Sensor fault diagnosis and correction for data center cooling system using hybrid multi-label random Forest and Bayesian Inference
BUILDING AND ENVIRONMENT(2024)
摘要
The measurement biases in working sensors significantly hampers the effective operation and control of cooling systems in the data center. However, previous studies only focus on sensor fault diagnosis or sensor bias correction, neglecting the simultaneous detection, diagnosis and correction of sensor faults. This study proposed a novel method, combining Multi-Label Random Forest and Bayesian Inference (HMLRF-BI), to simultaneously detect, diagnose and correct sensor faults. Case studies were conducted involving eight single sensors and six multiple sensor fault cases in the Computer Room Air Handler (CRAH) to comprehensively evaluate the diagnostic and correction performance of the proposed method. Simulation results demonstrate that the method accurately diagnoses sensor fault types with a diagnostic accuracy of 97.42 % and an F1 score exceeding 97.90 %. Moreover, the proposed method also performs well in single/multiple sensor bias correction scenarios, with a correction accuracy exceeding 96.81 % and 97.10 %, respectively. Furthermore, a novel cycle mechanism is proposed, which utilized the MLRF fault diagnosis model to re-diagnose the corrected fault sensor to complete the closed-loop cycle of the HMLRF-BI method with an overall accuracy of 96.17 %. This study successfully filled the knowledge gap in the simultaneous detection, diagnosis and correction of sensor faults in data center CRAH, providing a comprehensive solution to restore sensor measurement performance, which is a promising application method.
更多查看译文
关键词
Data center,Senor fault detection and diagnosis,Computer room air handler,Bias correction,Multi-label random forest,Bayesian inference
AI 理解论文
溯源树
样例
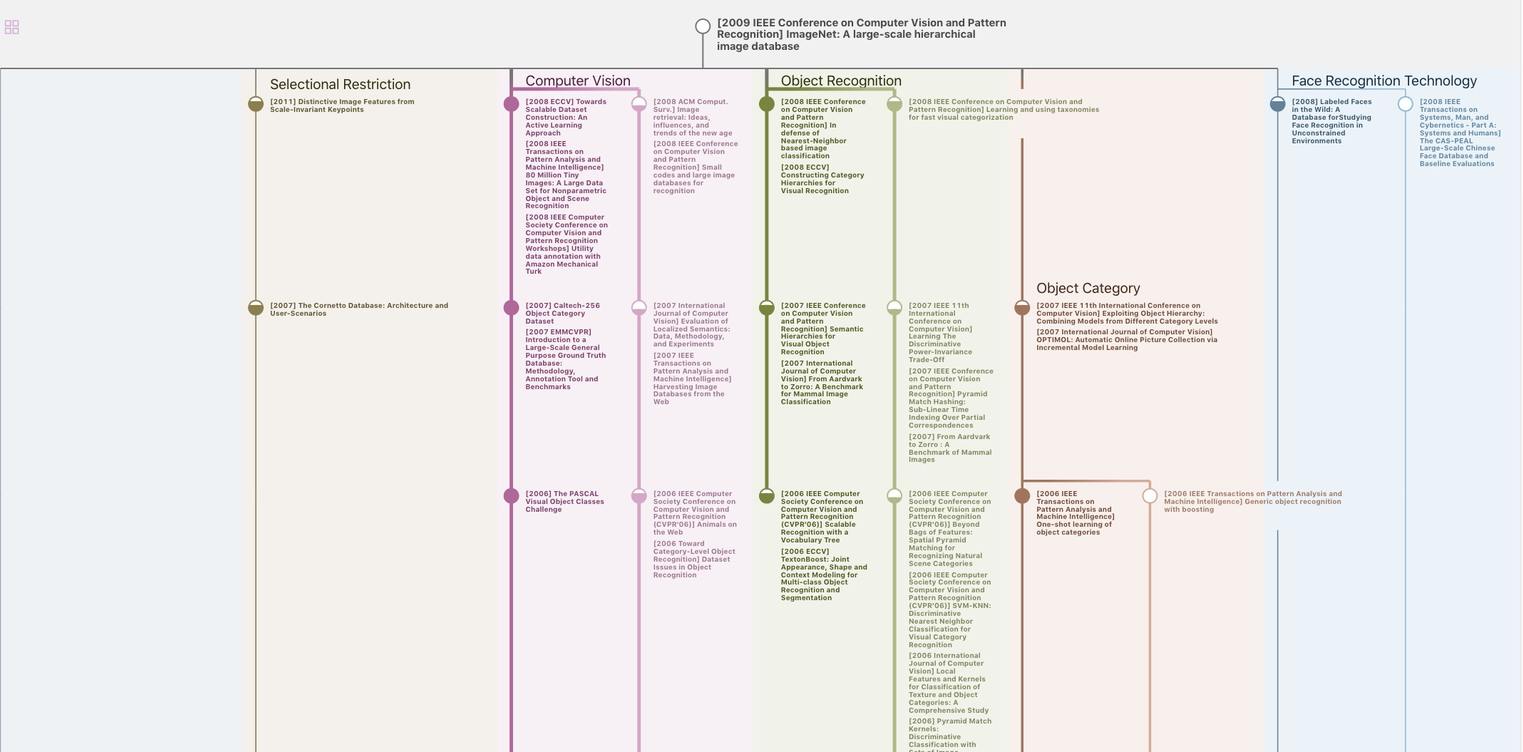
生成溯源树,研究论文发展脉络
Chat Paper
正在生成论文摘要