A Data-Driven Phantom Zeros Prediction Algorithm for Traction Force Sensor in Kinesthetic Demonstration.
2023 IEEE International Conference on Robotics and Biomimetics (ROBIO)(2023)
摘要
Kinesthetic demonstration requires accurate force/torque measurements from sensors. However, even in static conditions, the sensor readings exhibit non-zero fluctuations, which can lead to unstable force control and impair the quality of kinesthetic demonstration. In this paper, we refer to the above problem as phantom zeros and systematically analyze factors contributing to their variability of a traction force sensor. Neural networks (NN) are first introduced to model the complex nonlinear mapping between sensor orientations and phantom zeros. The initial parameters of the NN are then optimized using a genetic algorithm (GA) to prevent convergence to local optima and improve modeling accuracy. In addition, we develop an experimental platform with a physical UR10 robot and a custom traction sensor to comprehensively evaluate the proposed approach. Results demonstrate that the GA-optimized NN achieves higher precision and robustness in predicting phantom zeros under different orientations compared to least squares and vanilla NN baselines. By modeling and predicting phantom zeros, the proposed method can filter out phantom force fluctuations during kinesthetic demonstration, while preserving critical motion information. This work provides insights into modeling and mitigating force/torque sensor uncertainties for enabling more precise robot control and interactive guidance.
更多查看译文
AI 理解论文
溯源树
样例
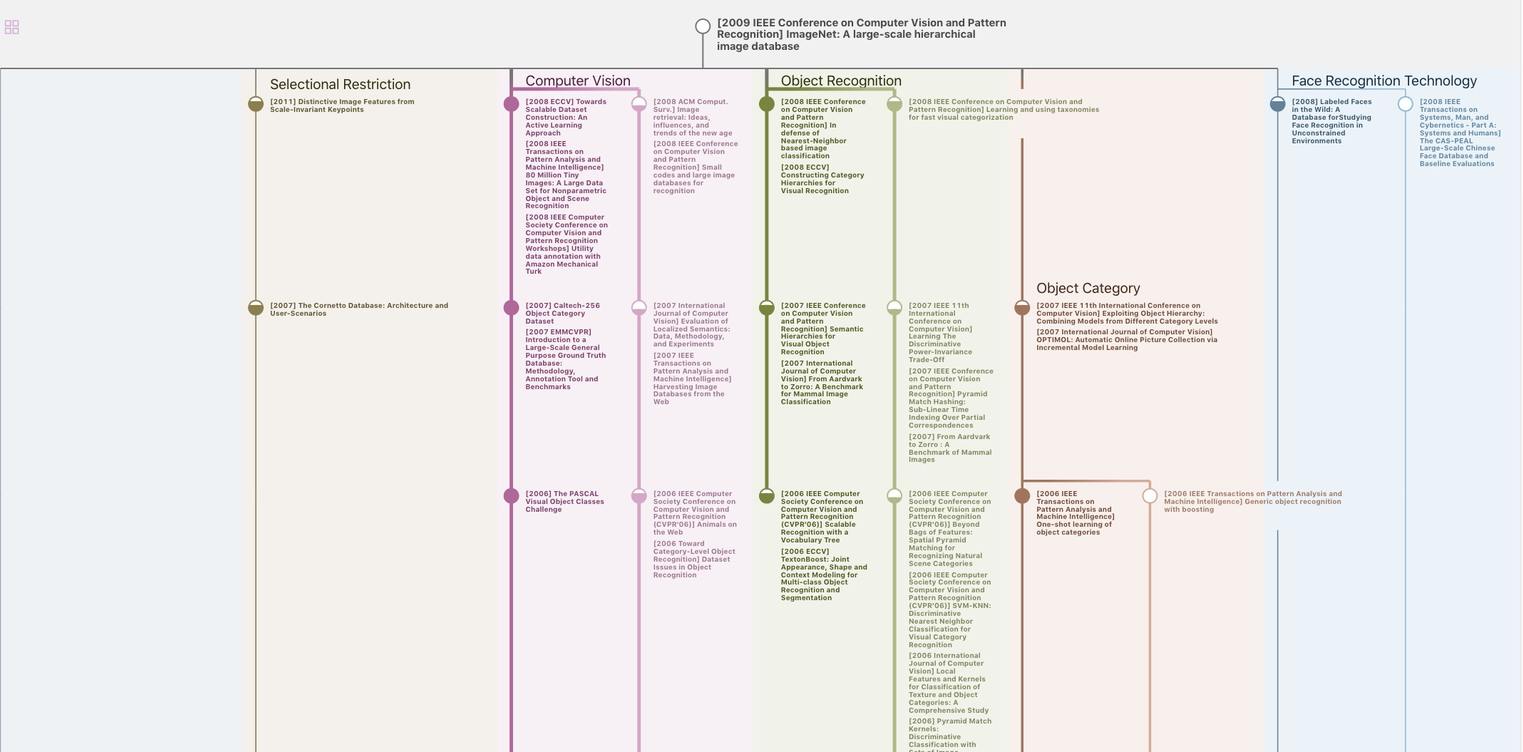
生成溯源树,研究论文发展脉络
Chat Paper
正在生成论文摘要