Paralinguistics-Enhanced Large Language Modeling of Spoken Dialogue
CoRR(2023)
摘要
Large Language Models (LLMs) have demonstrated superior abilities in tasks such as chatting, reasoning, and question-answering. However, standard LLMs may ignore crucial paralinguistic information, such as sentiment, emotion, and speaking style, which are essential for achieving natural, human-like spoken conversation, especially when such information is conveyed by acoustic cues. We therefore propose Paralinguistics-enhanced Generative Pretrained Transformer (ParalinGPT), an LLM utilizes text and speech modality to better model the linguistic content and paralinguistic attribute of spoken response. The model takes the conversational context of text, speech embeddings, and paralinguistic attributes as input prompts within a serialized multitasking multi-modal framework. Specifically, our framework serializes tasks in the order of current paralinguistic attribute prediction, response paralinguistic attribute prediction, and response text generation with autoregressive conditioning. We utilize the Switchboard-1 corpus, including its sentiment labels to be the paralinguistic attribute, as our spoken dialogue dataset. Experimental results indicate the proposed serialized multitasking method outperforms typical sequence classification techniques on current and response sentiment classification. Furthermore, leveraging conversational context and speech embeddings significantly improves both response text generation and sentiment prediction. Our proposed framework achieves relative improvements of 6.7 12.0 response text BLEU score, respectively.
更多查看译文
关键词
Spoken dialogue modeling,large language models,paralinguistics,speech sentiment analysis
AI 理解论文
溯源树
样例
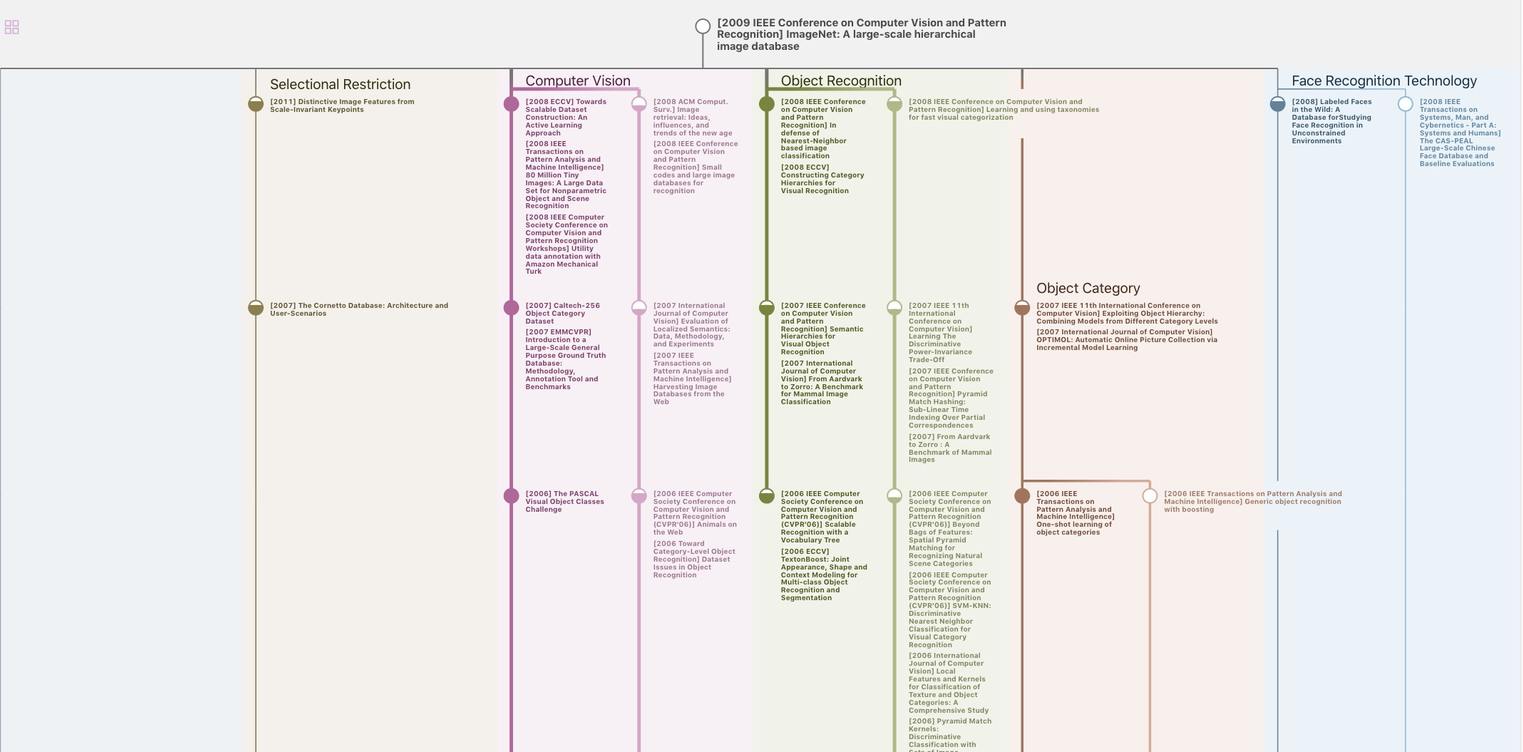
生成溯源树,研究论文发展脉络
Chat Paper
正在生成论文摘要