DSSE-net: dual stream skip edge-enhanced network with forgery loss for image forgery localization
International Journal of Machine Learning and Cybernetics(2023)
摘要
As deep learning has continuously made breakthroughs in computer vision, Image Forgery Localization (IFL) task has also started using deep learning frameworks. Currently, most deep learning-based IFL methods use binary cross entropy as the loss function during model training. However, the number of tampered pixels in image forgery is significantly smaller than the number of real pixels. This disparity makes it easier for the model to classify samples as real pixels during training, leading to a reduced F1 score. Therefore, in this paper, we have proposed a loss function for the IFL task: Forgery Loss. The Forgery Loss assigns weight to the classification loss of tampered pixels and edges, enhances tampered pixel constraints in the model, and amplifies the importance of difficult-to-classify samples. These enhancements facilitate the model to acquire more productive information. Consequently, the F1 score of the model is enhanced. Additionally, we designed an end-to-end, pixel-level detection network DSSE-Net. It comprises of a dual-stream codec network that extracts high-level and low-level features of images, and an edge attention stream. The edge attention stream have a Edge Attention Model which enhances the network’s attention to the high frequency edges of the image and, in conjunction with the edge enhancement algorithm in Forgery Loss, improves the model’s ability to detect tampered edges. Experiments demonstrate that Forgery Loss can effectively improve the F1 score, while the DSSE-Net accuracy outperforms the current SOTA algorithm.
更多查看译文
关键词
Image forgery localization,Dual stream network,Forgery loss,Attention mechanisms
AI 理解论文
溯源树
样例
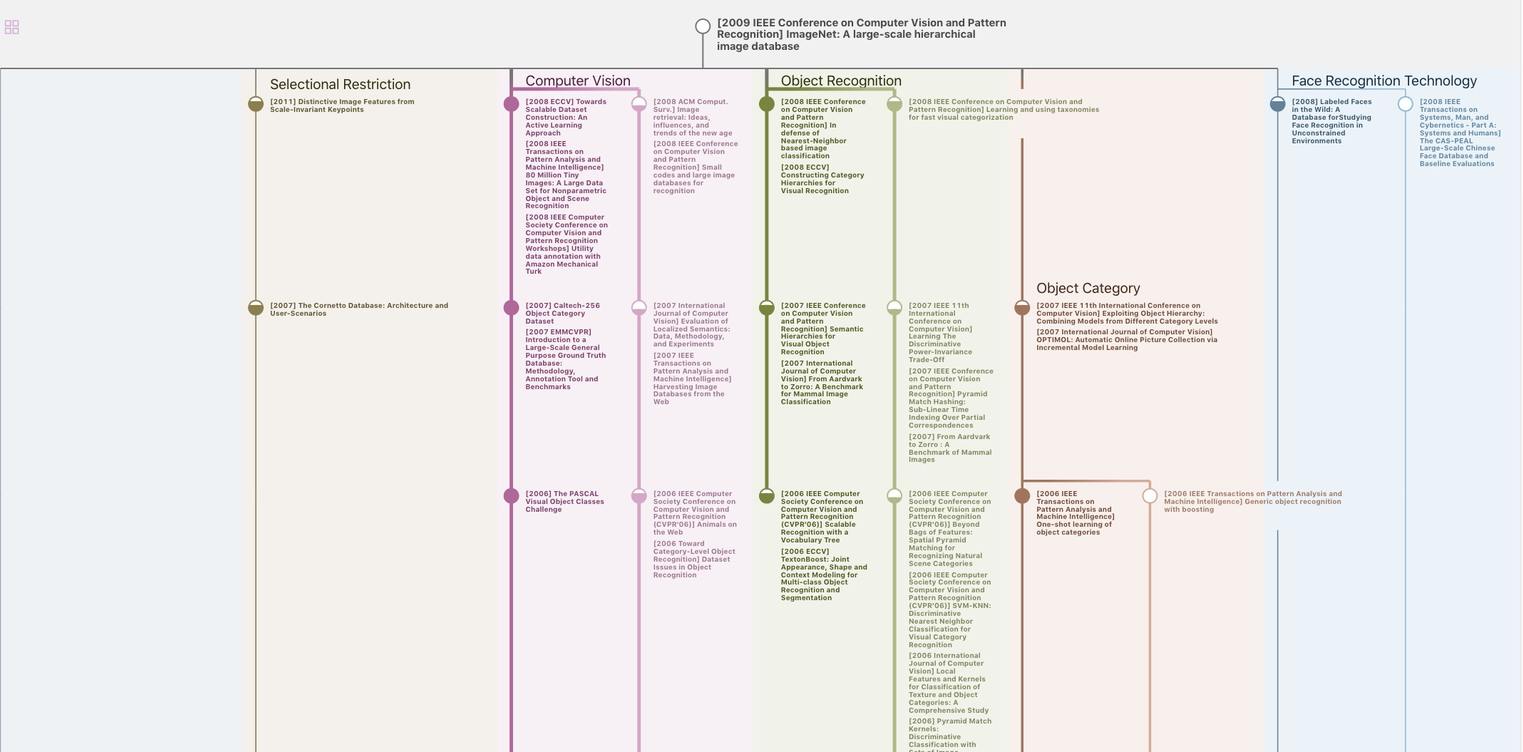
生成溯源树,研究论文发展脉络
Chat Paper
正在生成论文摘要