A signal-to-image fault classification method based on multi-sensor data for robotic grinding monitoring
Journal of Intelligent Manufacturing(2023)
摘要
This paper establishes a framework to analyze the state of grinding equipment by vibration signals of multi-sensors, which aims to solve the difficult problem of online quality monitoring in the grinding process. Firstly, the MSDP (modified symmetrized dot pattern) method is developed to transform time series into visualized images, which integrates multi-sensor data while involving more representative information for classification. Then, optimal parameters to generate MSDP images are selected based on the developed index to clearly distinguish different types of images while resembling each other of the same type, which improves the quality of training images for more effective feature extraction. Aiming at improving the ability of feature extraction, a multi-scale convolutional neural network (CNN) model is designed to classify the MSDP images representing different grinding processes, which utilizes different scales of kernels to acquire multi-scale fault information. Experiments are carried out on a real robotic grinding system to verify the performance of the fault classification framework for robotic grinding monitoring. The results show that the images generated by the selected optimal parameters along with the MSDP method are more conducive to classification, and the proposed multi-scale CNN achieves improved classification performance by involving multi-scale features.
更多查看译文
关键词
Fault classification,Symmetrized dot pattern (SDP),Multi-sensor data,Convolutional neural network (CNN),Robotic grinding monitoring
AI 理解论文
溯源树
样例
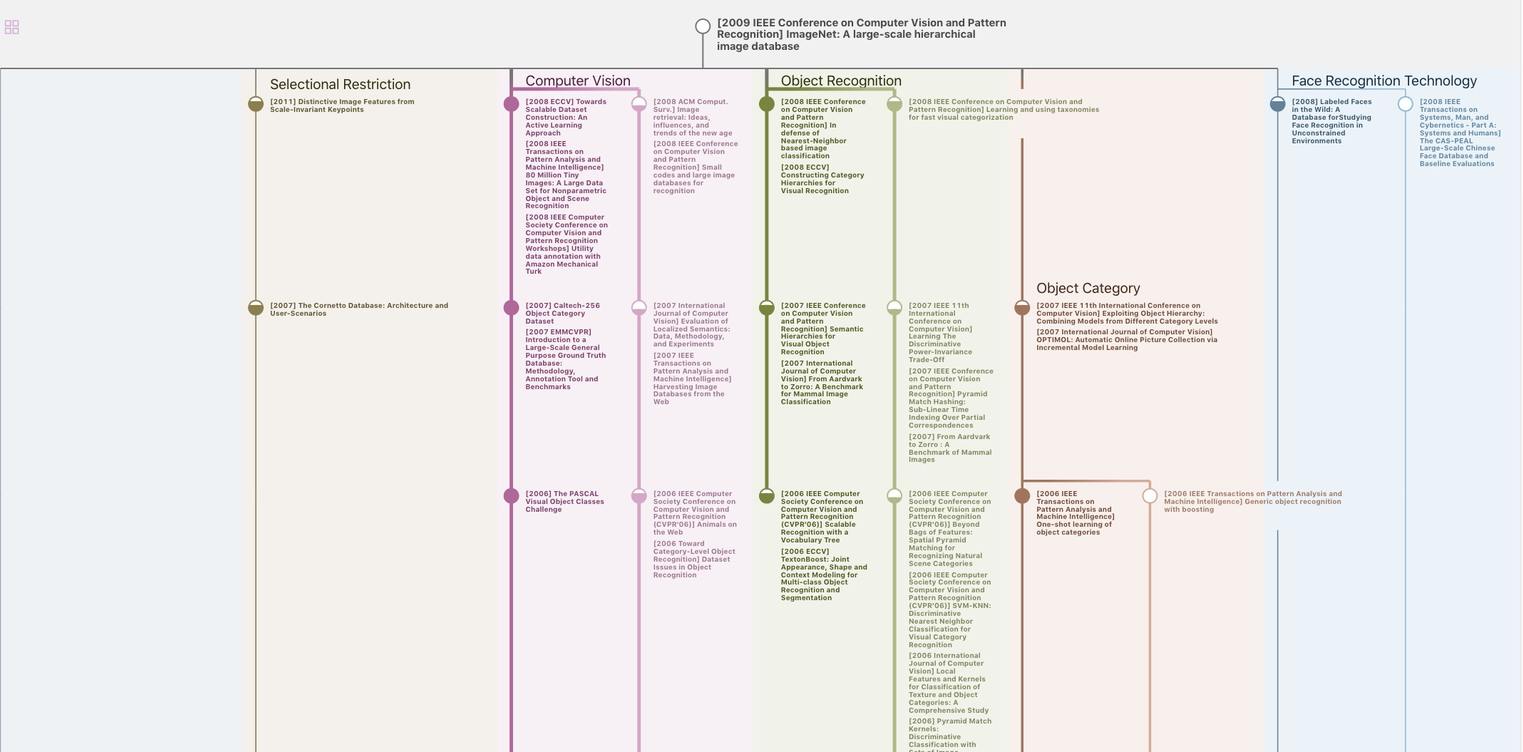
生成溯源树,研究论文发展脉络
Chat Paper
正在生成论文摘要