Unsupervised Monocular Depth Estimation for Monocular Visual SLAM Systems
IEEE TRANSACTIONS ON INSTRUMENTATION AND MEASUREMENT(2024)
摘要
Estimating monocular depth and ego-motion via unsupervised learning has emerged as a promising approach in autonomous driving, mobile robots, and augmented reality (AR)/VR applications. It avoids intensive efforts to collect a large amount of ground truth and further improves the scene construction density and long-term tracking accuracy in simultaneous localization and mapping (SLAM) systems. However, existing approaches are susceptible to illumination variations and blurry pictures due to fast movements in real-world driving scenarios. In this article, we propose a novel unsupervised learning framework to fuse the complementary strength of visual and inertial measurements for monocular depth estimation. It learns both forward and backward inertial sequences at multiple subspaces to produce environment-independent and scale-consistent motion features and selectively weights inertial and visual modalities to adapt to various scenes and motion states. In addition, we explore a novel virtual stereo model to adopt such depth estimates in the monocular SLAM system, thus improving the system efficiency and accuracy. Extensive experiments on the KITTI, EuRoC, and TUM datasets have shown our effectiveness in terms of monocular depth estimation, SLAM initialization efficiency, and pose estimation accuracy compared with the state-of-the-art.
更多查看译文
关键词
Estimation,Visualization,Simultaneous localization and mapping,Cameras,Lighting,Feature extraction,Pose estimation,Feature extraction,monocular depth estimation,monocular visual simultaneous localization and mapping (SLAM),unsupervised learning,visual-inertial fusion
AI 理解论文
溯源树
样例
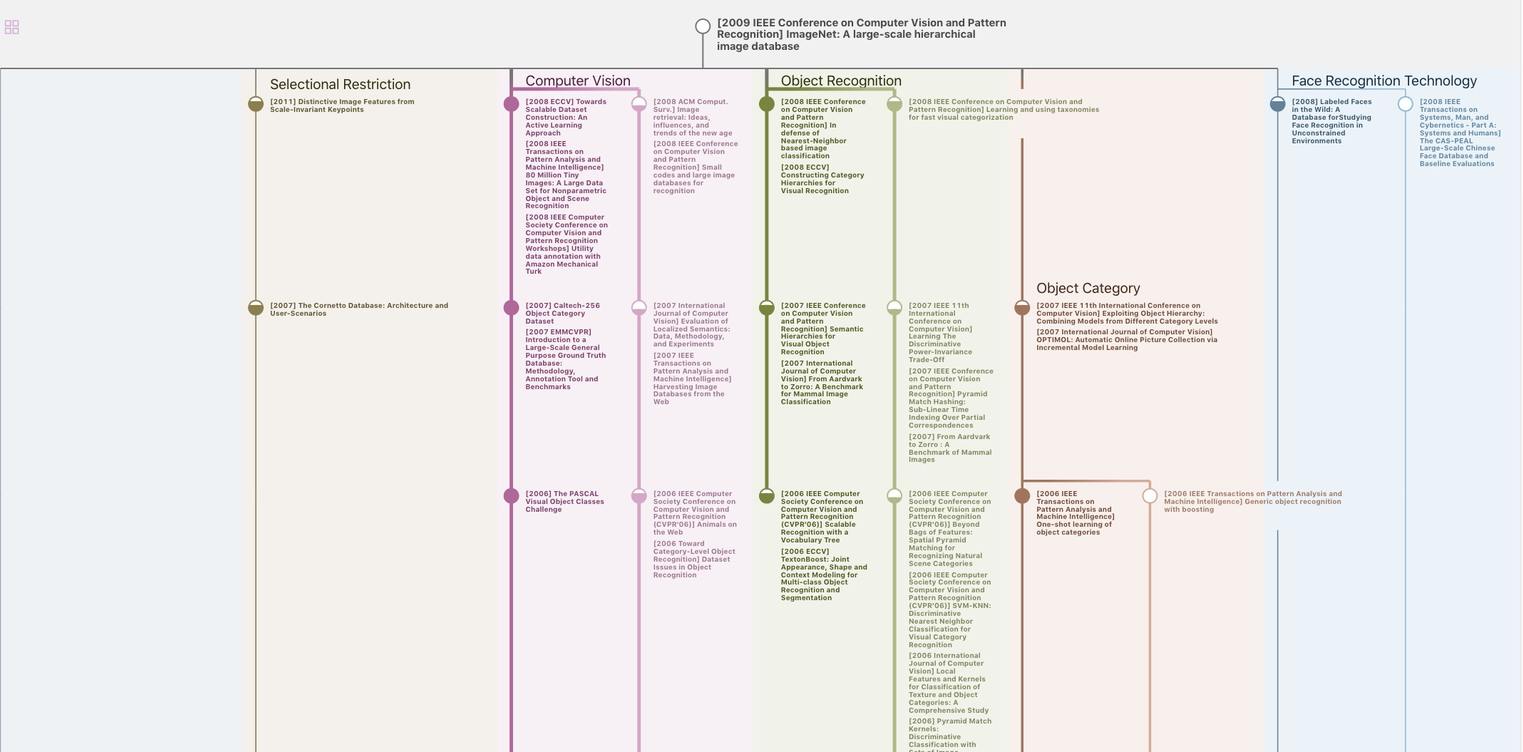
生成溯源树,研究论文发展脉络
Chat Paper
正在生成论文摘要