Fully Decentralized Client Selection for Energy-Efficient Federated Learning.
2023 IEEE Intl Conf on Dependable, Autonomic and Secure Computing, Intl Conf on Pervasive Intelligence and Computing, Intl Conf on Cloud and Big Data Computing, Intl Conf on Cyber Science and Technology Congress (DASC/PiCom/CBDCom/CyberSciTech)(2023)
摘要
Federated learning is the most famous algorithm for training machine learning models over distributed datasets without sharing the local data with a central server. However, in many real-life settings, not all clients are equally beneficial for training the model. The selection of the optimal subset of participating clients is critical for ensuring high performance and low energy consumption. This paper formulates the client selection problem as a multi-agent optimization task with the goal of finding a trade-off between performance and energy consumption. In particular, we propose a fully decentralized client selection policy based on non-stationary multi-armed bandits where clients autonomously decide to participate or not in the training process without relying on the central server. The proposed solution outperforms the random client selection policy reducing up to 12% both the number of rounds required to achieve the target accuracy and energy consumption.
更多查看译文
关键词
federated learning,data analysis,multi-agent systems,multi-armed bandit,energy efficiency,edge computing
AI 理解论文
溯源树
样例
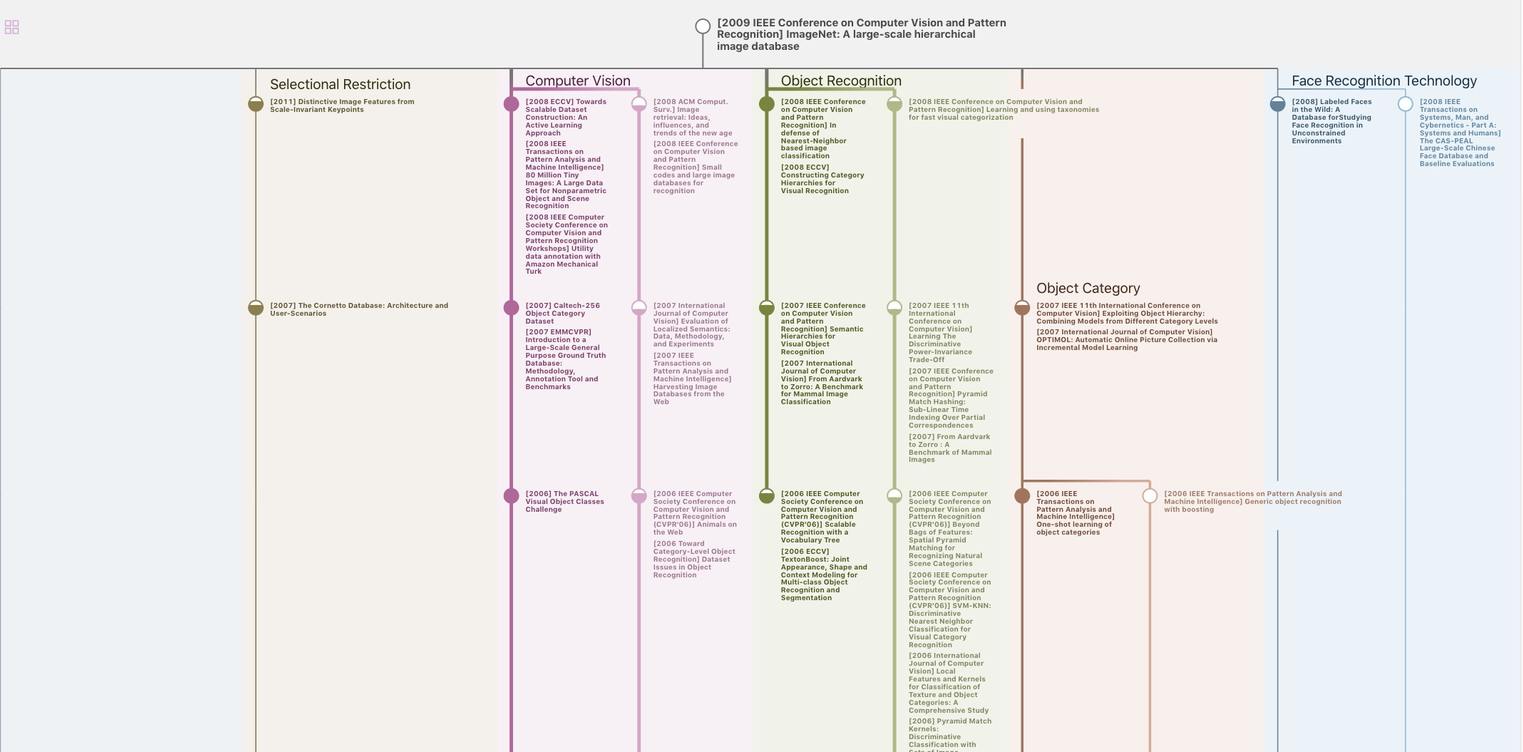
生成溯源树,研究论文发展脉络
Chat Paper
正在生成论文摘要