Integrating reinforcement-learning-based vehicle dispatch algorithm into agent-based modeling of autonomous taxis
Transportation(2023)
摘要
While increasing number of studies are using agent-based models to study the potential environmental, economic, and social impacts of shared autonomous vehicles, the idle vehicle dispatching in these models is often simplified to heuristic rules. Because the system performance of an autonomous taxi fleet can be significantly affected by the vehicle dispatching algorithm, refining the vehicle dispatching can help us better evaluate the potential benefits and impacts of shared autonomous vehicle systems. The recent development of reinforcement-learning-based vehicle dispatching algorithms provides opportunities to improve autonomous vehicle system modeling with efficient and scalable vehicle dispatching. This study integrates a reinforcement learning algorithm into to an agent-based simulation model of a ride hailing system. Using an autonomous taxi fleet in New York City as a case study, we compared the system performance of using the proposed Deep Q-Network (DQN) method for dispatching decision with common rule-based and heuristic dispatch algorithms from relevant literatures. The results show that (1) DQN dispatches vehicles more conservatively (with less dispatching activities and distances) but achieved similar (slightly lower) rider service level with proactive dispatch methods; and (2) DQN outperformed all other dispatch methods evaluated in this study with significantly higher dispatch efficiency, as measured by the ratio of the number of extra riders served due to dispatching to the extra fleet dispatch distance.
更多查看译文
关键词
Autonomous vehicles,Agent-based modeling,Reinforcement learning,Ride hailing,Vehicle dispatching efficiency
AI 理解论文
溯源树
样例
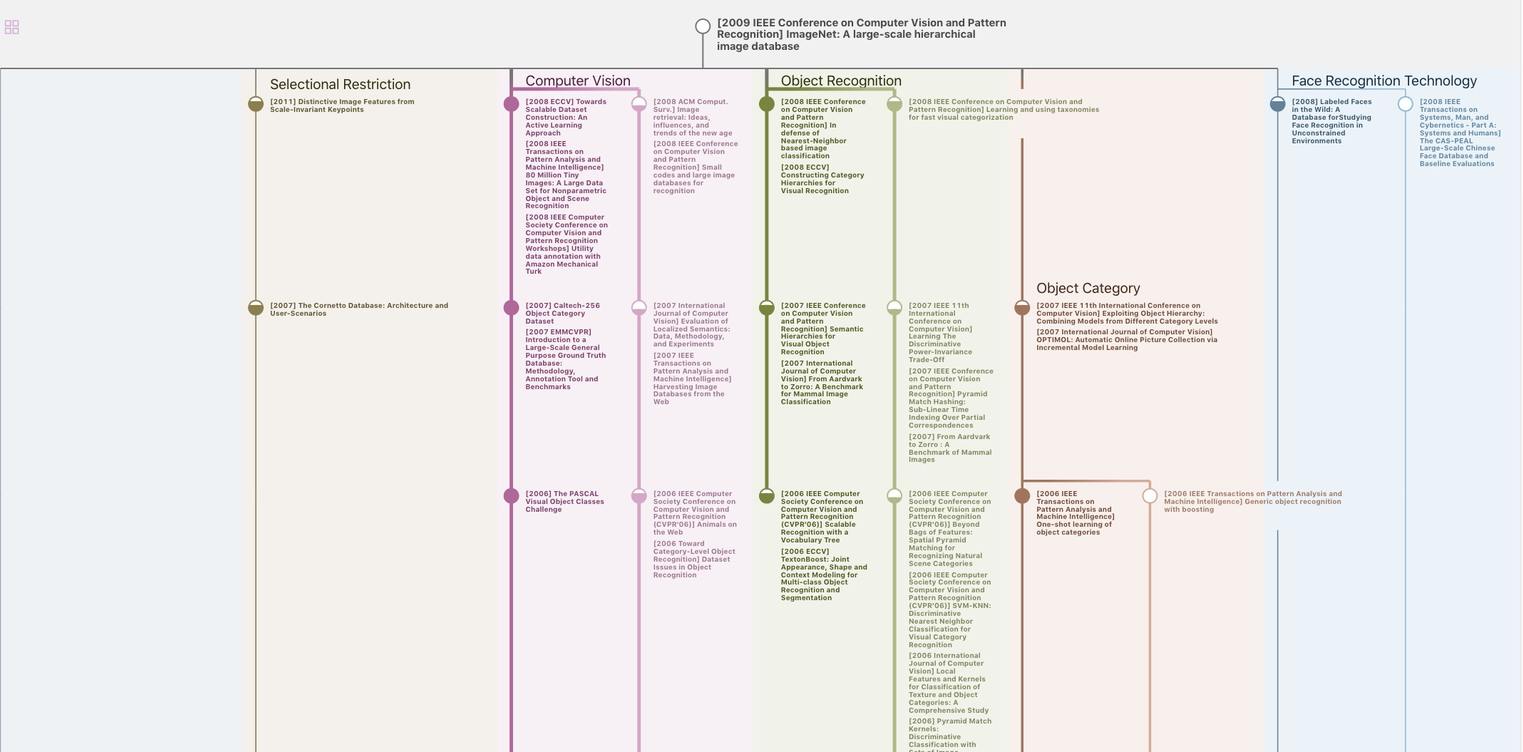
生成溯源树,研究论文发展脉络
Chat Paper
正在生成论文摘要