Aspect-level sentiment analysis based on aspect-sentence graph convolution network
INFORMATION FUSION(2024)
摘要
Aspect-level sentiment analysis aims to identify the sentiment polarity of aspect words in sentences. The existing research methods only focus on the grammatical dependencies between words, ignoring the semantic connections between aspect words and the dependency types between words, which limits the performance of the aspect-level sentiment analysis model. Therefore, this paper proposes an aspect-sentence Graph Convolutional Networks model (ASGCN) to perceive more comprehensive semantic information. Specifically, the model consists of sentence-focused GCN (SentenceGCN) and aspects-focused GCN (AspectsGCN) sub models. In the SentenceGCN model, this paper proposes a method to calculate the adjacency matrix (As) of syntactic dependency graph, which uses the position encoding mechanism and pays attention to the influence of different dependency types on semantics, so that SentenceGCN can capture the semantic information of the whole sentence more comprehensively. In the AspectsGCN model, this paper also proposes a method to calculate the adjacency matrix (Aa) of aspect words, which models the relational graph as fully connected and gives weight to the edges between aspect words according to the position, so that the AspectsGCN can pay attention to the semantic relation between different aspect words in the sentence. The proposed model outperforms all baseline models with 86.34 % accuracy and 79.96 F1 score, which indicates that there are more advantages in perceiving semantic information in ASGCN.
更多查看译文
关键词
Aspect-level sentiment analysis,Graph convolutional neural network,Aspect words,Syntactic dependency tree,Position coding
AI 理解论文
溯源树
样例
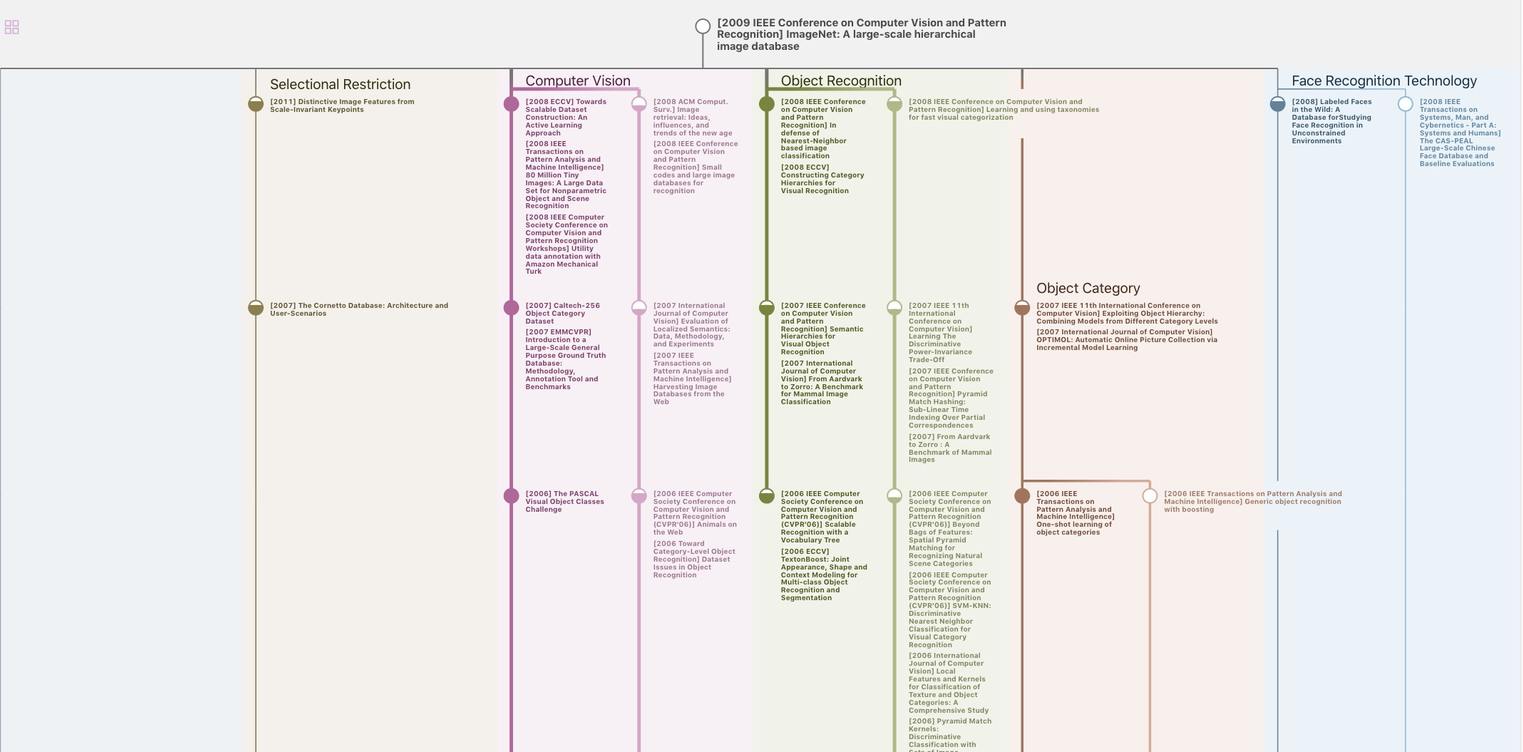
生成溯源树,研究论文发展脉络
Chat Paper
正在生成论文摘要