En-DeepONet: An enrichment approach for enhancing the expressivity of neural operators with applications to seismology
COMPUTER METHODS IN APPLIED MECHANICS AND ENGINEERING(2024)
摘要
The Eikonal equation plays a central role in seismic wave propagation and hypocenter localization, a crucial aspect of efficient earthquake early warning systems. Despite recent progress, real-time earthquake localization remains challenging due to the need to learn a generalizable Eikonal operator. We introduce a novel deep learning architecture, Enriched-DeepONet (En-DeepONet), addressing the limitations of current operator learning models in dealing with moving-solution operators. Leveraging addition and subtraction operations and a novel 'root' network, En-DeepONet is particularly suitable for learning such operators and achieves up to four orders of magnitude improved accuracy without increased training cost. We demonstrate the effectiveness of En-DeepONet in earthquake localization under variable velocity and arrival time conditions. Our results indicate that En-DeepONet paves the way for real-time hypocenter localization for velocity models of practical interest. The proposed method represents a significant advancement in operator learning that is applicable to a gamut of scientific problems, including those in seismology, fracture mechanics, and phase-field problems.
更多查看译文
关键词
Earthquake localization,Neural operators,DeepONet,Machine learning
AI 理解论文
溯源树
样例
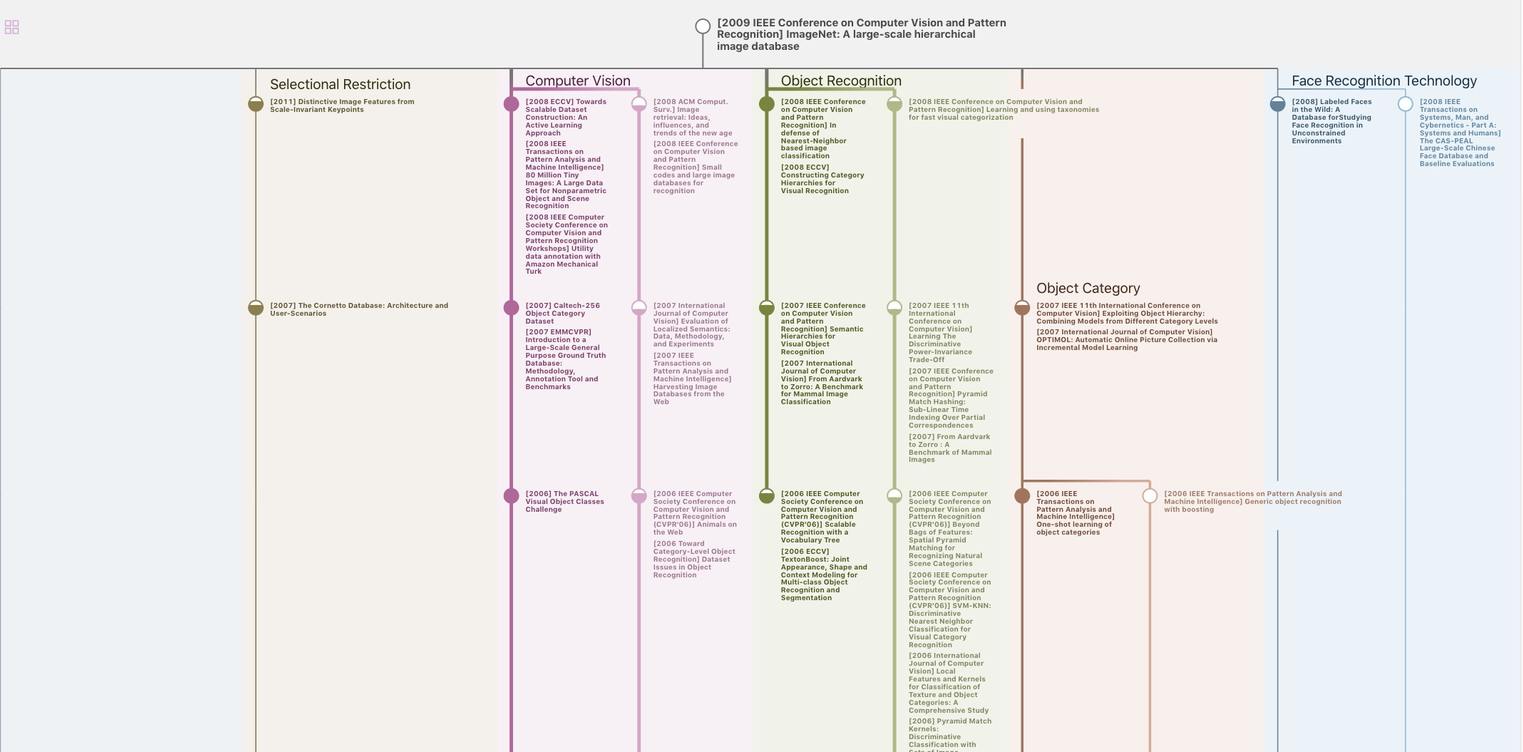
生成溯源树,研究论文发展脉络
Chat Paper
正在生成论文摘要