Cooperative Agent System for Quantifying Link Robustness in Tactical Networks
MILCOM 2023 - 2023 IEEE MILITARY COMMUNICATIONS CONFERENCE(2023)
摘要
This paper presents a cooperative agent system employing Reinforcement Learning (RL) to quantify path robustness in tactical networks. We implement an environment builder agent generating network graphs with dynamic topologies, constrained by tactical network characteristics and conditions derived from experimental results using real military radios (VHF). Subsequently, the RL agent interacts with this environment, learning to quantify the robustness of feasible transmission paths by solving an optimal control problem. Furthermore, we propose an adversary to evaluate the RL agent's robustness against adversarial perturbations. Experiments across a large set of network graphs reveal that policy optimization algorithms (PPO and TRPO) outperform others (ARS, DQN and A2C), demonstrating high rewards (similar to 105) in the absence of adversaries. However, when assessing performance in scenarios with adversarial noise injection, experimental results indicate that iterative, and momentum boosting attack techniques can substantially degrade the RL model's performance by up to 300% lower rewards.
更多查看译文
关键词
Tactical Networks,Reinforcement Learning,Network Robustness,Adversarial Attacks
AI 理解论文
溯源树
样例
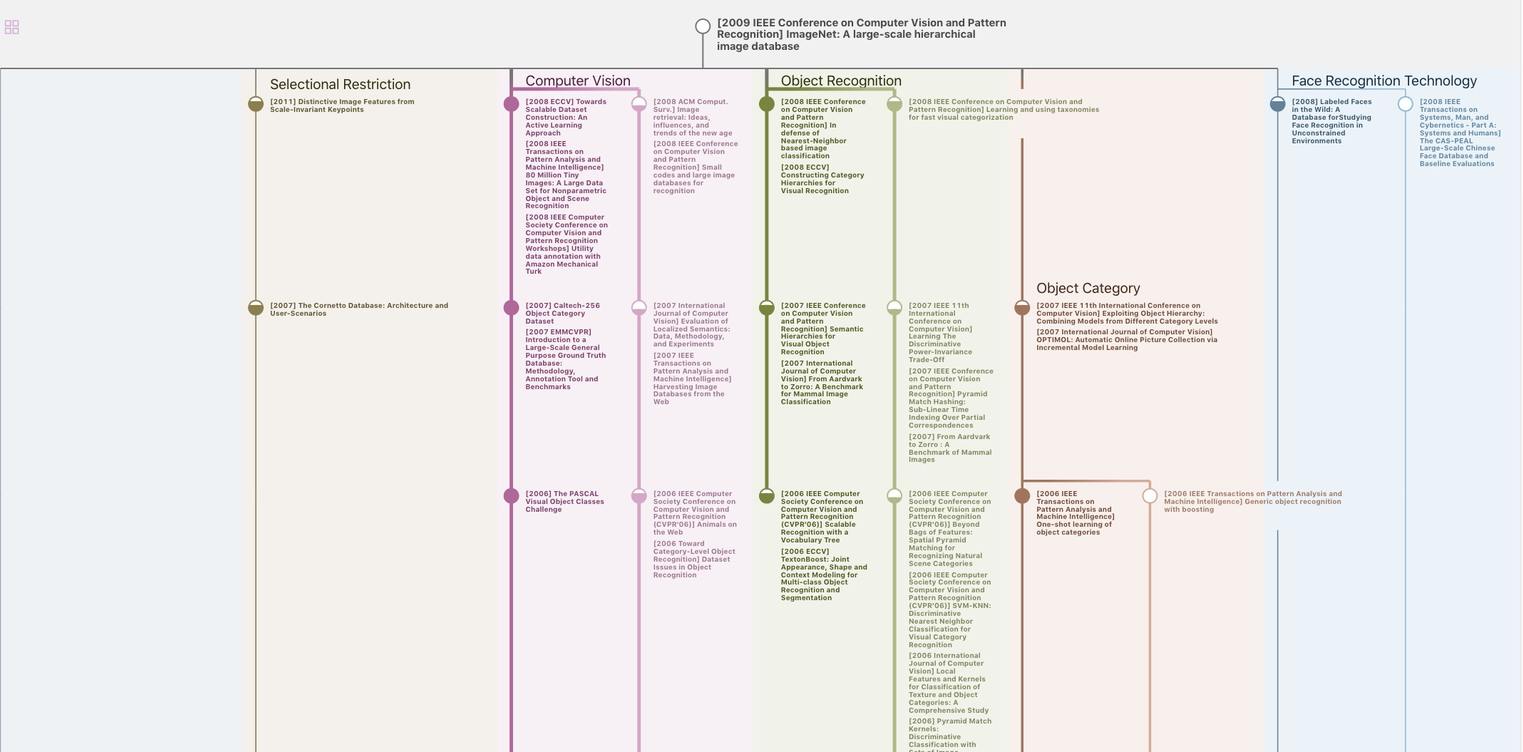
生成溯源树,研究论文发展脉络
Chat Paper
正在生成论文摘要