HACNet: End-to-end learning of interpretable table-to-image converter and convolutional neural network
KNOWLEDGE-BASED SYSTEMS(2024)
摘要
Motivated by the high prediction performance of convolutional neural networks (CNNs), several works have applied them to tabular datasets. As CNNs are built to accept images, several transformations of tabular data have been proposed to obtain images. However, existing methods transform the tabular data into images prior to CNN training, which fails to take the prediction error into account. Additionally, they employ all features from the tables, including unimportant ones, to produce the images. Moreover, the created images might not become human-interpretable because they do not consider the interpretability of images as a metric. To overcome these problems, we propose a hard attention-based converter combined with a convolutional neural network (HACNet), consisting of an attention-based table-to-image converter and a CNN-based predictor. HACNet trains its components simultaneously by minimizing CNN prediction loss and mean squared error (MSE) between created and template images. Minimizing this MSE loss allows us to visually distinguish the created images with different labels. The attention-based converter selects exactly one feature for each pixel in the image via its hard attention mechanism with Gumbel-Softmax, enabling feature selection. We experimentally show that HACNet produces human-interpretable images, reduces used features, and achieves prediction performances comparative with existing methods on several benchmark datasets.
更多查看译文
关键词
Convolutional neural network,Tabular data,End-to-end learning,Interpretability,Feature selection
AI 理解论文
溯源树
样例
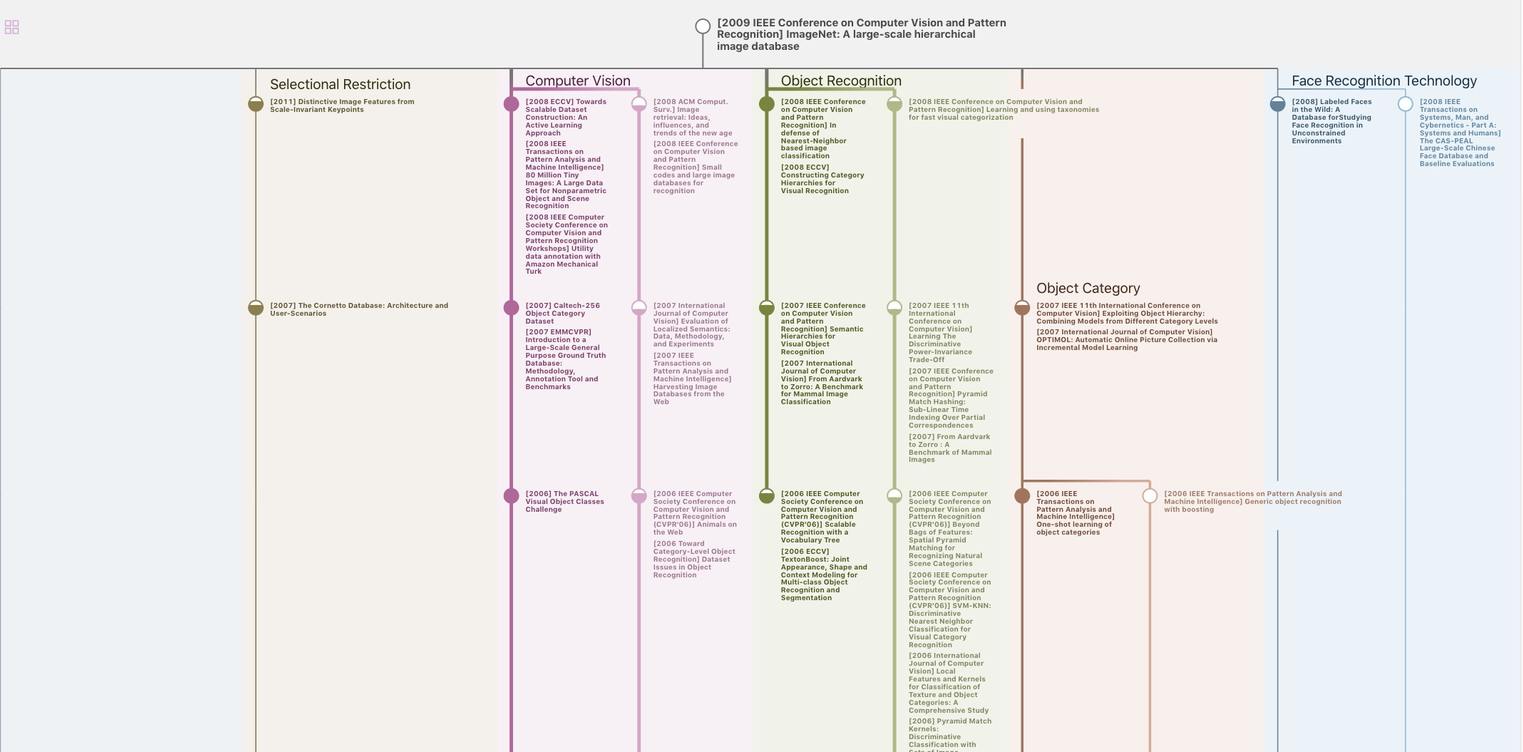
生成溯源树,研究论文发展脉络
Chat Paper
正在生成论文摘要