MotifPiece: A Data-Driven Approach for Effective Motif Extraction and Molecular Representation Learning
CoRR(2023)
摘要
Motif extraction is an important task in motif based molecular representation learning. Previously, machine learning approaches employing either rule-based or string-based techniques to extract motifs. Rule-based approaches may extract motifs that aren't frequent or prevalent within the molecular data, which can lead to an incomplete understanding of essential structural patterns in molecules. String-based methods often lose the topological information inherent in molecules. This can be a significant drawback because topology plays a vital role in defining the spatial arrangement and connectivity of atoms within a molecule, which can be critical for understanding its properties and behavior. In this paper, we develop a data-driven motif extraction technique known as MotifPiece, which employs statistical measures to define motifs. To comprehensively evaluate the effectiveness of MotifPiece, we introduce a heterogeneous learning module. Our model shows an improvement compared to previously reported models. Additionally, we demonstrate that its performance can be further enhanced in two ways: first, by incorporating more data to aid in generating a richer motif vocabulary, and second, by merging multiple datasets that share enough motifs, allowing for cross-dataset learning.
更多查看译文
AI 理解论文
溯源树
样例
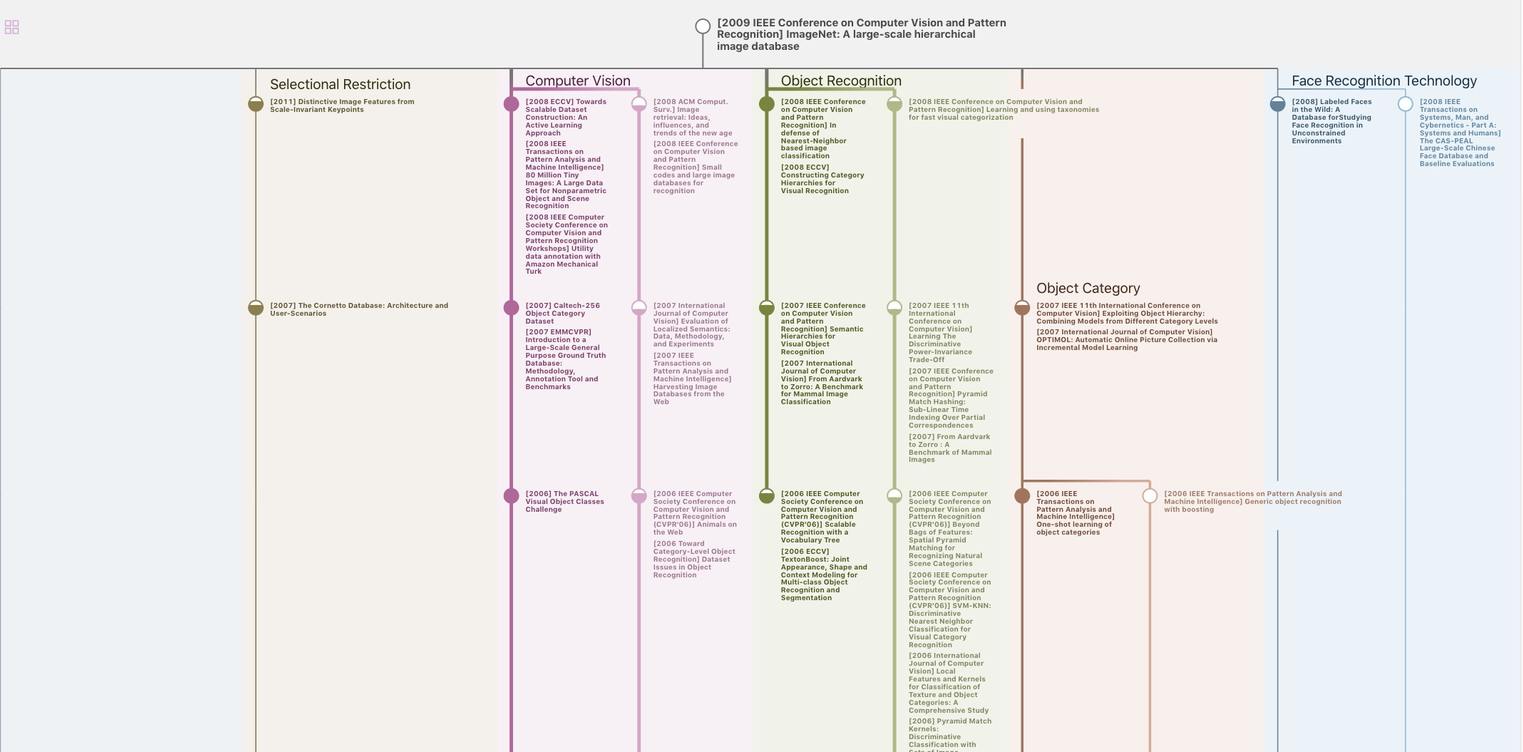
生成溯源树,研究论文发展脉络
Chat Paper
正在生成论文摘要