Age- and Severity-Specific Deep Learning Models for Autism Spectrum Disorder Classification Using Functional Connectivity Measures
Arabian Journal for Science and Engineering(2023)
摘要
Autism spectrum disorder (ASD) is characterized by divergent etiological factors, comorbidities, severity levels, genetic influences, and functional connectivity (FC) patterns in the brain. In the literature, ASD classification based on age and severity using fMRI data is extremely limited. This study explores the impact of age, symptom severity, and brain FC patterns on the diagnosis of ASD using deep neural networks (DNNs). The ability to classify ASD by age and severity using fMRI data is extremely limited. This study explores the impact of age, symptom severity, and brain FC patterns on the diagnosis of ASD using deep neural networks (DNNs). The FC measures were extracted using Pearson's correlation coefficient (PCC), fractal connectivity (FrC), and nonfractal connectivity (NFrC) from the ABIDE I and II databases. We studied three age groups (6 to 11, 11 to 18, and 6 to 18 years) and two severity groups (ADOS score ≤ 11 and ADOS score > 11). The FC matrices are constructed from blood oxygen level-dependent (BOLD) time series signals, and the heat maps are used to generate features for the convolutional neural network (CNN), MobileNetV2, and DenseNet201 models. The MobileNetV2 classifier achieved 76.25
更多查看译文
关键词
Autism spectrum disorder,Age and severity,Fractal and non-fractal connectivity,Deep learning
AI 理解论文
溯源树
样例
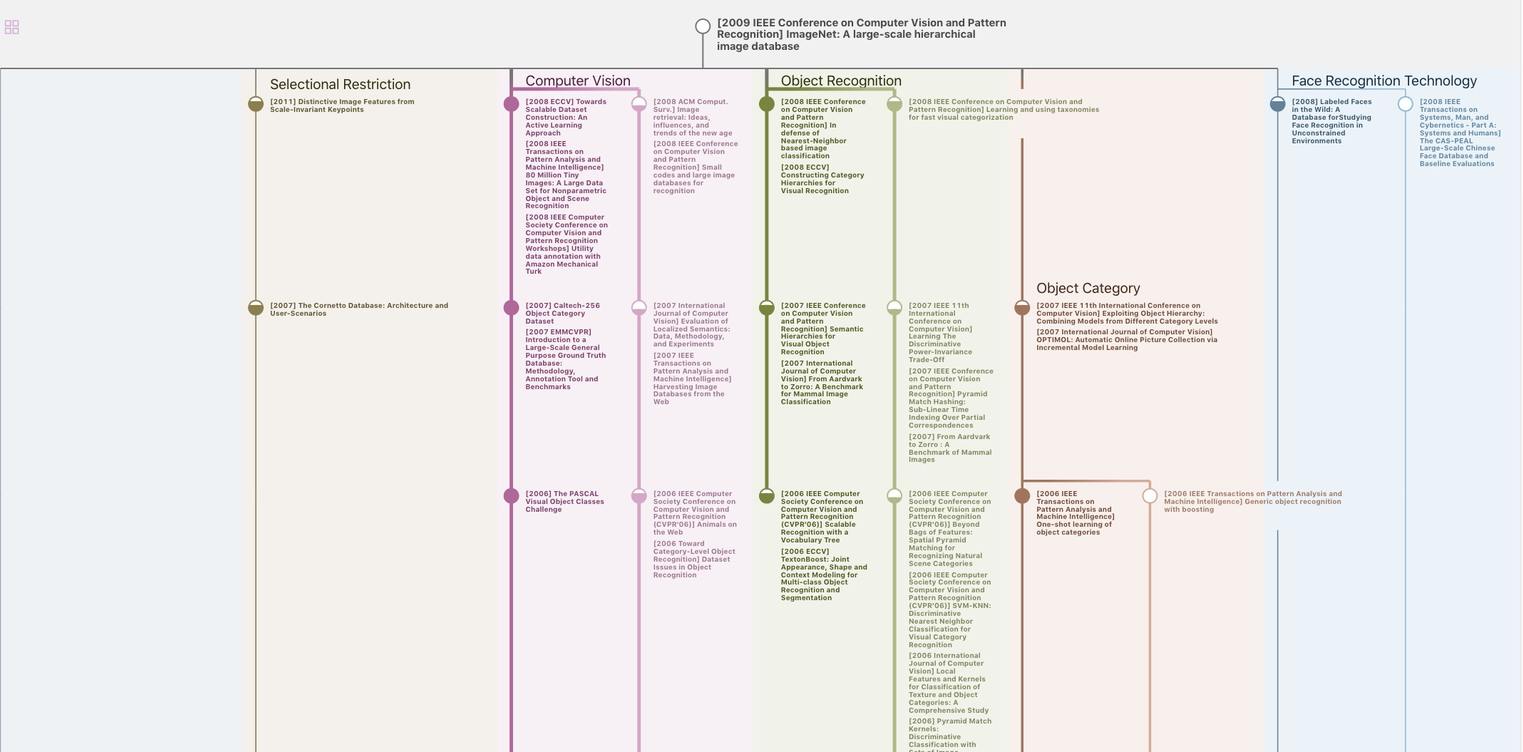
生成溯源树,研究论文发展脉络
Chat Paper
正在生成论文摘要