Discovering modular solutions that generalize compositionally
CoRR(2023)
摘要
Many complex tasks and environments can be decomposed into simpler, independent parts. Discovering such underlying compositional structure has the potential to expedite adaptation and enable compositional generalization. Despite progress, our most powerful systems struggle to compose flexibly. While most of these systems are monolithic, modularity promises to allow capturing the compositional nature of many tasks. However, it is unclear under which circumstances modular systems discover this hidden compositional structure. To shed light on this question, we study a teacher-student setting with a modular teacher where we have full control over the composition of ground truth modules. This allows us to relate the problem of compositional generalization to that of identification of the underlying modules. We show theoretically that identification up to linear transformation purely from demonstrations is possible in hypernetworks without having to learn an exponential number of module combinations. While our theory assumes the infinite data limit, in an extensive empirical study we demonstrate how meta-learning from finite data can discover modular solutions that generalize compositionally in modular but not monolithic architectures. We further show that our insights translate outside the teacher-student setting and demonstrate that in tasks with compositional preferences and tasks with compositional goals hypernetworks can discover modular policies that compositionally generalize.
更多查看译文
关键词
modularity,compositionality,compositional generalization,teacher-student,meta-learning,hypernetworks
AI 理解论文
溯源树
样例
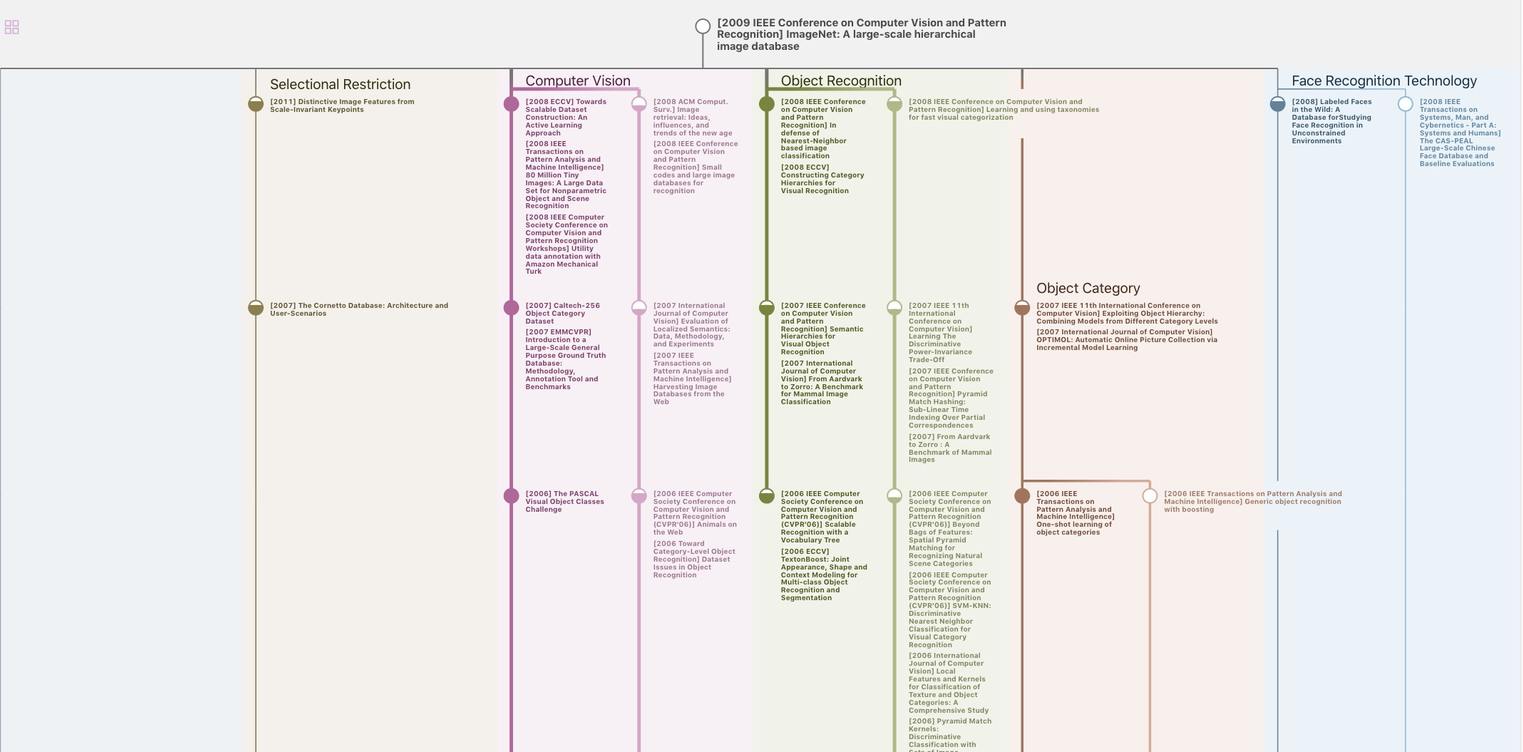
生成溯源树,研究论文发展脉络
Chat Paper
正在生成论文摘要