Towards the FAIR Asset Tracking across Models, Datasets, and Performance Evaluation Scenarios
2023 IEEE HIGH PERFORMANCE EXTREME COMPUTING CONFERENCE, HPEC(2023)
摘要
In order to ensure the reproducibility and give full account of the results obtained from scientific simulations assisted by ML/AI models, a new set of methodological innovations have to take place, that account for provenance, deployment, usage, updates, and archiving of variety of digital assets. We present a design and implementation of a methodology, that not only addresses these very aspects of modern computational science but is also a major step towards practically achieving this lofty goal for a variety of established models and their datasets, that are of particular importance to the progress of science simulations utilizing ML/AI models. We also show experimental results of applying our approach to a specific evaluation scenario and show how it maintains the performance efficiency, delivers accurate training results, and captures sufficiently rich context of the runtime behavior to inform both the particular domain science and machine learning communities.
更多查看译文
AI 理解论文
溯源树
样例
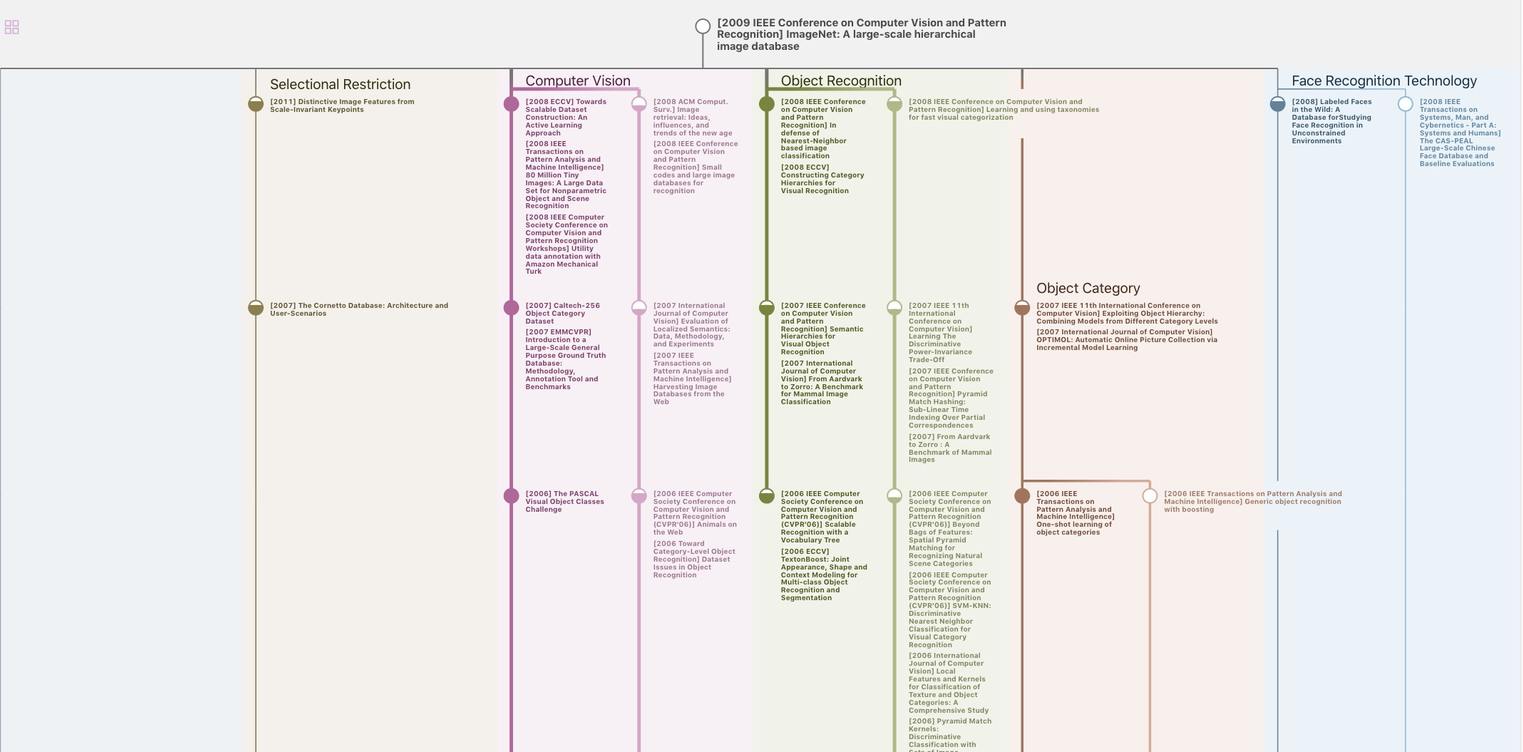
生成溯源树,研究论文发展脉络
Chat Paper
正在生成论文摘要