multiagent soft actor-critic (FA-MASAC) algorithm is designed to help agents collaboratively learn optimal edge caching and bitrate selection decisions in a distributed way, in which each video category is treated as an agent. Finally, experimental results on real-world data sets show that our proposed strategy can greatly benefit users' average QoE compared to existing strategies.
更多查看译文
关键词
Streaming media,Bit rate,Quality of experience,Video recording,Quality assessment,Games,Bandwidth,Bitrate selection,edge caching,multiagent deep reinforcement learning (MADRL),multicategory 360 degrees video streaming,Quality of Experience (QoE)
AI 理解论文
溯源树
样例
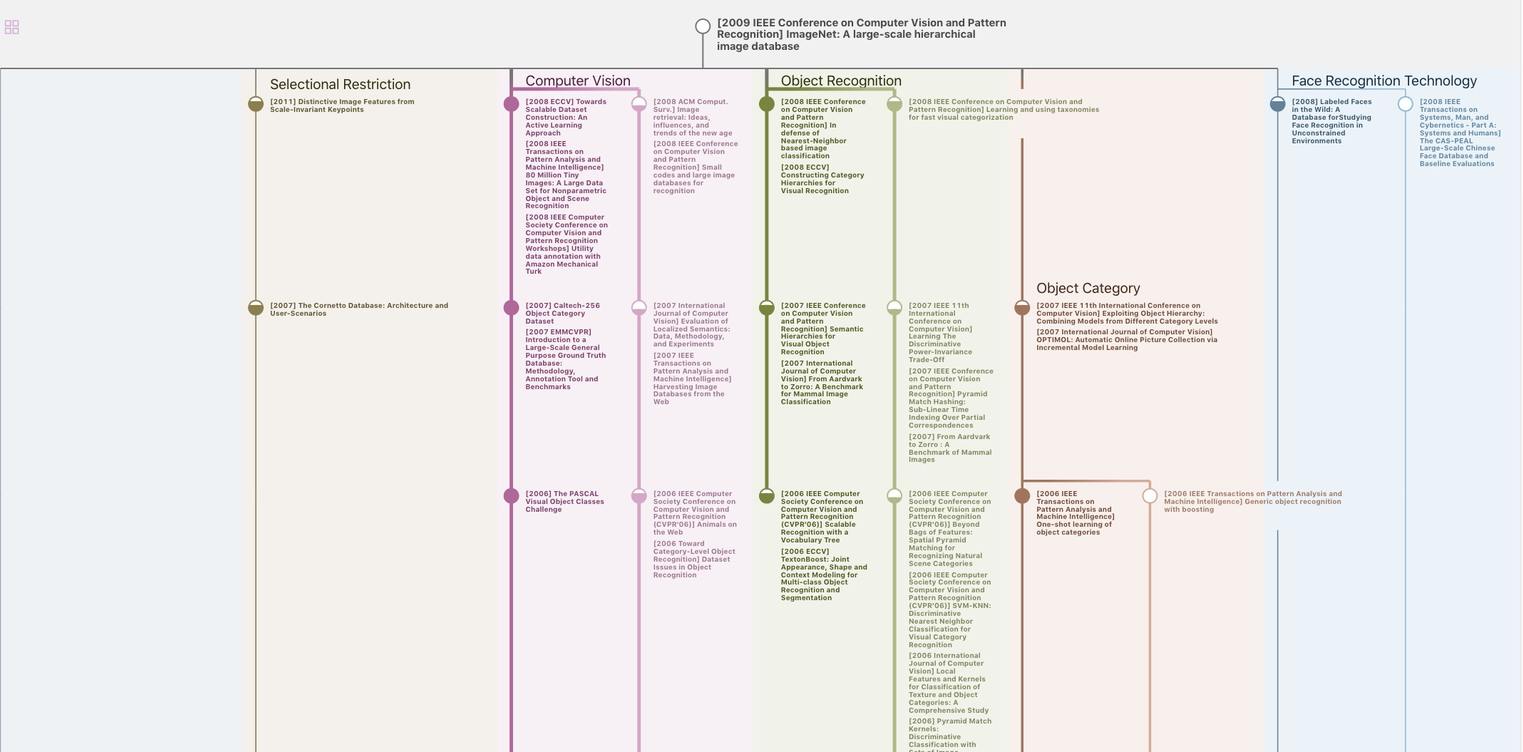
生成溯源树,研究论文发展脉络
Chat Paper
正在生成论文摘要