Traffic Flow Prediction Based on Dynamic Graph Feature Learning.
2023 IEEE Intl Conf on Dependable, Autonomic and Secure Computing, Intl Conf on Pervasive Intelligence and Computing, Intl Conf on Cloud and Big Data Computing, Intl Conf on Cyber Science and Technology Congress (DASC/PiCom/CBDCom/CyberSciTech)(2023)
摘要
Accurate traffic flow prediction can help traffic platform managers to dispatch resources, so as to better meet people's travel needs, and reduce traffic jams and accidents. This paper proposes a traffic flow prediction model so called DGNN based on dynamic graph feature learning. It consists of four parts: spatio-temporal embedding module, temporal convolution module, dynamic graph feature learning module and prediction module. The key idea of DGNN is to construct dynamic adjacency matrices through real-time traffic state information, then pass the matrices and the input sequence through a dynamic graph convolution network to extract dynamic spatial features. Experiments are established on three real-world public datasets with different data scale and number of sensor nodes, and compared with several baseline methods. The results show that DGNN outperforms baseline methods in both short term and long term traffic prediction tasks.
更多查看译文
关键词
Traffic Flow Prediction,Spatio-Temporal Correlation,Graph Convolution Network,Dynamic Graph Neural Network
AI 理解论文
溯源树
样例
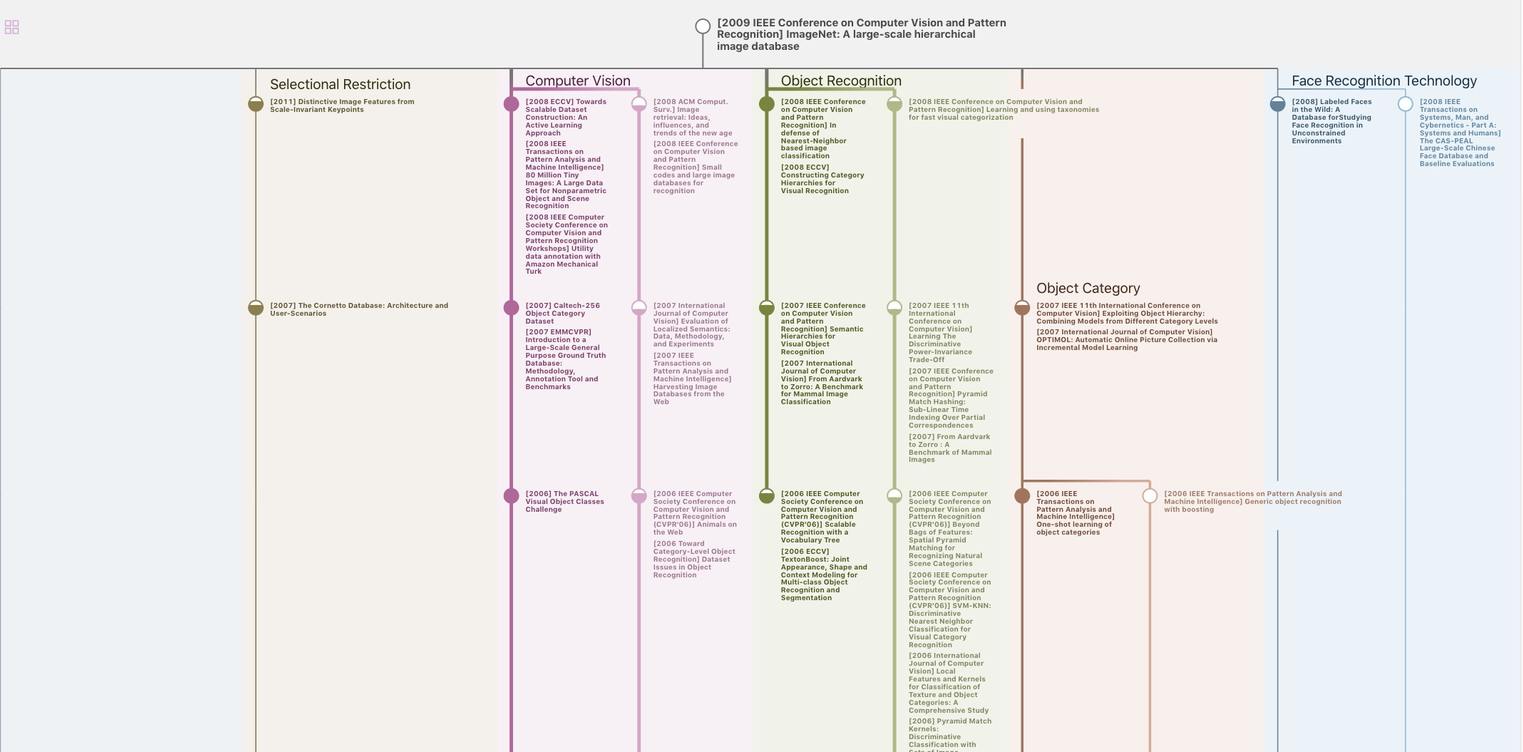
生成溯源树,研究论文发展脉络
Chat Paper
正在生成论文摘要