SecCT: Secure and scalable count query models on encrypted genomic data.
2023 IEEE 28th Pacific Rim International Symposium on Dependable Computing (PRDC)(2023)
摘要
Recently, due to the continued reduction in DNA sequencing cost, large-scale genetic samples are being gathered for accelerating predispositions to specific diseases, tailoring treatment of efficient drugs and therapies, etc. Massive genetic samples are encrypted-and-then-delegated to a public cloud to both save investment and maintenance costs and prevent the potential leakage of sensitive information. However, such a manner compromises the serviceability of a public cloud, since encryption inevitably breaks the semantic information of genetic samples. Secure count query of single-nucleotide polymorphisms (SNPs), as a kernel component for GWASs and related genomic analysis, is attracting much more attention.Existing methods lack provable security, suffer low efficiency caused by multiple interactions with the cloud, etc. In this paper, a secure virtual CT-Tree (secure vCT-Tree) is carefully constructed to confuse the tree structure by introducing a hash function and a Paillier system. Furthermore, by delegating the secure vCT-Tree to the cloud, concrete models (i.e., SecCT and SecCT+) are presented to resolve secure count query problems on-the-fly. Both models advance the provable security of genetic research and are proven to be secure under the adaptive chosen keyword (query) attack (IND-CKA2) model. Furthermore, massive experiments are evaluated on realistic data to show the superiority of SecCT.
更多查看译文
关键词
Secure count query,genotypic and phenotypic data,IND-CKA security,scalability
AI 理解论文
溯源树
样例
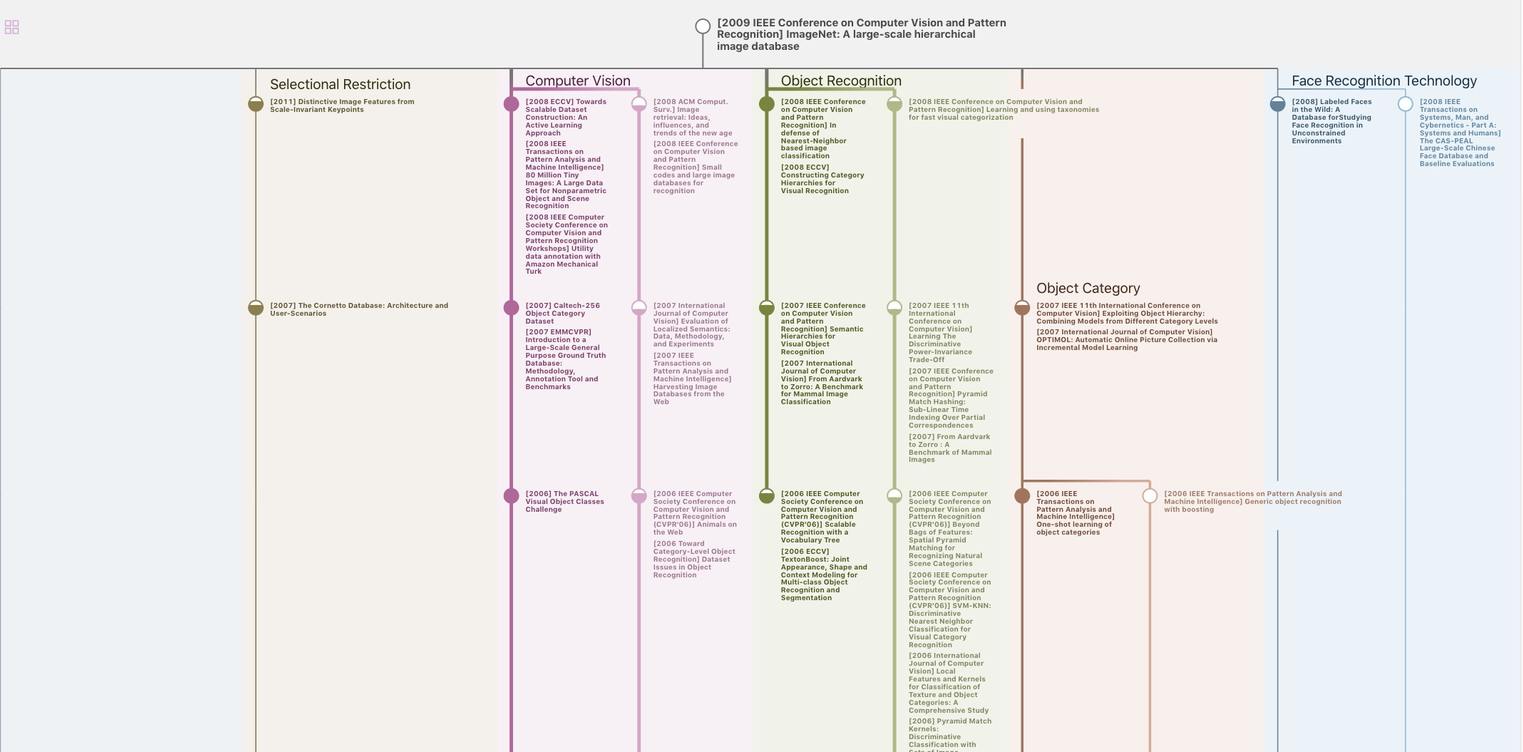
生成溯源树,研究论文发展脉络
Chat Paper
正在生成论文摘要