State Representation Learning for Task and Motion Planning in Robot Manipulation
2023 IEEE INTERNATIONAL CONFERENCE ON DEVELOPMENT AND LEARNING, ICDL(2023)
摘要
Employing the knowledge representation model designed by human experts has long been the dominant methodology in task and motion planning (TAMP). However, this type of method is time-consuming and suffers from the domain-dependence problem. In this paper, we focus on TAMP of robot arm manipulation based on state representation learning. We present a state representation learning method and a joint learning strategy for both the state representation model and the environment model, enabling the robot to learn the environment model autonomously, thereby mitigating the issue of domain-dependence. To improve planning efficiency and task success rate, we also incorporate a search pruning strategy based on value function learning and a re-planning method based on Model Predictive Control (MPC). The proposed method is evaluated in the simulation and real-robot experiments and shown to be effective compared to current TAMP systems.
更多查看译文
关键词
state representation learning,environment model,task and motion planning,robot manipulation
AI 理解论文
溯源树
样例
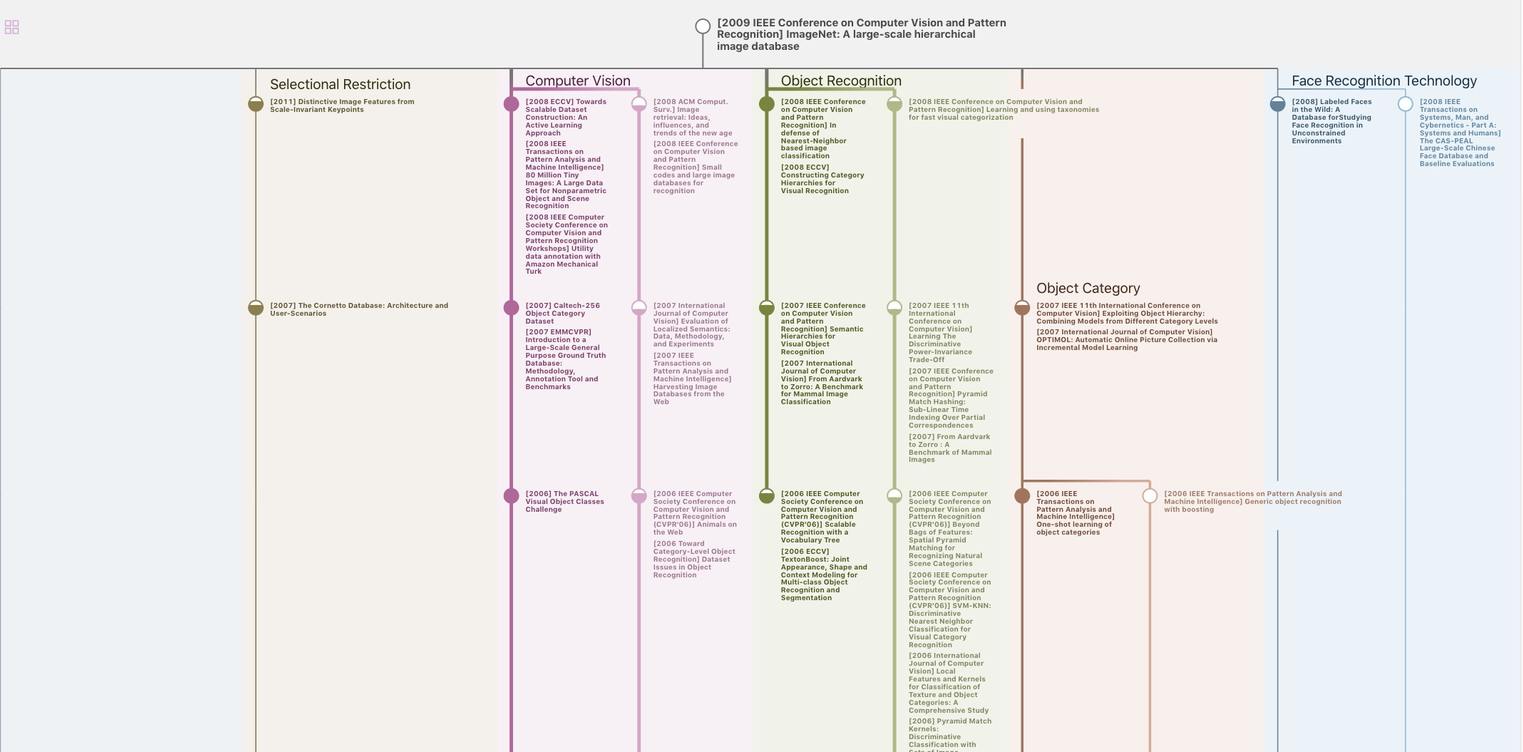
生成溯源树,研究论文发展脉络
Chat Paper
正在生成论文摘要