Analysis of the relationship between metro ridership and built environment: A machine learning method considering combinational features
TUNNELLING AND UNDERGROUND SPACE TECHNOLOGY(2024)
摘要
Limited studies have examined the relationship between combinational features of the built environment and metro ridership. In this study, we applied the gradient boosting regression tree (GBRT) to explore the non-linearity effects for metro commuter ridership and non-commuter ridership, respectively. The results show that the number of bus stops is an important predictor of urban metro commuter ridership, whereas residential and community services, road density, and leisure and entertainment are important predictors of urban metro non-commuter ridership. Based on the partial dependence plots generated by GBRT, the results confirm that combinational features of the built environment have a larger impact on commuter ridership (e.g., high-high level road density-life services on the destination side). Combinational features at a higher level exhibit a more substantial influence on commuter ridership, whereas combinational features at a lower level demonstrate a greater impact on non-commuter ridership.
更多查看译文
关键词
Metro,Built environment,Combinational features,Nonlinear interaction,Gradient boosting regression tree
AI 理解论文
溯源树
样例
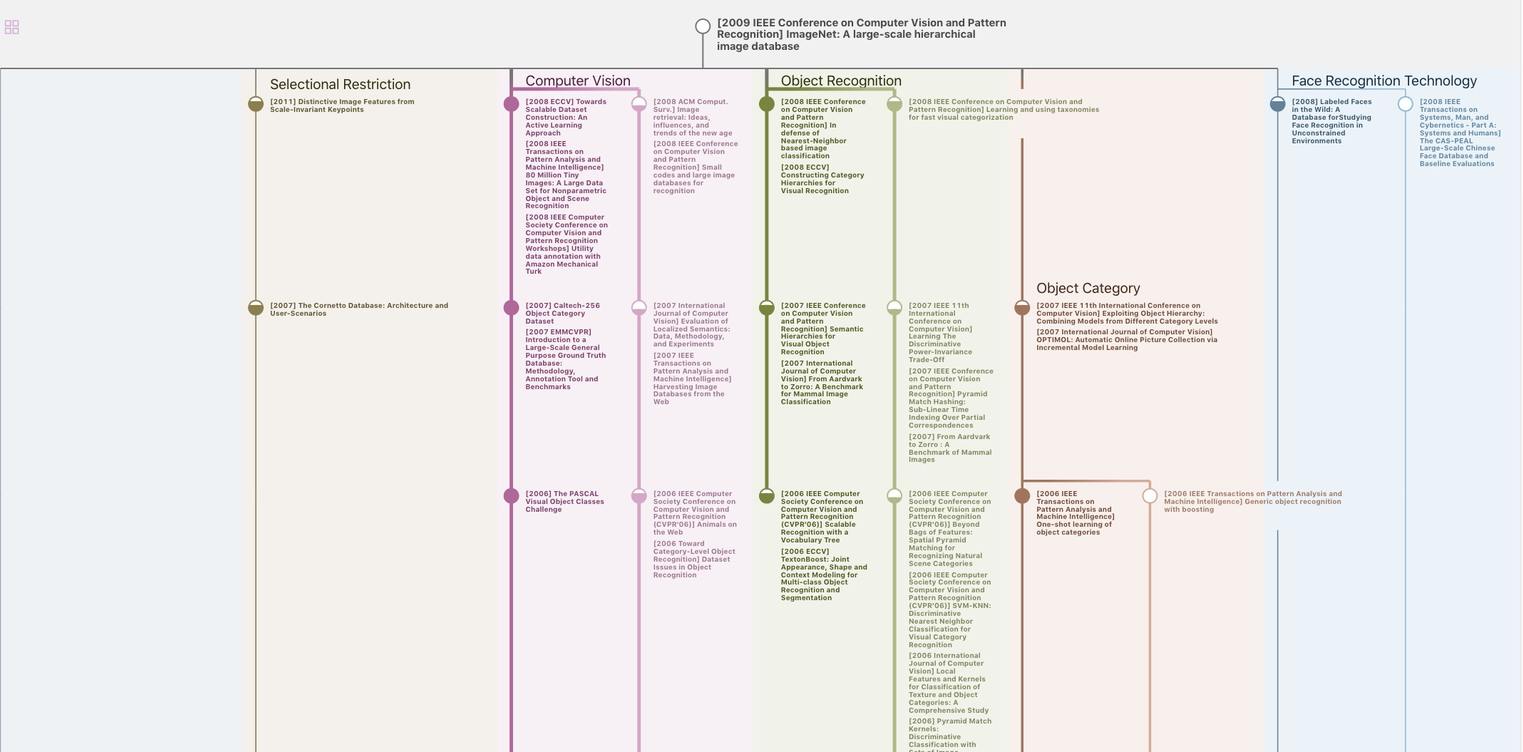
生成溯源树,研究论文发展脉络
Chat Paper
正在生成论文摘要